Subtype classification of malignant lymphoma using immunohistochemical staining pattern
International Journal of Computer Assisted Radiology and Surgery(2022)
摘要
Purpose For the image classification problem, the construction of appropriate training data is important for improving the generalization ability of the classifier in particular when the size of the training data is small. We propose a method that quantitatively evaluates the typicality of a hematoxylin-and-eosin (H&E)-stained tissue slide from a set of immunohistochemical (IHC) stains and applies the typicality to instance selection for the construction of classifiers that predict the subtype of malignant lymphoma to improve the generalization ability. Methods We define the typicality of the H&E-stained tissue slides by the ratio of the probability density of the IHC staining patterns on low-dimensional embedded space. Employing a multiple-instance-learning-based convolutional neural network for the construction of the subtype classifier without the annotations indicating cancerous regions in whole slide images, we select the training data by referring to the evaluated typicality to improve the generalization ability. We demonstrate the effectiveness of the instance selection based on the proposed typicality in a three-class subtype classification of 262 malignant lymphoma cases. Results In the experiment, we confirmed that the subtypes of typical instances could be predicted more accurately than those of atypical instances. Furthermore, it was confirmed that instance selection for the training data based on the proposed typicality improved the generalization ability of the classifier, wherein the classification accuracy was improved from 0.664 to 0.683 compared with the baseline method when the training data was constructed focusing on typical instances. Conclusion The experimental results showed that the typicality of the H&E-stained tissue slides computed from IHC staining patterns is useful as a criterion for instance selection to enhance the generalization ability, and this typicality could be employed for instance selection under some practical limitations.
更多查看译文
关键词
Digital pathology, Image classification, Typicality, Malignant lymphoma, Instance selection
AI 理解论文
溯源树
样例
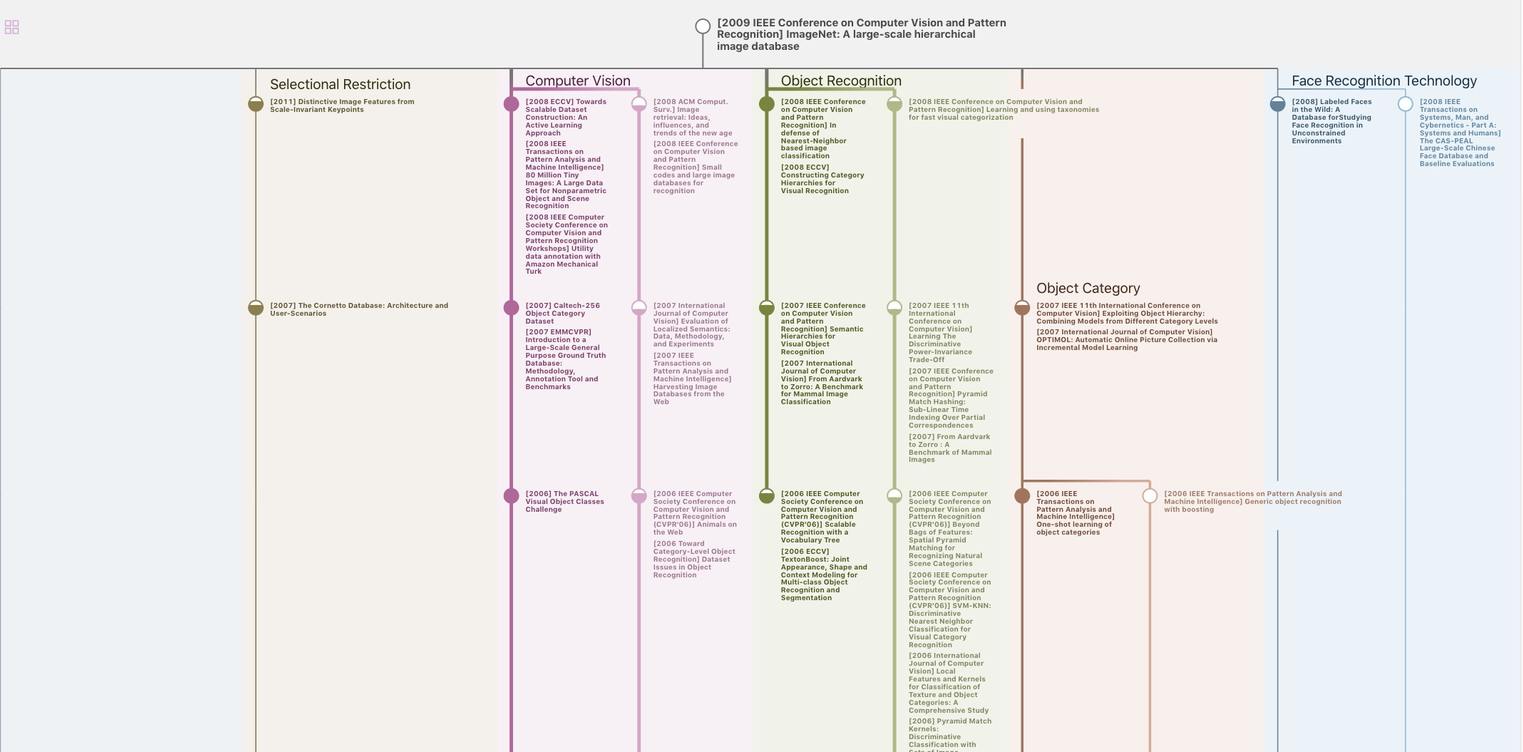
生成溯源树,研究论文发展脉络
Chat Paper
正在生成论文摘要