Escaping Saddle Points with Bias-Variance Reduced Local Perturbed SGD for Communication Efficient Nonconvex Distributed Learning
NeurIPS 2022(2022)
摘要
In recent centralized nonconvex distributed learning and federated learning, local methods are one of the promising approaches to reduce communication time. However, existing work has mainly focused on studying first-order optimality guarantees. On the other side, second-order optimality guaranteed algorithms have been extensively studied in the non-distributed optimization literature. In this paper, we study a new local algorithm called Bias-Variance Reduced Local Perturbed SGD (BVR-L-PSGD), that combines the existing bias-variance reduced gradient estimator with parameter perturbation to find second-order optimal points in centralized nonconvex distributed optimization. BVR-L-PSGD enjoys second-order optimality with nearly the same communication complexity as the best known one of BVR-L-SGD to find first-order optimality. Particularly, the communication complexity is better than non-local methods when the local datasets heterogeneity is smaller than the smoothness of the local loss. In an extreme case, the communication complexity approaches to $\widetilde \Theta(1)$ when the local datasets heterogeneity goes to zero.
更多查看译文
关键词
nonconvex optimization,distributed learning,local SGD,escaping saddle points,communication efficiency
AI 理解论文
溯源树
样例
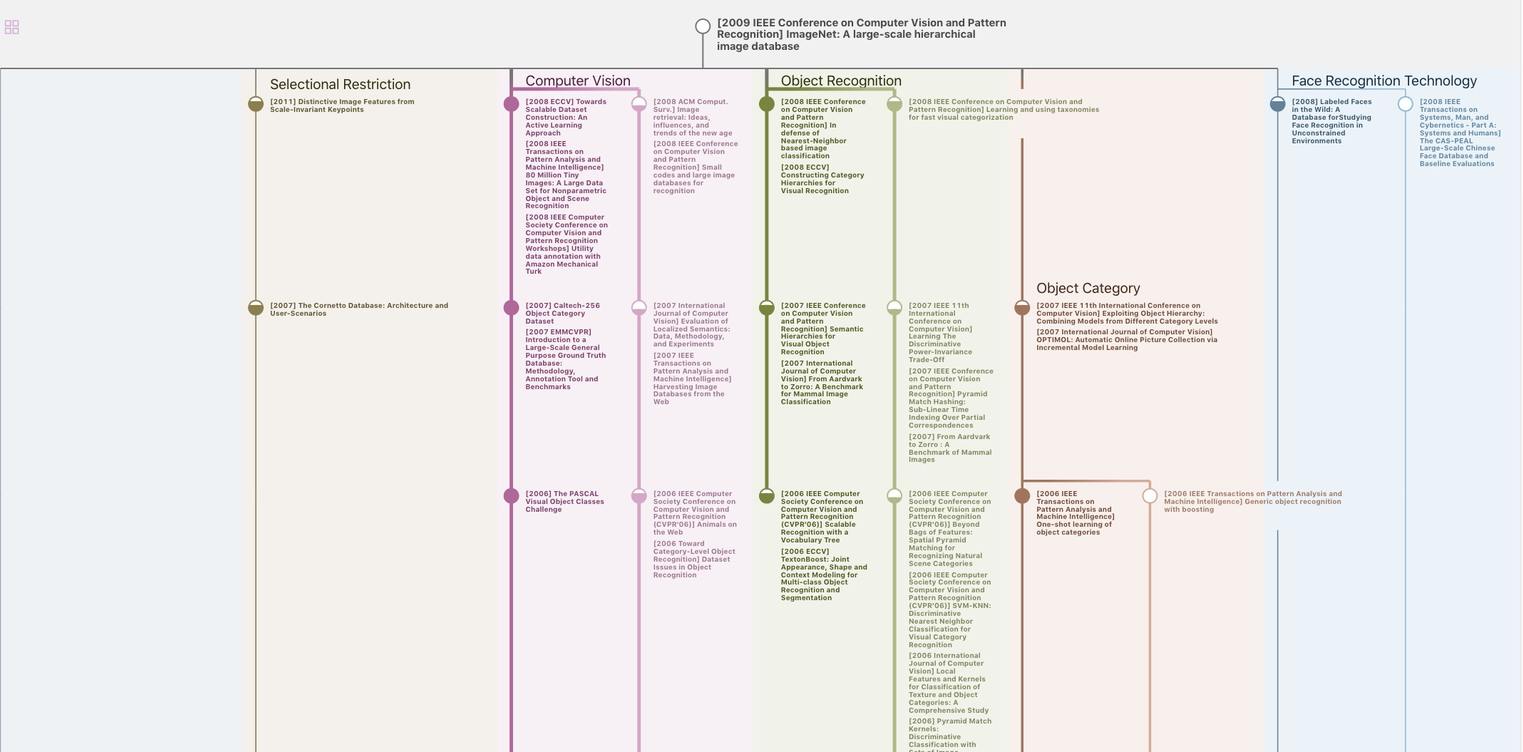
生成溯源树,研究论文发展脉络
Chat Paper
正在生成论文摘要