Reducing redundancy in the bottleneck representation of autoencoders
PATTERN RECOGNITION LETTERS(2024)
摘要
Autoencoders (AEs) are a type of unsupervised neural networks, which can be used to solve various tasks, e.g., dimensionality reduction, image compression, and image denoising. An AE has two goals: (i) compress the original input to a low -dimensional space at the bottleneck of the network topology using an encoder, (ii) reconstruct the input from the representation at the bottleneck using a decoder. Both encoder and decoder are optimized jointly by minimizing a distortion -based loss which implicitly forces the model to keep only the information in input data required to reconstruct them and to reduce redundancies. In this paper, we propose a scheme to explicitly penalize feature redundancies in the bottleneck representation. To this end, we propose an additional loss term, based on the pairwise covariances of the network units, which complements the data reconstruction loss forcing the encoder to learn a more diverse and richer representation of the input. We tested our approach across different tasks, namely dimensionality reduction, image compression, and image denoising. Experimental results show that the proposed loss leads consistently to superior performance compared to using the standard AE loss.
更多查看译文
关键词
Autoencoders,Unsupervised learning,Diversity,Feature representation,Dimensionality reduction,Image denoising,Image compression
AI 理解论文
溯源树
样例
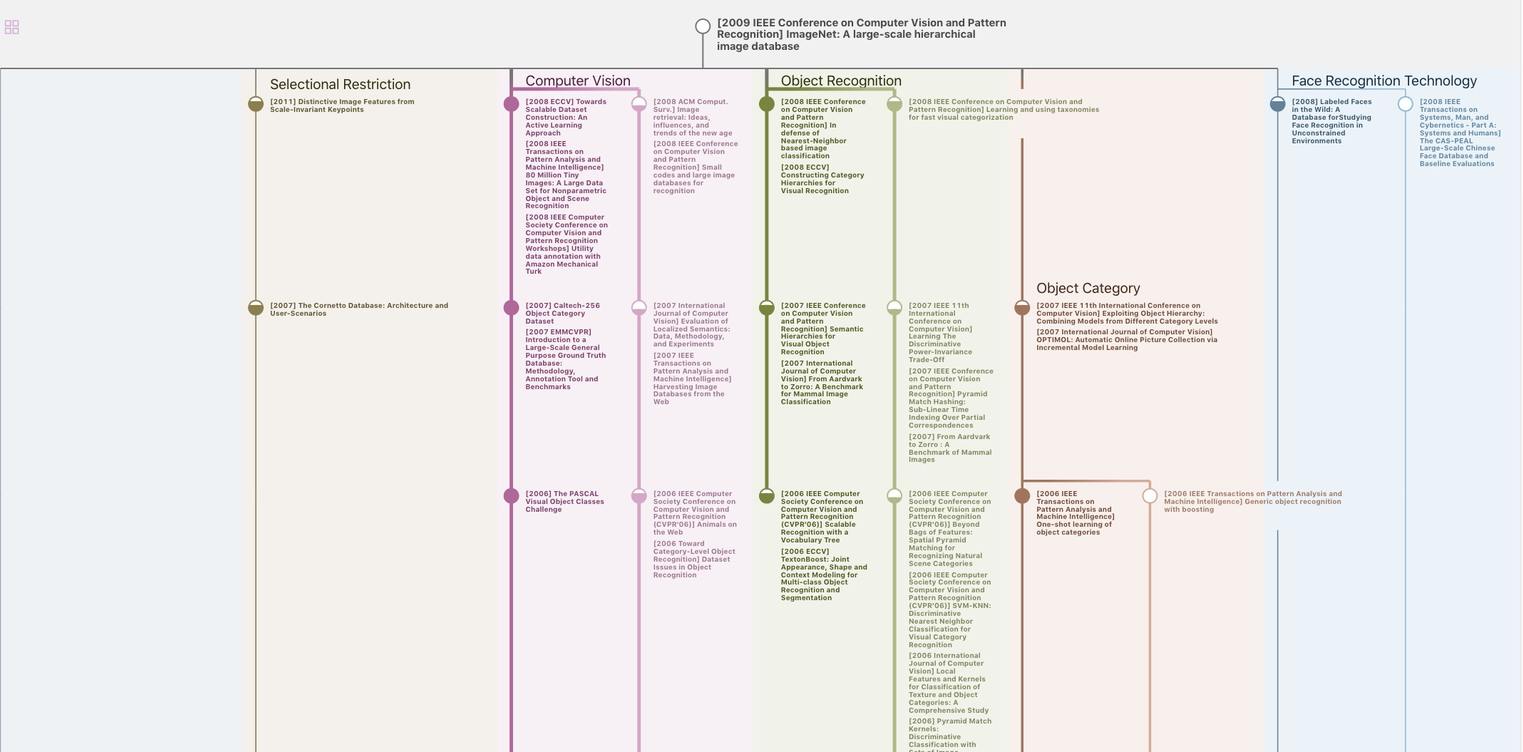
生成溯源树,研究论文发展脉络
Chat Paper
正在生成论文摘要