Optimal PET-based radiomic signature construction based on the cross-combination method for predicting the survival of patients with diffuse large B-cell lymphoma
European Journal of Nuclear Medicine and Molecular Imaging(2022)
摘要
Purpose To develop and externally validate models incorporating a PET radiomics signature (R-signature) obtained by the cross-combination method for predicting the survival of patients with diffuse large B-cell lymphoma (DLBCL). Methods A total of 383 patients with DLBCL from two medical centres between 2011 and 2019 were included. The cross-combination method was used on three types of PET radiomics features from the training cohort to generate 49 feature selection-classification candidates based on 7 different machine learning models. The R-signature was then built by selecting the optimal candidates based on their progression-free survival (PFS) and overall survival (OS). Cox regression analysis was used to develop the survival prediction models. The calibration, discrimination, and clinical utility of the models were assessed and externally validated. Results The R-signatures determined by 12 and 31 radiomics features were significantly associated with PFS and OS, respectively ( P <0.05). The combined models that incorporated R-signatures, metabolic metrics, and clinical risk factors exhibited significant prognostic superiority over the clinical models, PET-based models, and the National Comprehensive Cancer Network International Prognostic Index in terms of both PFS (C-index: 0.801 vs. 0.732 vs. 0.785 vs. 0.720, respectively) and OS (C-index: 0.807 vs. 0.740 vs. 0.773 vs. 0.726, respectively). For external validation, the C-indices were 0.758 vs. 0.621 vs. 0.732 vs. 0.673 and 0.794 vs. 0.696 vs. 0.781 vs. 0.708 in the PFS and OS analyses, respectively. The calibration curves showed good consistency, and the decision curve analysis supported the clinical utility of the combined model. Conclusion The R-signature could be used as a survival predictor for DLBCL, and its combination with clinical factors may allow for accurate risk stratification.
更多查看译文
关键词
[18F]-FDG PET/CT,Diffuse large B-cell lymphoma,Prognosis,Machine learning,Radiomics
AI 理解论文
溯源树
样例
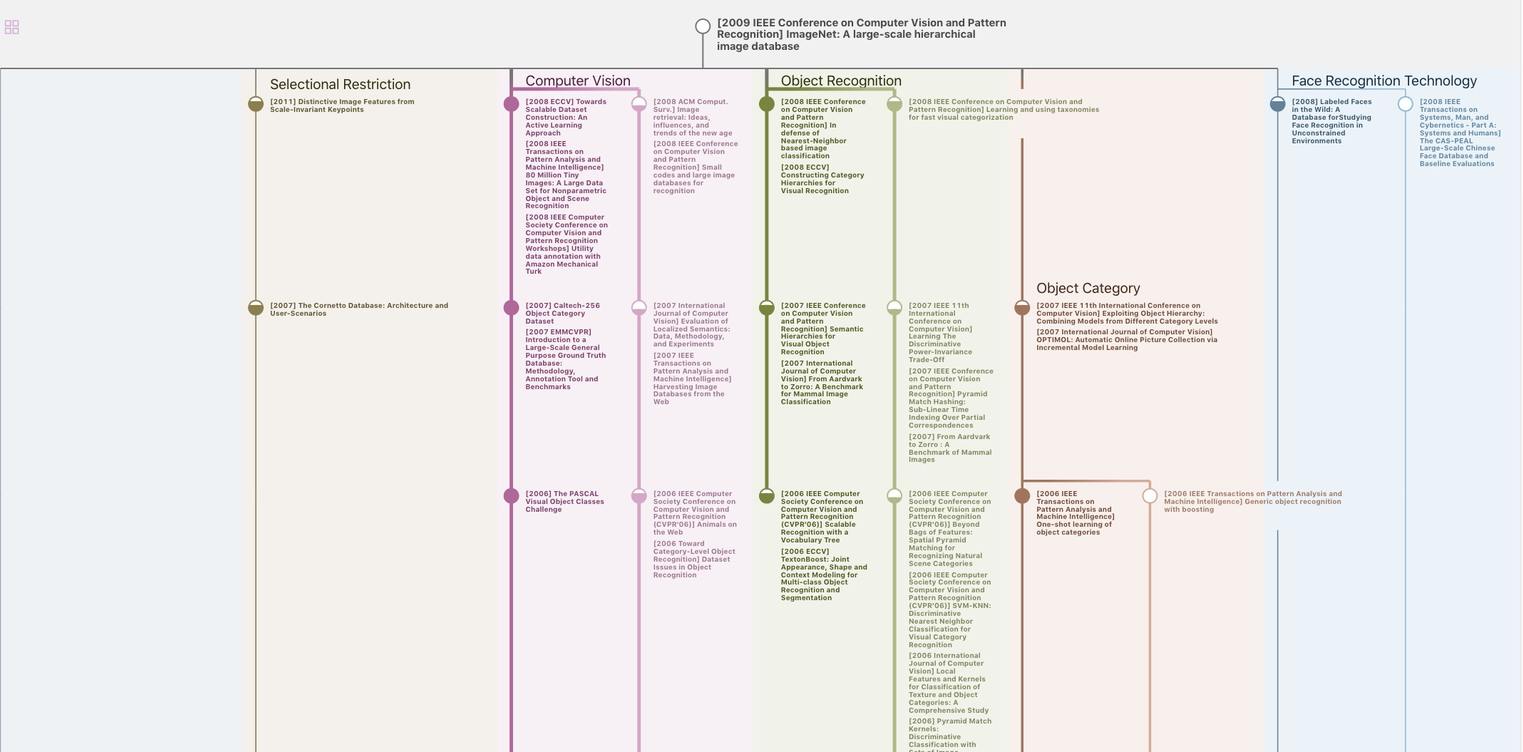
生成溯源树,研究论文发展脉络
Chat Paper
正在生成论文摘要