Development of a beta-Variational Autoencoder for Disentangled Latent Space Representation of Anterior Segment Optical Coherence Tomography Images
TRANSLATIONAL VISION SCIENCE & TECHNOLOGY(2022)
摘要
Purpose: To investigate the feasibility of extracting a low-dimensional latent structure of anterior segment optical coherence tomography (AS-OCT) images by use of a /3Methods: We retrospectively collected 2111 AS-OCT images from 2111 eyes of 1261 participants from the ongoing Asan Glaucoma Progression Study. After hyperparameter optimization, the images were analyzed with /3-VAE. Results: The mean participant age was 64.4 years, with mean values of visual field index and mean deviation of 86.4% and -5.33 dB, respectively. After experiments, a latent space size of 6 and /3 value of 53 were selected for latent space analysis with /3-VAE. Latent variables were successfully disentangled, showing readily interpretable distinct characteristics, such as the overall depth and area of the anterior chamber (771), pupil diameter (772), iris profile (773 and 774), and corneal curvature (775). Conclusions: /3-VAE can successfully be applied for disentangled latent space representation of AS-OCT images, revealing the high possibility of applying unsupervised learning in the medical image analysis. Translational Relevance: This study demonstrates that a deep learning-based latent space model can be applied for the analysis of AS-OCT images.
更多查看译文
关键词
anterior segment OCT, deep learning, artificial intelligence, 3-variational autoencoder
AI 理解论文
溯源树
样例
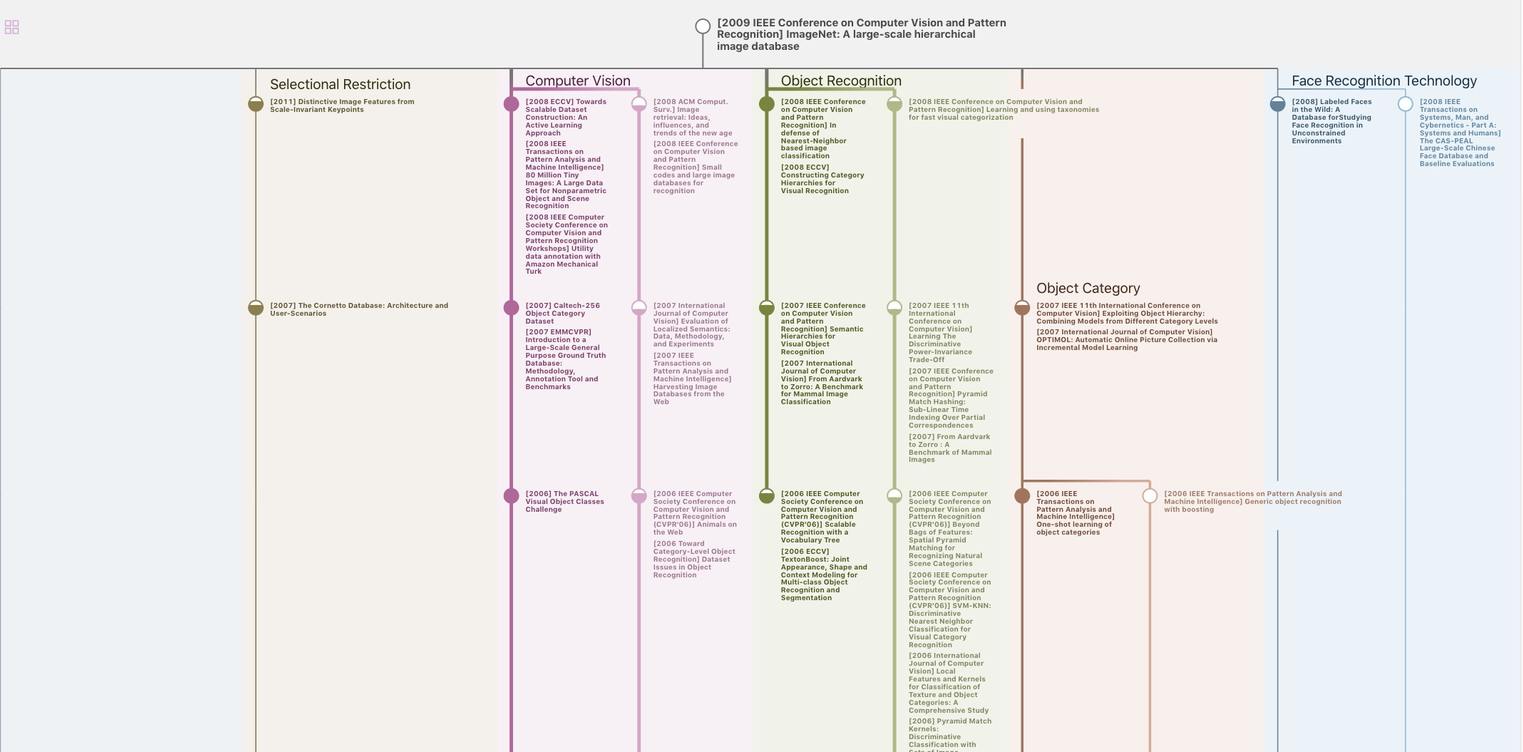
生成溯源树,研究论文发展脉络
Chat Paper
正在生成论文摘要