Expectation consistent plug-and-play for mri.
Proceedings of the ... IEEE International Conference on Acoustics, Speech, and Signal Processing. ICASSP (Conference)(2022)
摘要
For image recovery problems, plug-and-play (PnP) methods have been developed that replace the proximal step in an optimization algorithm with a call to an application-specific denoiser, often implemented using a deep neural network. Although such methods have been successful, they can be improved. For example, the denoiser is often trained using white Gaussian noise, while PnP's denoiser input error is often far from white and Gaussian, with statistics that are difficult to predict from iteration to iteration. PnP methods based on approximate message passing (AMP) are an exception, but only when the forward operator behaves like a large random matrix. In this work, we design a PnP method using the expectation consistent (EC) approximation algorithm, a generalization of AMP, that offers predictable error statistics at each iteration, from which a deep-net denoiser can be effectively trained.
更多查看译文
关键词
approximate message passing,AMP,expectation consistent approximation algorithm,predictable error statistics,iteration,deep-net denoiser,MRI,image recovery problems,proximal step,optimization algorithm,application-specific denoiser,deep neural network,white Gaussian noise,expectation consistent plug-and-play,PnP denoiser input error,forward operator,random matrix
AI 理解论文
溯源树
样例
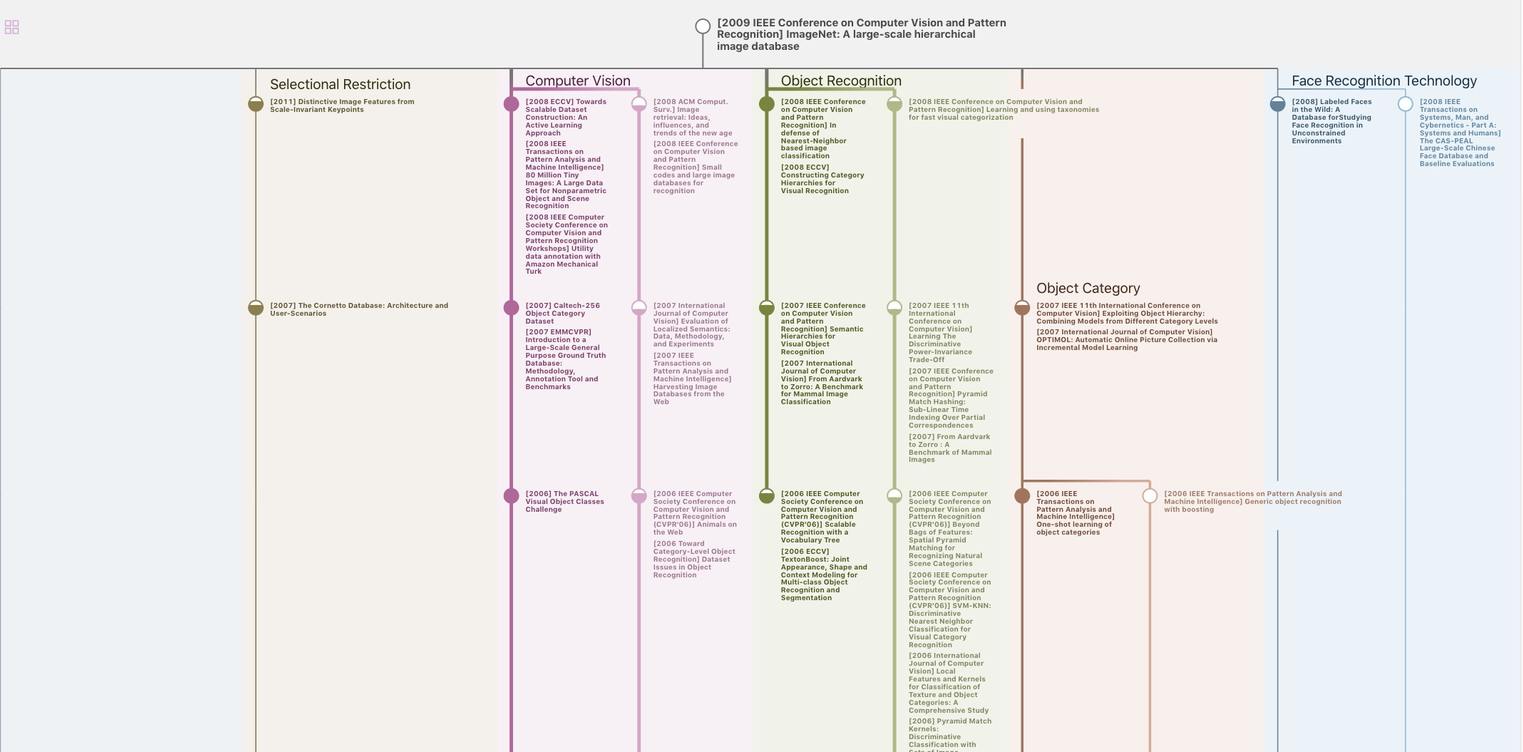
生成溯源树,研究论文发展脉络
Chat Paper
正在生成论文摘要