Robust Audio Anomaly Detection
arxiv(2022)
摘要
We propose an outlier robust multivariate time series model which can be used for detecting previously unseen anomalous sounds based on noisy training data. The presented approach doesn't assume the presence of labeled anomalies in the training dataset and uses a novel deep neural network architecture to learn the temporal dynamics of the multivariate time series at multiple resolutions while being robust to contaminations in the training dataset. The temporal dynamics are modeled using recurrent layers augmented with attention mechanism. These recurrent layers are built on top of convolutional layers allowing the network to extract features at multiple resolutions. The output of the network is an outlier robust probability density function modeling the conditional probability of future samples given the time series history. State-of-the-art approaches using other multiresolution architectures are contrasted with our proposed approach. We validate our solution using publicly available machine sound datasets. We demonstrate the effectiveness of our approach in anomaly detection by comparing against several state-of-the-art models.
更多查看译文
关键词
anomaly detection
AI 理解论文
溯源树
样例
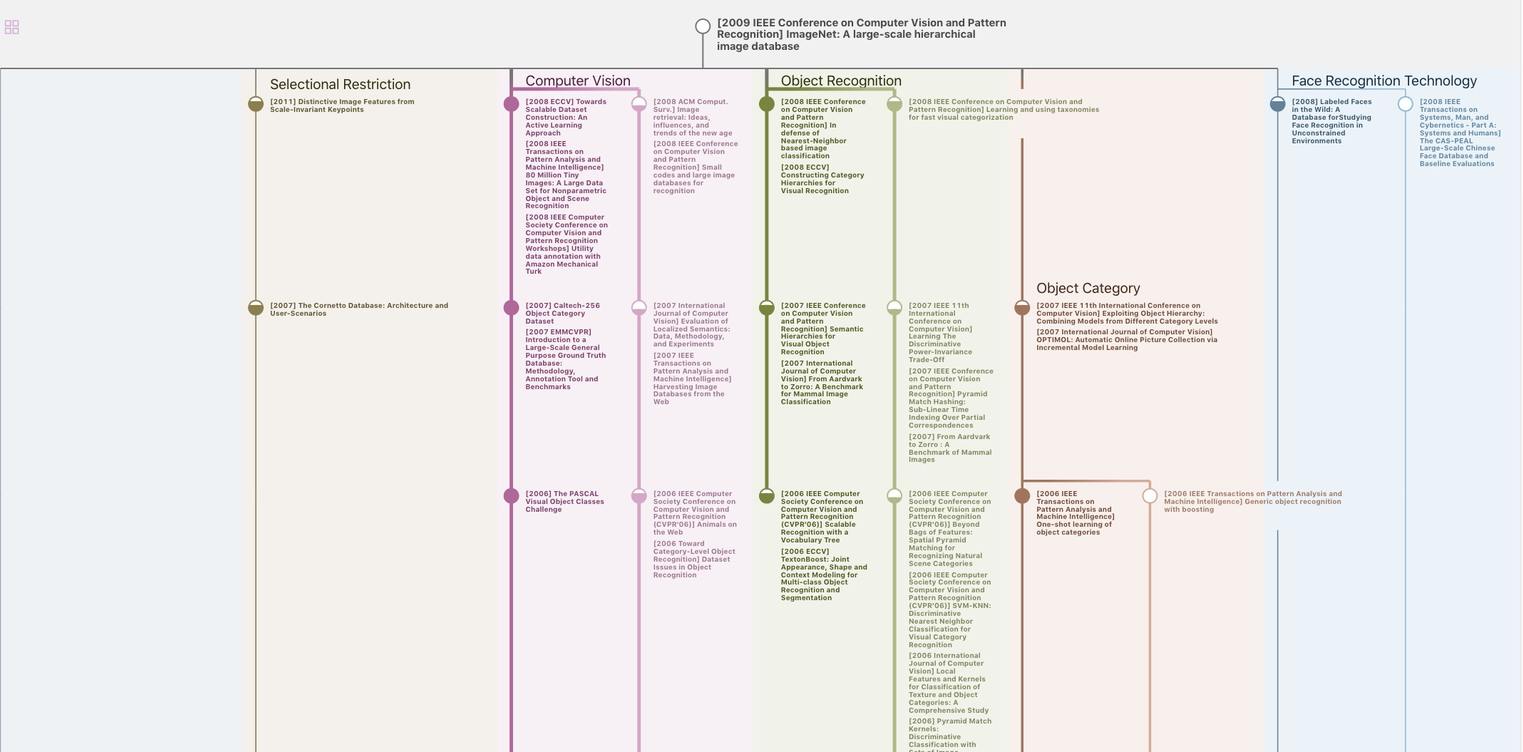
生成溯源树,研究论文发展脉络
Chat Paper
正在生成论文摘要