Towards Characterizing the Semantic Robustness of Face Recognition
arxiv(2023)
摘要
Deep Neural Networks (DNNs) lack robustness against imperceptible perturbations to their input. Face Recognition Models (FRMs) based on DNNs inherit this vulnerability. We propose a methodology for assessing and characterizing the robustness of FRMs against semantic perturbations to their input. Our methodology causes FRMs to malfunction by designing adversarial attacks that search for identity-preserving modifications to faces. In particular, given a face, our attacks find identity-preserving variants of the face such that an FRM fails to recognize the images belonging to the same identity. We model these identity-preserving semantic modifications via direction- and magnitude-constrained perturbations in the latent space of StyleGAN. We further propose to characterize the semantic robustness of an FRM by statistically describing the perturbations that induce the FRM to malfunction. Finally, we combine our methodology with a certification technique, thus providing (i) theoretical guarantees on the performance of an FRM, and (ii) a formal description of how an FRM may model the notion of face identity.
更多查看译文
关键词
adversarial attacks,certification technique,deep neural networks,direction-constrained perturbations,DNNs,face identity,face recognition models,FRM,identity-preserving semantic modifications,identity-preserving variants,image recognition,imperceptible perturbations,latent space,magnitude-constrained perturbations,semantic perturbations,semantic robustness,StyleGAN
AI 理解论文
溯源树
样例
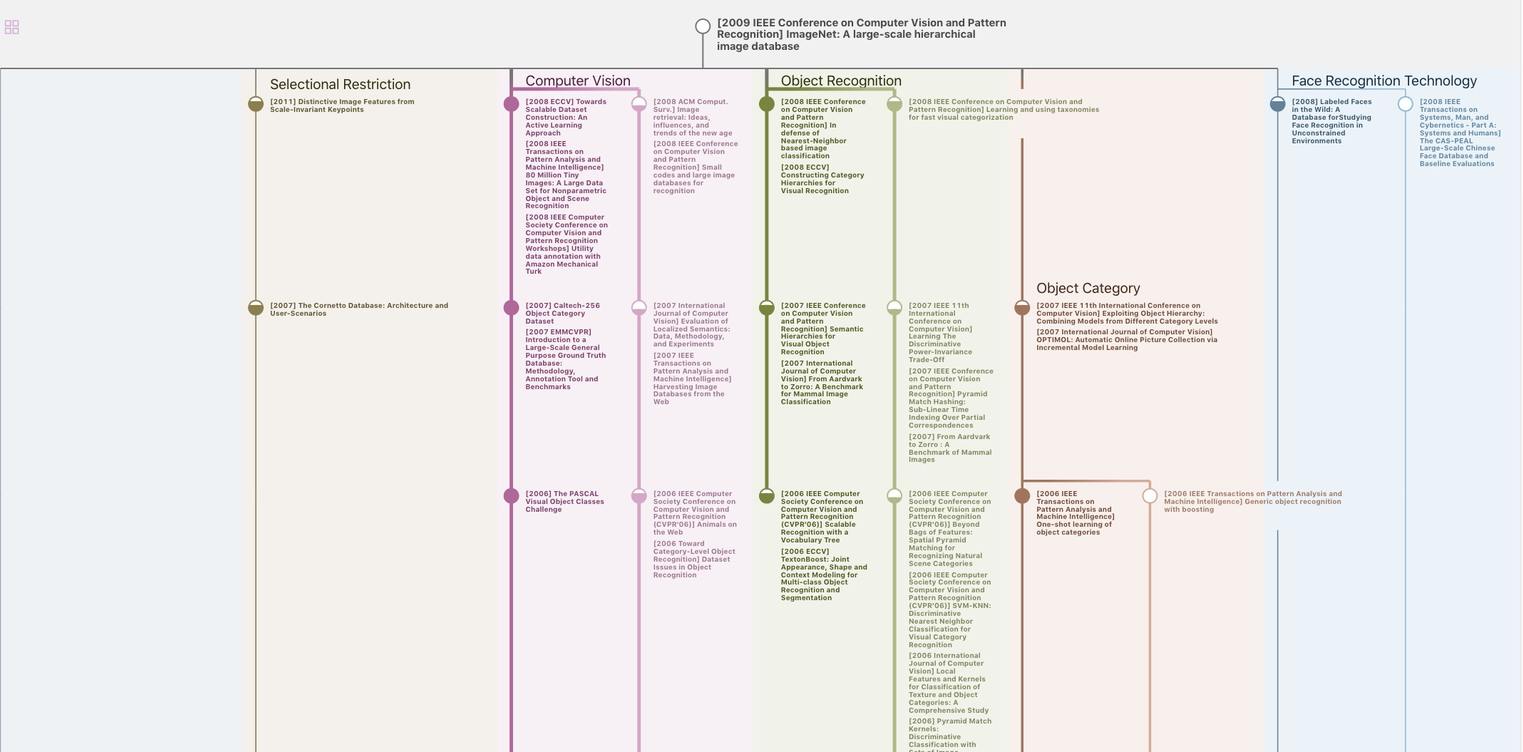
生成溯源树,研究论文发展脉络
Chat Paper
正在生成论文摘要