Resolving combinatorial ambiguities in dilepton t(t)over-bar event topologies with neural networks
PHYSICAL REVIEW D(2022)
摘要
We study the potential of deep learning to resolve the combinatorial problem in supersymmetrylike events with two invisible particles at the LHC. As a concrete example, we focus on dileptonic (tt) over bar events, where the combinatorial problem becomes an issue of binary classification: pairing the correct lepton with each b quark coming from the decays of the tops. We investigate the performance of a number of machine learning algorithms, including attention-based networks, which have been used for a similar problem in the fully hadronic channel of (tt) over bar production, and the Lorentz Boost Network, which is motivated by physics principles. We then consider the general case when the underlying mass spectrum is unknown, and hence no kinematic end point information is available. Compared against existing methods based on kinematic variables, we demonstrate that the efficiency for selecting the correct pairing is greatly improved by utilizing deep learning techniques.
更多查看译文
AI 理解论文
溯源树
样例
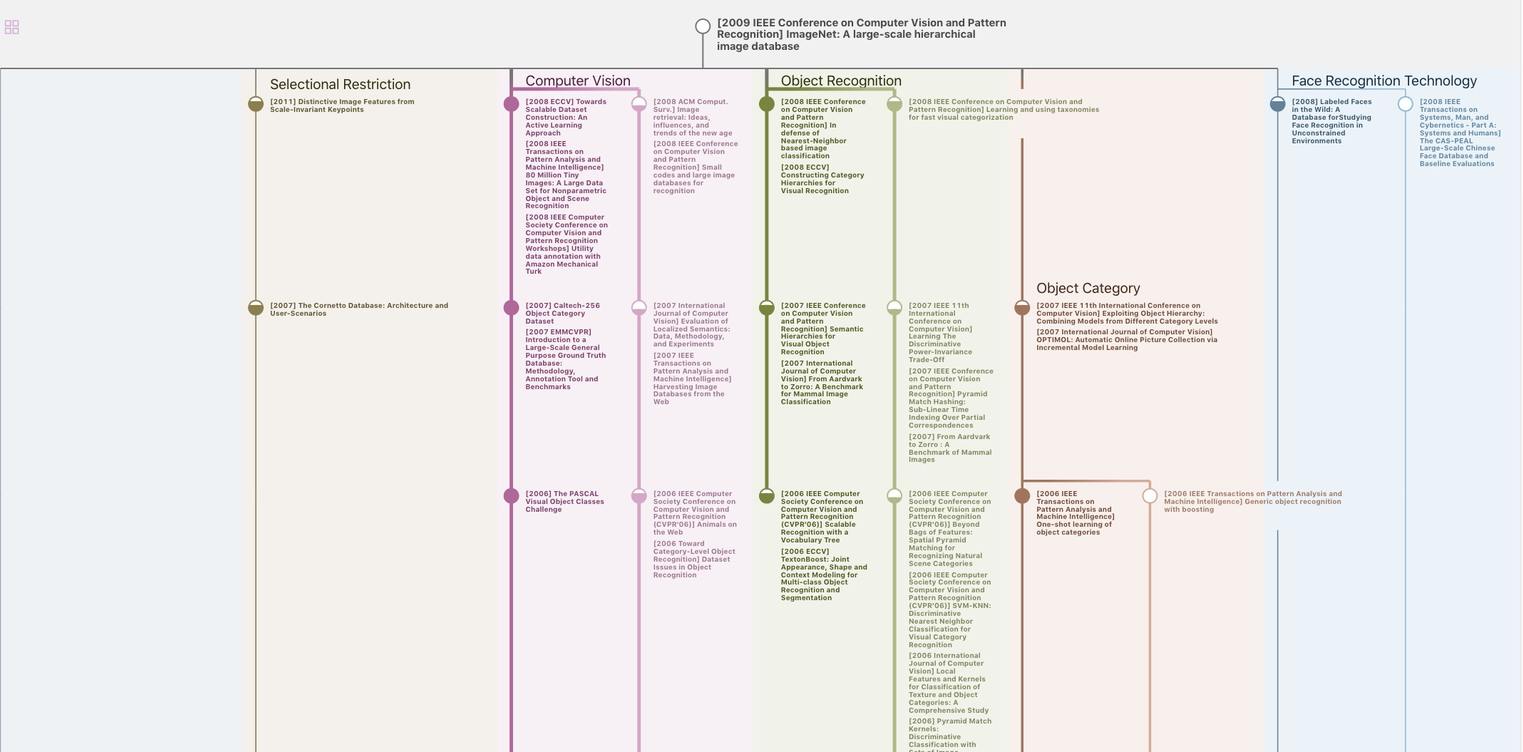
生成溯源树,研究论文发展脉络
Chat Paper
正在生成论文摘要