Causal Judgment in the Wild: Evidence from the 2020 US Presidential Election
COGNITIVE SCIENCE(2022)
摘要
When explaining why an event occurred, people intuitively highlight some causes while ignoring others. How do people decide which causes to select? Models of causal judgment have been evaluated in simple and controlled laboratory experiments, but they have yet to be tested in a complex real-world setting. Here, we provide such a test, in the context of the 2020 U.S. presidential election. Across tens of thousands of simulations of possible election outcomes, we computed, for each state, an adjusted measure of the correlation between a Biden victory in that state and a Biden election victory. These effect size measures accurately predicted the extent to which U.S. participants (N = 207, preregistered) viewed victory in a given state as having caused Biden to win the presidency. Our findings support the theory that people intuitively select as causes of an outcome the factors with the largest standardized causal effect on that outcome across possible counterfactual worlds.
更多查看译文
关键词
Causality, Causal judgment, Causal selection, Counterfactuals, Computational modeling
AI 理解论文
溯源树
样例
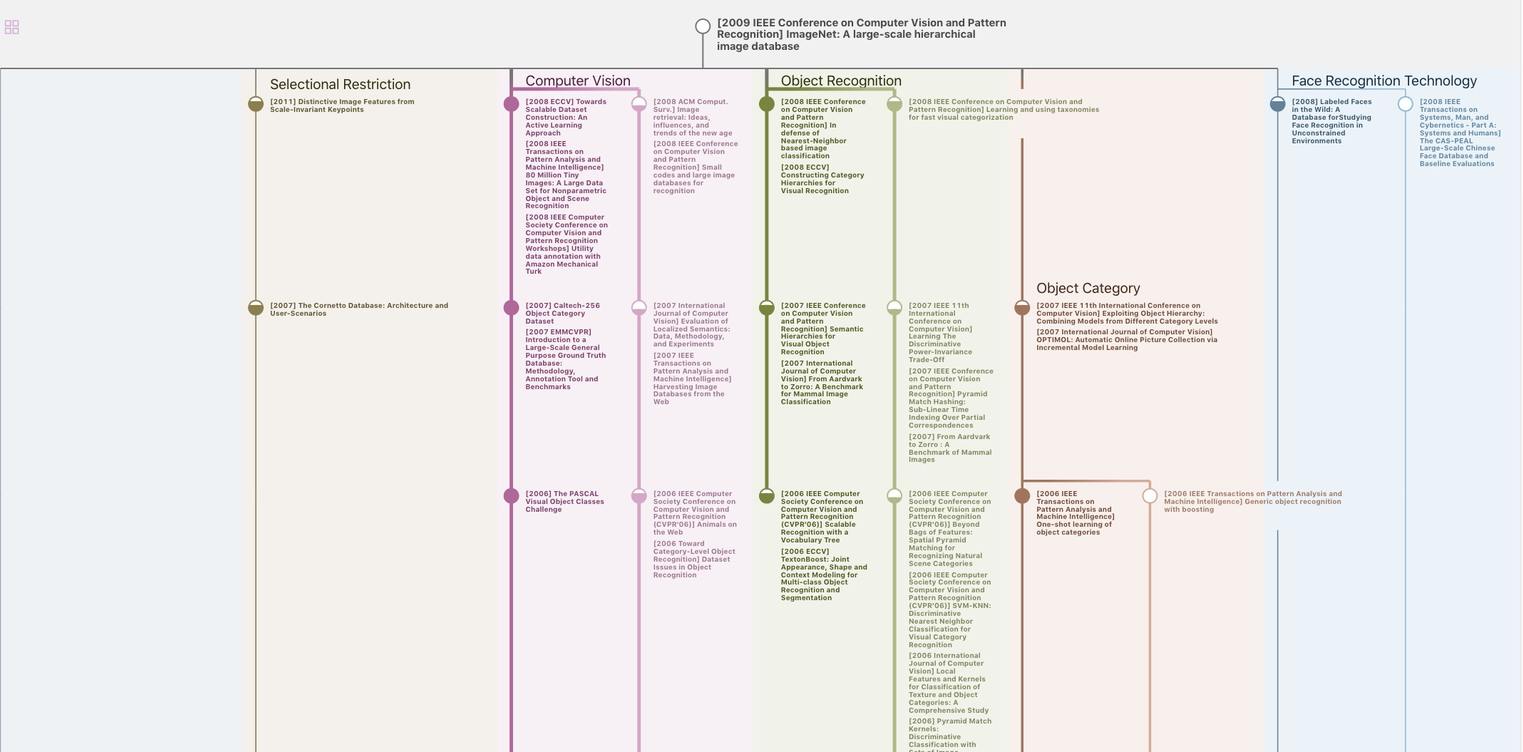
生成溯源树,研究论文发展脉络
Chat Paper
正在生成论文摘要