Machine learning-based approach KEVOLVE efficiently identifies SARS-CoV-2 variant-specific genomic signatures
biorxiv(2022)
摘要
Machine learning has proven to be a powerful tool for the identification of distinctive genomic signatures among viral sequences. Such signatures are motifs present in the viral genome that differentiate species or variants. In the context of SARS-CoV-2, the identification of such signatures can contribute to taxonomic and phylogenetic studies, help in recognizing and defining distinct emerging variants, and focus the characterization of functional properties of polymorphic gene products. Here, we study KEVOLVE, an approach based on a genetic algorithm with a machine learning kernel, to identify several genomic signatures based on minimal sets of k-mers. In a comparative study, in which we analyzed large SARS-CoV-2 genome dataset, KEVOLVE performed better in identifying variant-discriminative signatures than several gold-standard reference statistical tools. Subsequently, these signatures were characterized to highlight potential biological functions. The majority were associated with known mutations among the different variants, with respect to functional and pathological impact based on available literature. Notably, we found show evidence of new motifs, specifically in the Omicron variant, some of which include silent mutations, indicating potentially novel, variant-specific virulence determinants. The source code of the method and additional resources are available at: https://github.com/bioinfoUQAM/KEVOLVE.
### Competing Interest Statement
I have read the journal's policy and the authors of this manuscript have the following competing interests: [Soren Gantt receive research funds from Moderna for a COVID-19 vaccine trial, as well as research and consulting fees from Moderna and Merck related to CMV vaccine development, and consulting fees from GSK related to vaccine safety Abdoulaye Banire Diallo is co-founder of My Intelligent Machines]
更多查看译文
AI 理解论文
溯源树
样例
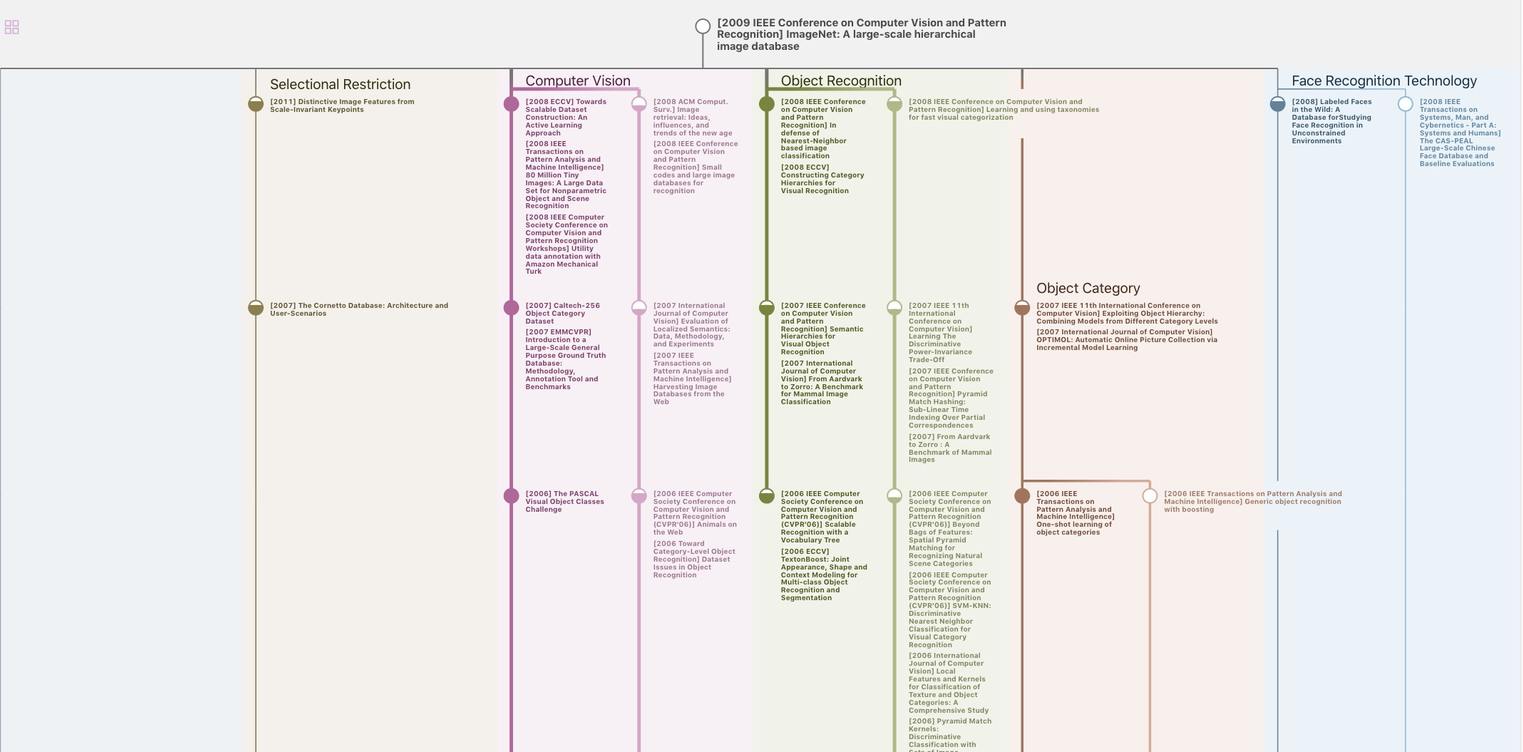
生成溯源树,研究论文发展脉络
Chat Paper
正在生成论文摘要