Leveraging Experience in Lifelong Multi-Agent Pathfinding.
Symposium on Combinatorial Search (SOCS)(2022)
摘要
In Lifelong Multi-Agent Path Finding (L-MAPF) a team of agents performs a stream of tasks consisting of multiple locations to be visited by the agents on a shared graph while avoiding collisions with one another. L-MAPF is typically tackled by partitioning it into multiple consecutive, and hence similar, "one-shot" MAPF queries with a single task assigned to each agent, as in the Rolling-Horizon Collision Resolution (RHCR) algorithm. Thus, a solution to one query informs the next query, which leads to similarity with respect to the agents' start and goal positions, and how collisions need to be resolved from one query to the next. Thus, experience from solving one MAPF query can potentially be used to speedup solving the next one. Despite this intuition, current L-MAPF planners solve consecutive MAPF queries from scratch. In this paper, we introduce a new RHCR-inspired approach called exRHCR, which exploits experience in its constituent MAPF queries. In particular, exRHCR employs a new extension of Priority-Based Search (PBS), a state-of-the-art MAPF solver. Our extension, called exPBS, allows to warm-start the search with the priorities between agents used by PBS in the previous MAPF instances. We demonstrate empirically that exRHCR solves L-MAPF up to 25% faster than RHCR, and allows to increase throughput for given task streams by as much as 3%-16% by increasing the number of agents we can cope with for a given time budget.
更多查看译文
关键词
experience,multi-agent
AI 理解论文
溯源树
样例
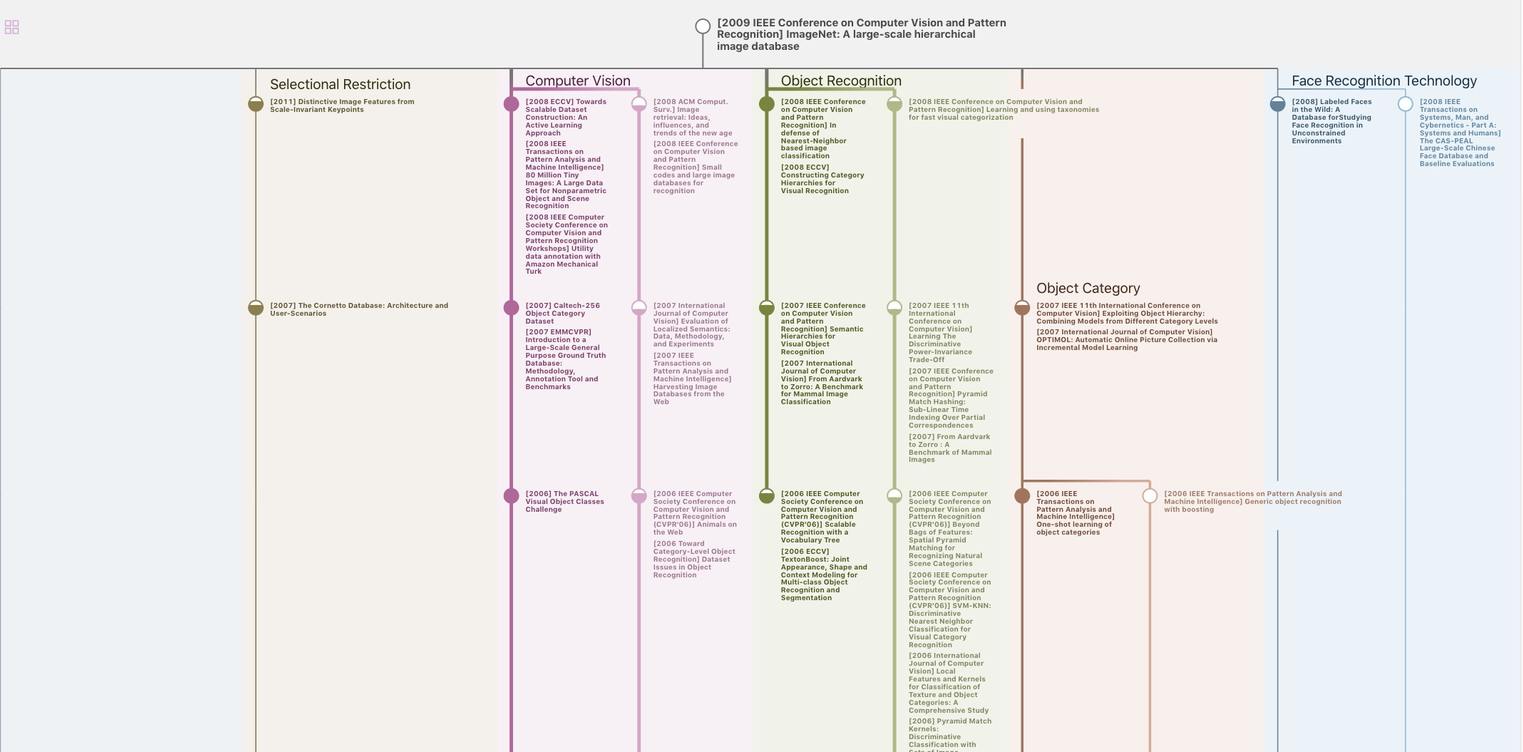
生成溯源树,研究论文发展脉络
Chat Paper
正在生成论文摘要