Beyond NaN: Resiliency of Optimization Layers in The Face of Infeasibility
arxiv(2023)
摘要
Prior work has successfully incorporated optimization layers as the last layer in neural networks for various problems, thereby allowing joint learning and planning in one neural network forward pass. In this work, we identify a weakness in such a set-up where inputs to the optimization layer lead to undefined output of the neural network. Such undefined decision outputs can lead to possible catastrophic outcomes in critical real time applications. We show that an adversary can cause such failures by forcing rank deficiency on the matrix fed to the optimization layer which results in the optimization failing to produce a solution. We provide a defense for the failure cases by controlling the condition number of the input matrix. We study the problem in the settings of synthetic data, Jigsaw Sudoku, and in speed planning for autonomous driving, building on top of prior frameworks in end-to-end learning and optimization. We show that our proposed defense effectively prevents the framework from failing with undefined output. Finally, we surface a number of edge cases which lead to serious bugs in popular equation and optimization solvers which can be abused as well.
更多查看译文
关键词
optimization layers,infeasibility,resiliency
AI 理解论文
溯源树
样例
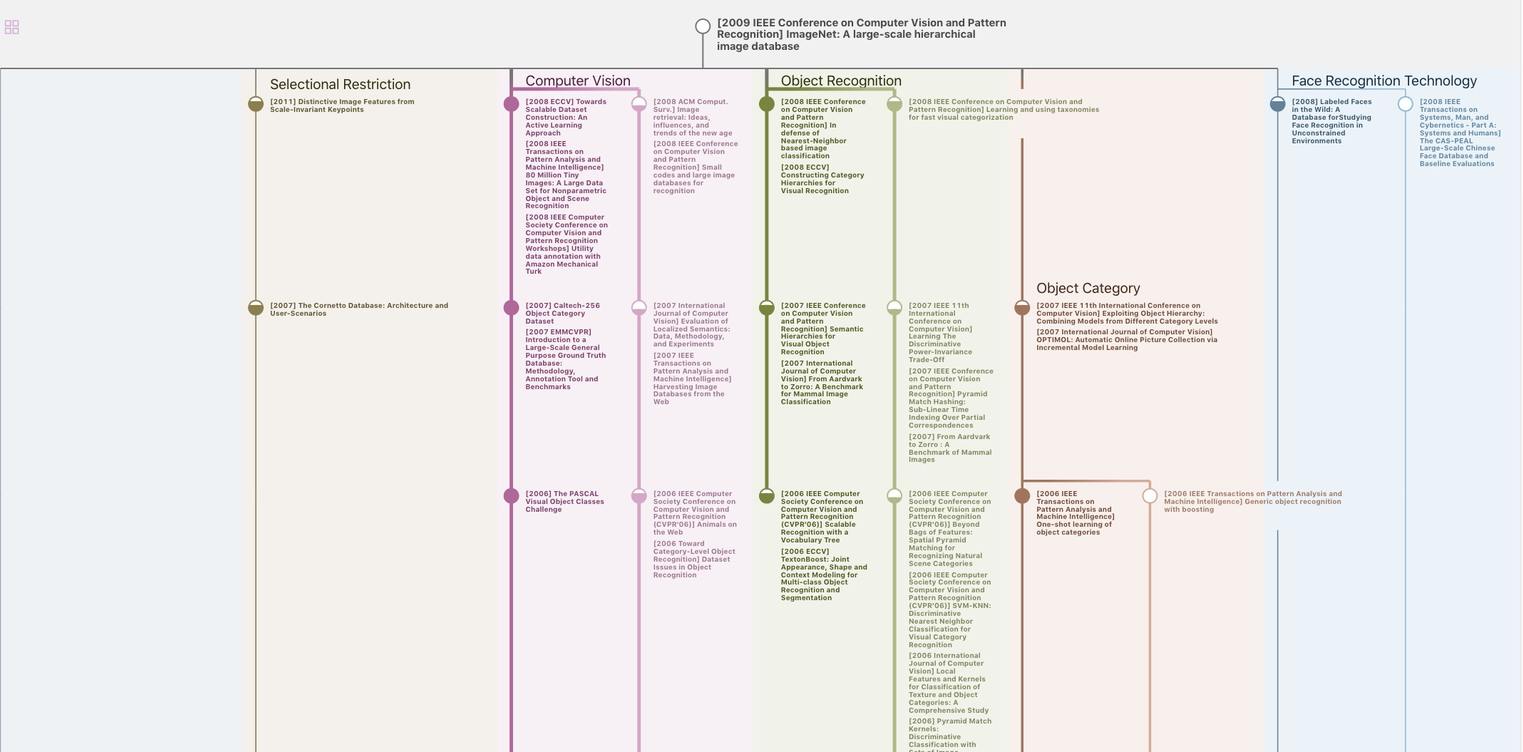
生成溯源树,研究论文发展脉络
Chat Paper
正在生成论文摘要