Counterfactual Multi-Token Fairness in Text Classification
arxiv(2022)
摘要
The counterfactual token generation has been limited to perturbing only a single token in texts that are generally short and single sentences. These tokens are often associated with one of many sensitive attributes. With limited counterfactuals generated, the goal to achieve invariant nature for machine learning classification models towards any sensitive attribute gets bounded, and the formulation of Counterfactual Fairness gets narrowed. In this paper, we overcome these limitations by solving root problems and opening bigger domains for understanding. We have curated a resource of sensitive tokens and their corresponding perturbation tokens, even extending the support beyond traditionally used sensitive attributes like \textit{Age}, \textit{Gender}, and \textit{Race} to \textit{Nationality}, \textit{Disability}, and \textit{Religion}. The concept of Counterfactual Generation has been extended to multi-token support valid over all forms of texts and documents. We define the method of generating counterfactuals by perturbing multiple sensitive tokens as \textbf{Counterfactual Multi-token Generation}. The method has been conceptualized to showcase significant performance improvement over single-token methods and validated over multiple benchmark datasets. The emendation in counterfactual generation propagates in achieving improved \textbf{Counterfactual Multi-token Fairness}.
更多查看译文
AI 理解论文
溯源树
样例
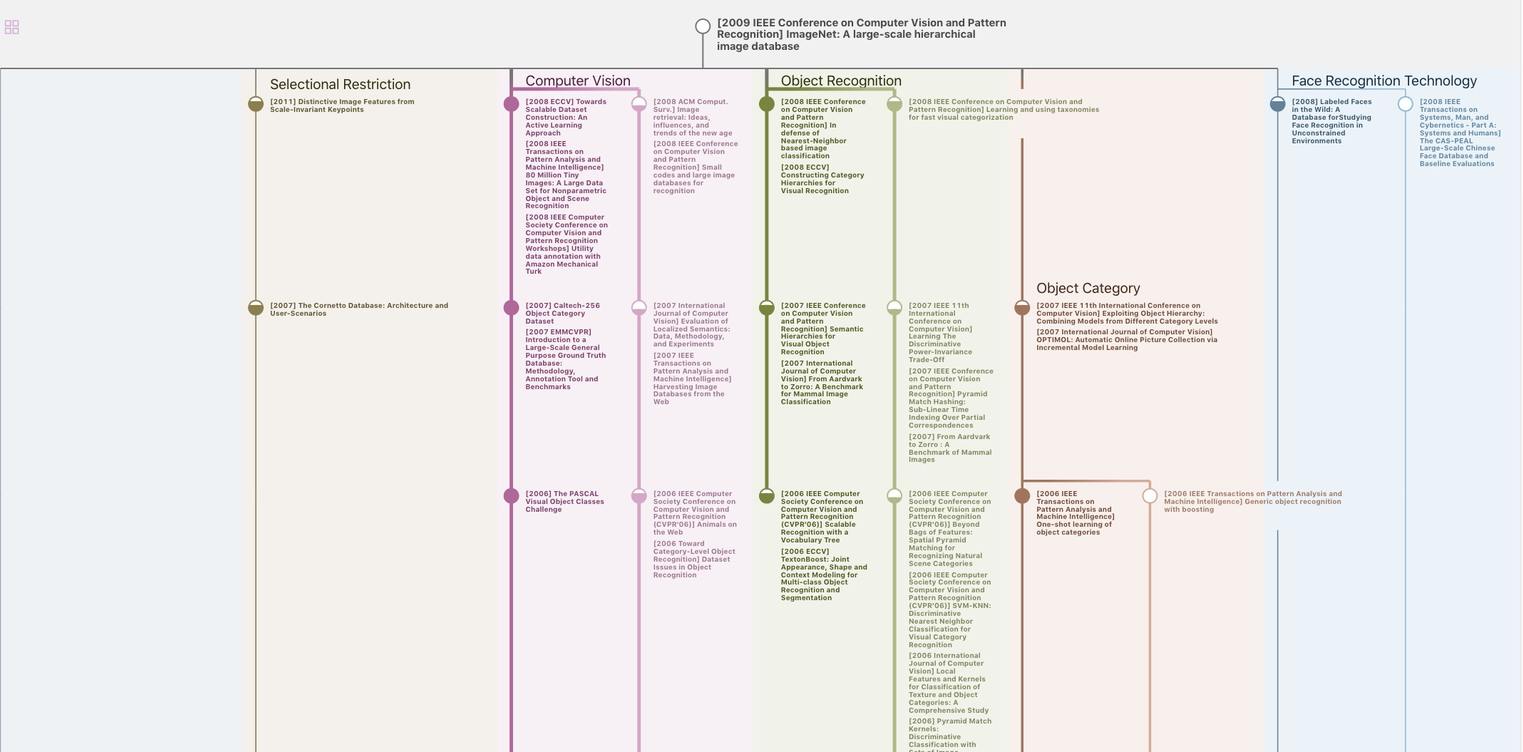
生成溯源树,研究论文发展脉络
Chat Paper
正在生成论文摘要