MLProbs: A Data-Centric Pipeline for Better Multiple Sequence Alignment
IEEE/ACM Transactions on Computational Biology and Bioinformatics(2023)
摘要
In this paper, we explore using the data-centric approach to tackle the Multiple Sequence Alignment (MSA) construction problem. Unlike the algorithm-centric approach, which reduces the construction problem to a combinatorial optimization problem based on an abstract mathematical model, the data-centric approach explores using classification models trained from existing benchmark data to guide the construction. We identified two simple classifications to help us choose a better alignment tool and determine whether and how much to carry out realignment. We show that shallow machine-learning algorithms suffice to train sensitive models for these classifications. Based on these models, we implemented a new multiple sequence alignment pipeline, called MLProbs. Compared with 10 other popular alignment tools over four benchmark databases (namely, BAliBASE, OXBench, OXBench-X and SABMark), MLProbs consistently gives the highest TC score. More importantly, MLProbs shows non-trivial improvement for protein families with low similarity; in particular, when evaluated against the 1,356 protein families with similarity
$\leq$
50%, MLProbs achieves a TC score of 56.93, while the next best three tools are in the range of [55.41, 55.91] (increased by more than 1.8%). We also compared the performance of MLProbs and other MSA tools in two real-life applications – Phylogenetic Tree Construction Analysis and Protein Secondary Structure Prediction – and MLProbs also had the best performance. In our study, we used only shallow machine-learning algorithms to train our models. It would be interesting to study whether deep-learning methods can help make further improvements, so we suggest some possible research directions in the conclusion section.
更多查看译文
关键词
Multiple sequence alignment,protein family,machine learning,classification
AI 理解论文
溯源树
样例
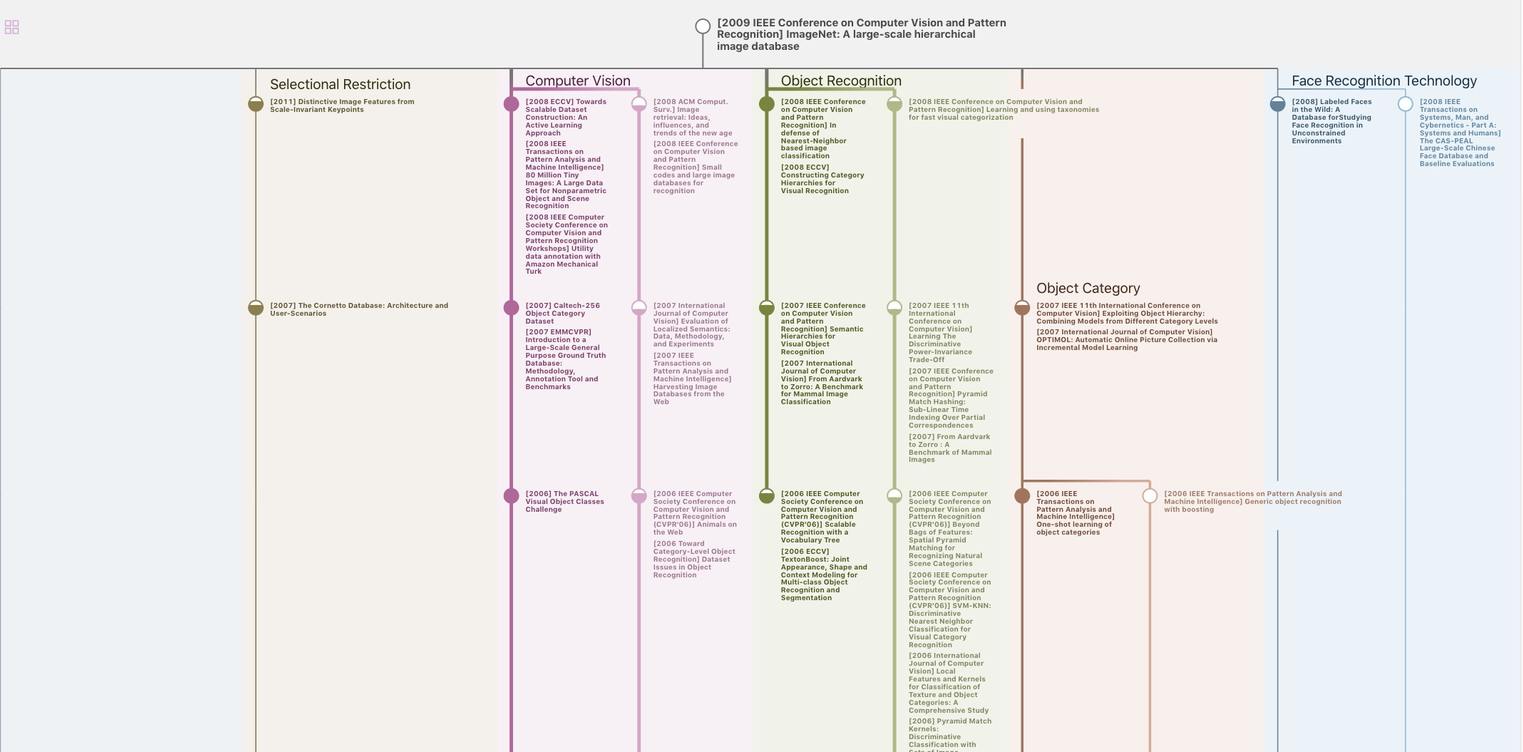
生成溯源树,研究论文发展脉络
Chat Paper
正在生成论文摘要