CheXstray: Real-time Multi-Modal Data Concordance for Drift Detection in Medical Imaging AI
arxiv(2022)
摘要
Rapidly expanding Clinical AI applications worldwide have the potential to impact to all areas of medical practice. Medical imaging applications constitute a vast majority of approved clinical AI applications. Though healthcare systems are eager to adopt AI solutions a fundamental question remains: \textit{what happens after the AI model goes into production?} We use the CheXpert and PadChest public datasets to build and test a medical imaging AI drift monitoring workflow that tracks data and model drift without contemporaneous ground truth. We simulate drift in multiple experiments to compare model performance with our novel multi-modal drift metric, which uses DICOM metadata, image appearance representation from a variational autoencoder (VAE), and model output probabilities as input. Through experimentation, we demonstrate a strong proxy for ground truth performance using unsupervised distributional shifts in relevant metadata, predicted probabilities, and VAE latent representation. Our key contributions include (1) proof-of-concept for medical imaging drift detection including use of VAE and domain specific statistical methods (2) a multi-modal methodology for measuring and unifying drift metrics (3) new insights into the challenges and solutions for observing deployed medical imaging AI (4) creation of open-source tools enabling others to easily run their own workflows or scenarios. This work has important implications for addressing the translation gap related to continuous medical imaging AI model monitoring in dynamic healthcare environments.
更多查看译文
关键词
drift detection,medical imaging ai,real-time,multi-modal
AI 理解论文
溯源树
样例
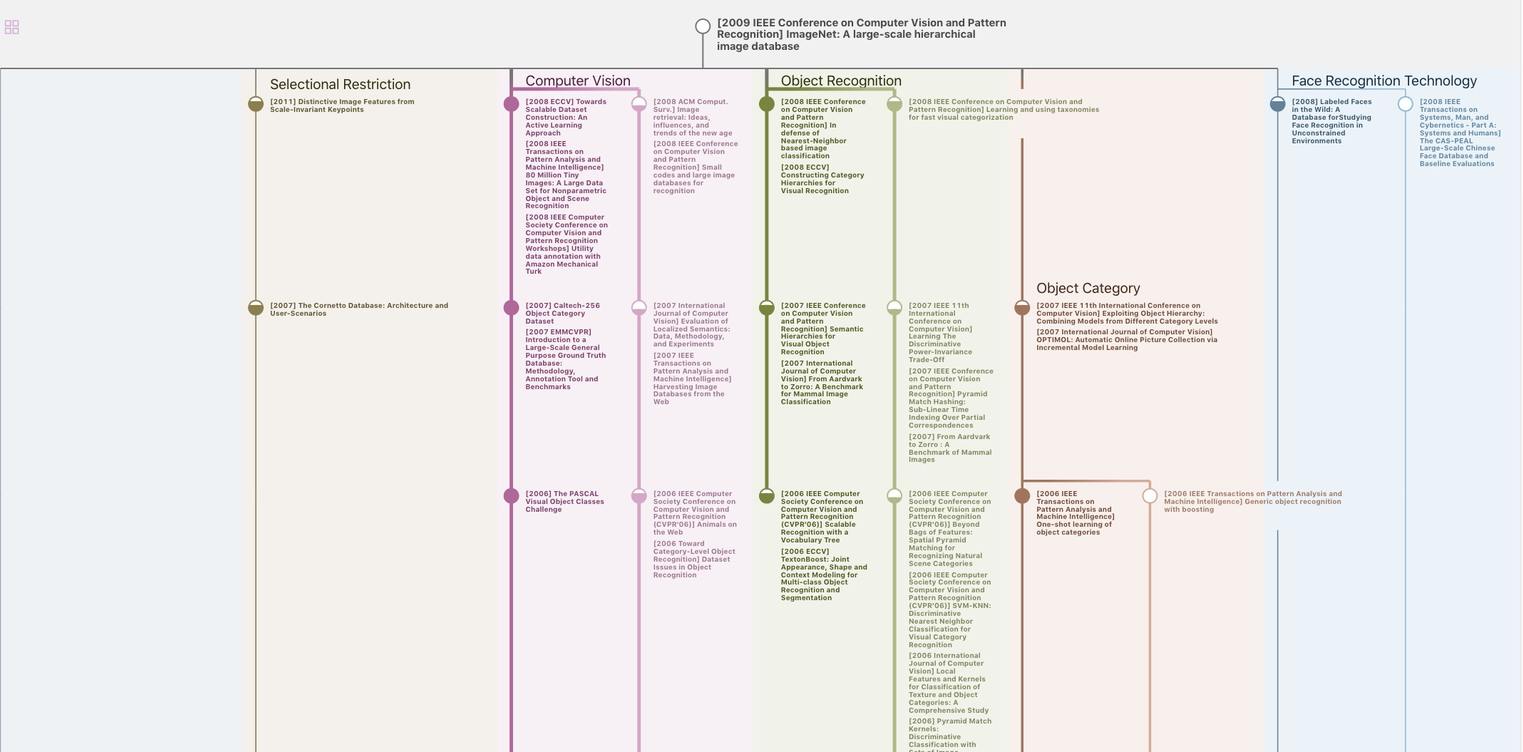
生成溯源树,研究论文发展脉络
Chat Paper
正在生成论文摘要