Automated Architecture Search for Brain-inspired Hyperdimensional Computing
arxiv(2022)
摘要
This paper represents the first effort to explore an automated architecture search for hyperdimensional computing (HDC), a type of brain-inspired neural network. Currently, HDC design is largely carried out in an application-specific ad-hoc manner, which significantly limits its application. Furthermore, the approach leads to inferior accuracy and efficiency, which suggests that HDC cannot perform competitively against deep neural networks. Herein, we present a thorough study to formulate an HDC architecture search space. On top of the search space, we apply reinforcement-learning to automatically explore the HDC architectures. The searched HDC architectures show competitive performance on case studies involving a drug discovery dataset and a language recognition task. On the Clintox dataset, which tries to learn features from developed drugs that passed/failed clinical trials for toxicity reasons, the searched HDC architecture obtains the state-of-the-art ROC-AUC scores, which are 0.80% higher than the manually designed HDC and 9.75% higher than conventional neural networks. Similar results are achieved on the language recognition task, with 1.27% higher performance than conventional methods.
更多查看译文
关键词
automated architecture search,hyperdimensional
AI 理解论文
溯源树
样例
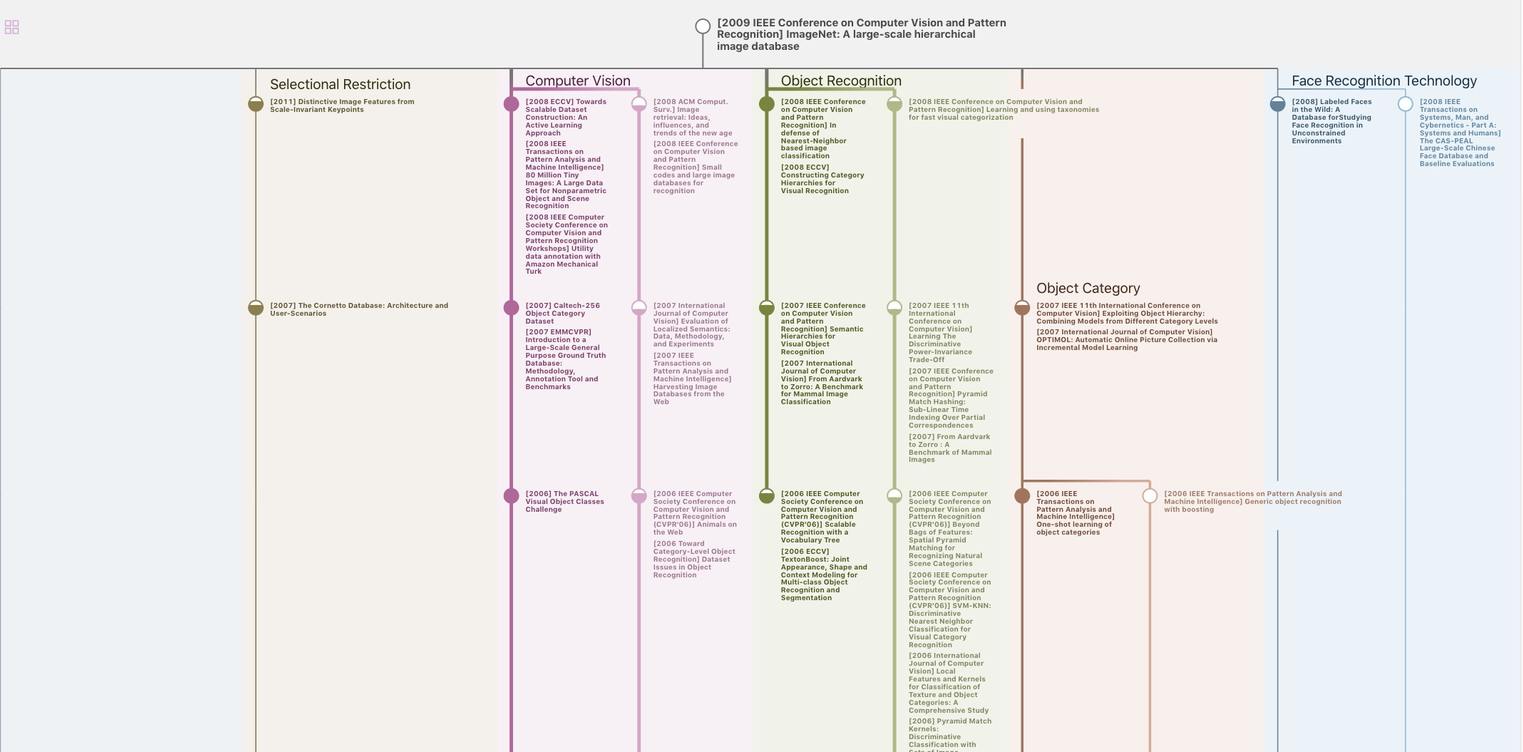
生成溯源树,研究论文发展脉络
Chat Paper
正在生成论文摘要