Causal associations of genetic factors with clinical progression in amyotrophic lateral sclerosis
Computer Methods and Programs in Biomedicine(2022)
摘要
Background and objective
Recent advances in the genetic causes of ALS reveals that about 10% of ALS patients have a genetic origin and that more than 30 genes are likely to contribute to this disease. However, four genes are more frequently associated with ALS: C9ORF72, TARDBP, SOD1, and FUS. The relationship between genetic factors and ALS progression rate is not clear. In this study, we carried out a causal analysis of ALS disease with a genetics perspective in order to assess the contribution of the four mentioned genes to the progression rate of ALS.
Methods
In this work, we applied a novel causal learning model to the CRESLA dataset which is a longitudinal clinical dataset of ALS patients including genetic information of such patients. This study aims to discover the relationship between four mentioned genes and ALS progression rate from a causation perspective using machine learning and probabilistic methods.
Results
The results indicate a meaningful association between genetic factors and ALS progression rate with causality viewpoint. Our findings revealed that causal relationships between ALSFRS-R items associated with bulbar regions have the strongest association with genetic factors, especially C9ORF72; and other three genes have the greatest contribution to the respiratory ALSFRS-R items with a causation point of view.
Conclusions
The findings revealed that genetic factors have a significant causal effect on the rate of ALS progression. Since C9ORF72 patients have higher proportion compared to those carrying other three gene mutations in the CRESLA cohort, we need a large multi-centric study to better analyze SOD1, TARDBP and FUS contribution to the ALS clinical progression. We conclude that causal associations between ALSFRS-R clinical factors is a suitable predictor for designing a prognostic model of ALS.
更多查看译文
关键词
Amyotrophic lateral sclerosis,Longitudinal analysis,Machine learning,Prognosis,Causal discovery
AI 理解论文
溯源树
样例
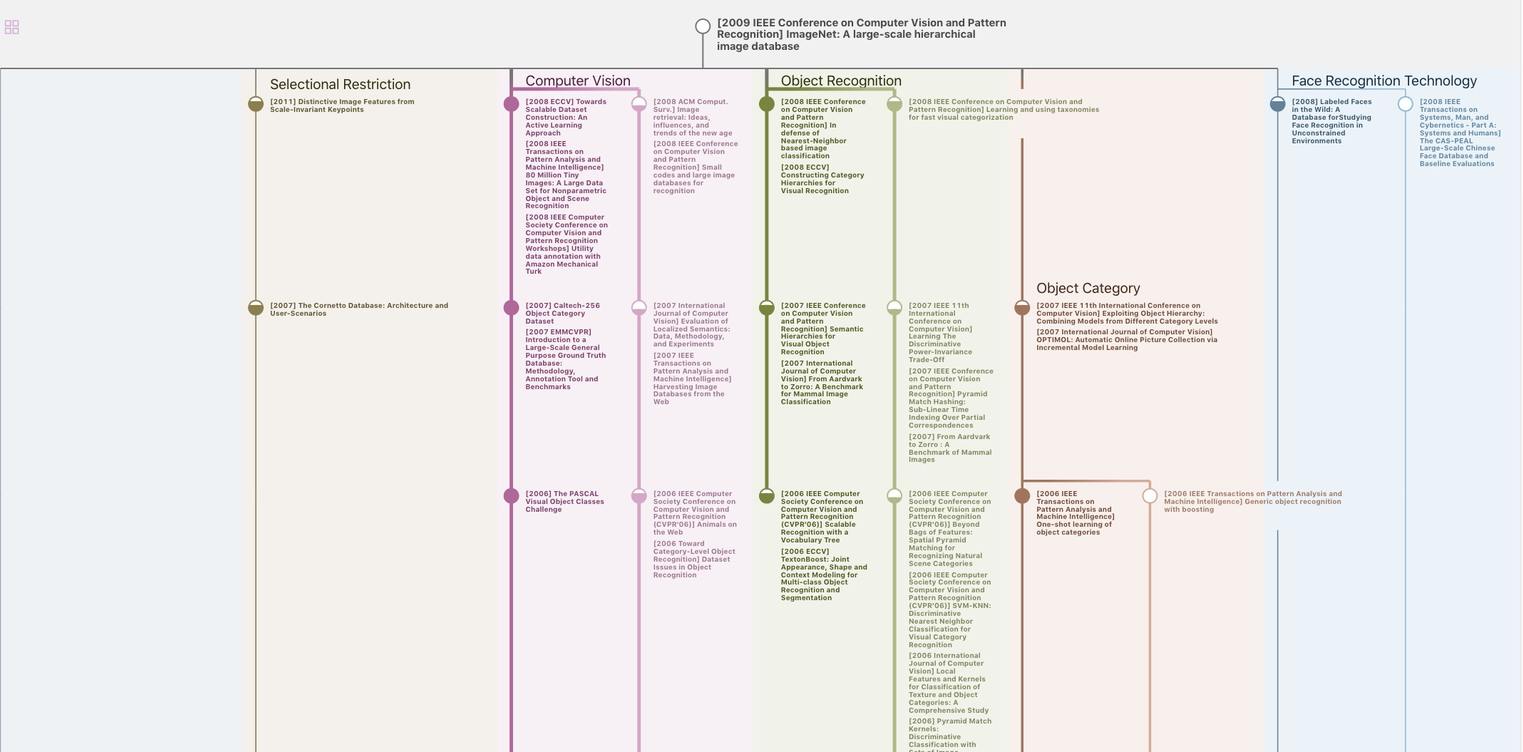
生成溯源树,研究论文发展脉络
Chat Paper
正在生成论文摘要