Computational analysis and prediction of PE_PGRS proteins using machine learning.
Computational and structural biotechnology journal(2022)
摘要
genome comprises approximately 10% of two families of poorly characterised genes due to their high GC content and highly repetitive nature. The largest sub-group, the proline-glutamic acid polymorphic guanine-cytosine-rich sequence (PE_PGRS) family, is thought to be involved in host response and disease pathogenicity. Due to their high genetic variability and complexity of analysis, they are typically disregarded for further research in genomic studies. There are currently limited online resources and homology computational tools that can identify and analyse PE_PGRS proteins. In addition, they are computational-intensive and time-consuming, and lack sensitivity. Therefore, computational methods that can rapidly and accurately identify PE_PGRS proteins are valuable to facilitate the functional elucidation of the PE_PGRS family proteins. In this study, we developed the first machine learning-based bioinformatics approach, termed PEPPER, to allow users to identify PE_PGRS proteins rapidly and accurately. PEPPER was built upon a comprehensive evaluation of 13 popular machine learning algorithms with various sequence and physicochemical features. Empirical studies demonstrated that PEPPER achieved significantly better performance than alignment-based approaches, BLASTP and PHMMER, in both prediction accuracy and speed. PEPPER is anticipated to facilitate community-wide efforts to conduct high-throughput identification and analysis of PE_PGRS proteins.
更多查看译文
关键词
Bioinformatics,Machine learning,Mycobacterial,PE_PGRS,Sequence analysis
AI 理解论文
溯源树
样例
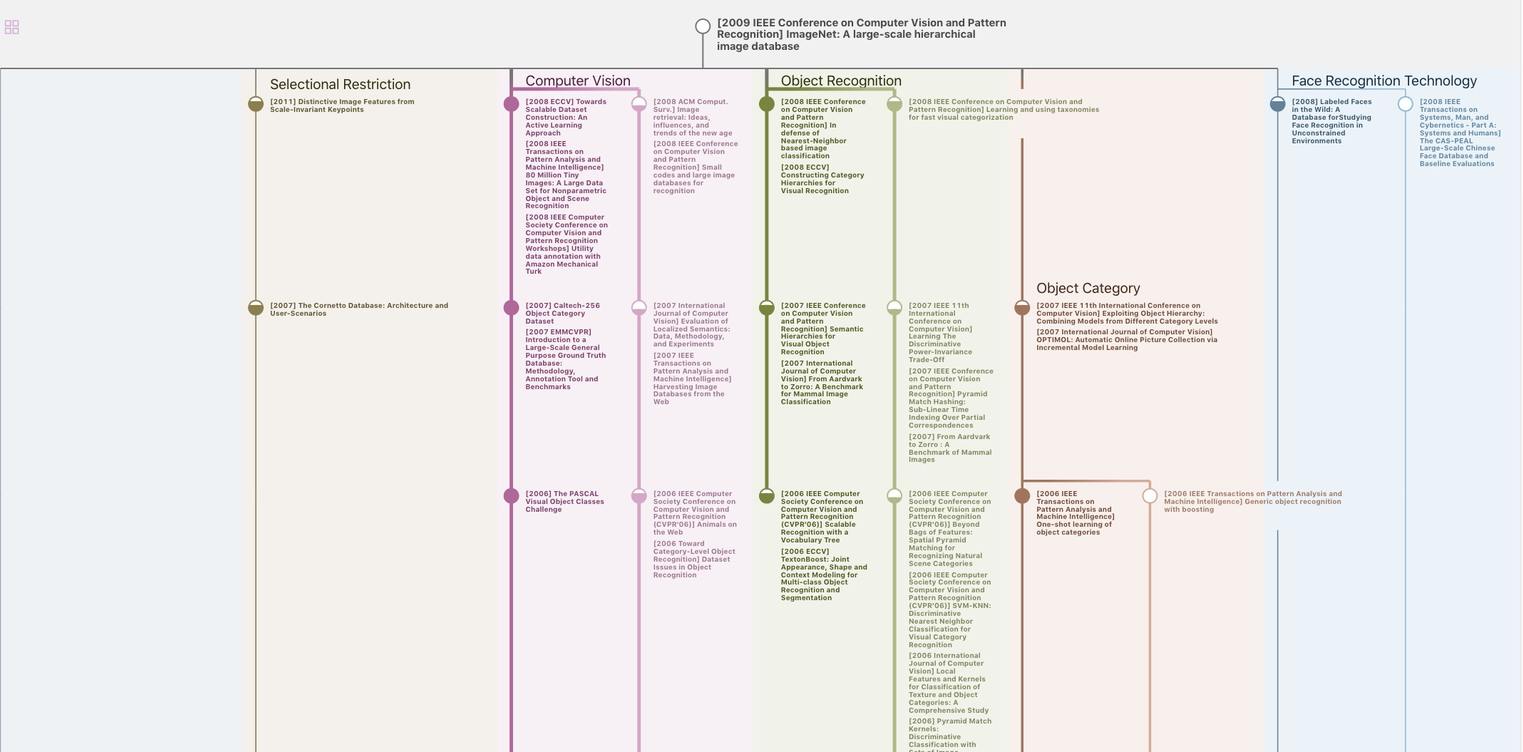
生成溯源树,研究论文发展脉络
Chat Paper
正在生成论文摘要