Measurably Stronger Explanation Reliability Via Model Canonization
arxiv(2022)
摘要
While rule-based attribution methods have proven useful for providing local explanations for Deep Neural Networks, explaining modern and more varied network architectures yields new challenges in generating trustworthy explanations, since the established rule sets might not be sufficient or applicable to novel network structures. As an elegant solution to the above issue, network canonization has recently been introduced. This procedure leverages the implementation-dependency of rule-based attributions and restructures a model into a functionally identical equivalent of alternative design to which established attribution rules can be applied. However, the idea of canonization and its usefulness have so far only been explored qualitatively. In this work, we quantitatively verify the beneficial effects of network canonization to rule-based attributions on VGG-16 and ResNet18 models with BatchNorm layers and thus extend the current best practices for obtaining reliable neural network explanations.
更多查看译文
关键词
BatchNorm layers,deep neural networks,established attribution rules,established rule sets,functionally identical equivalent,measurably stronger explanation reliability,modern network architectures,network canonization,reliable neural network explanations,ResNet18 models,rule-based attributions,trustworthy explanations,VGG-16 models
AI 理解论文
溯源树
样例
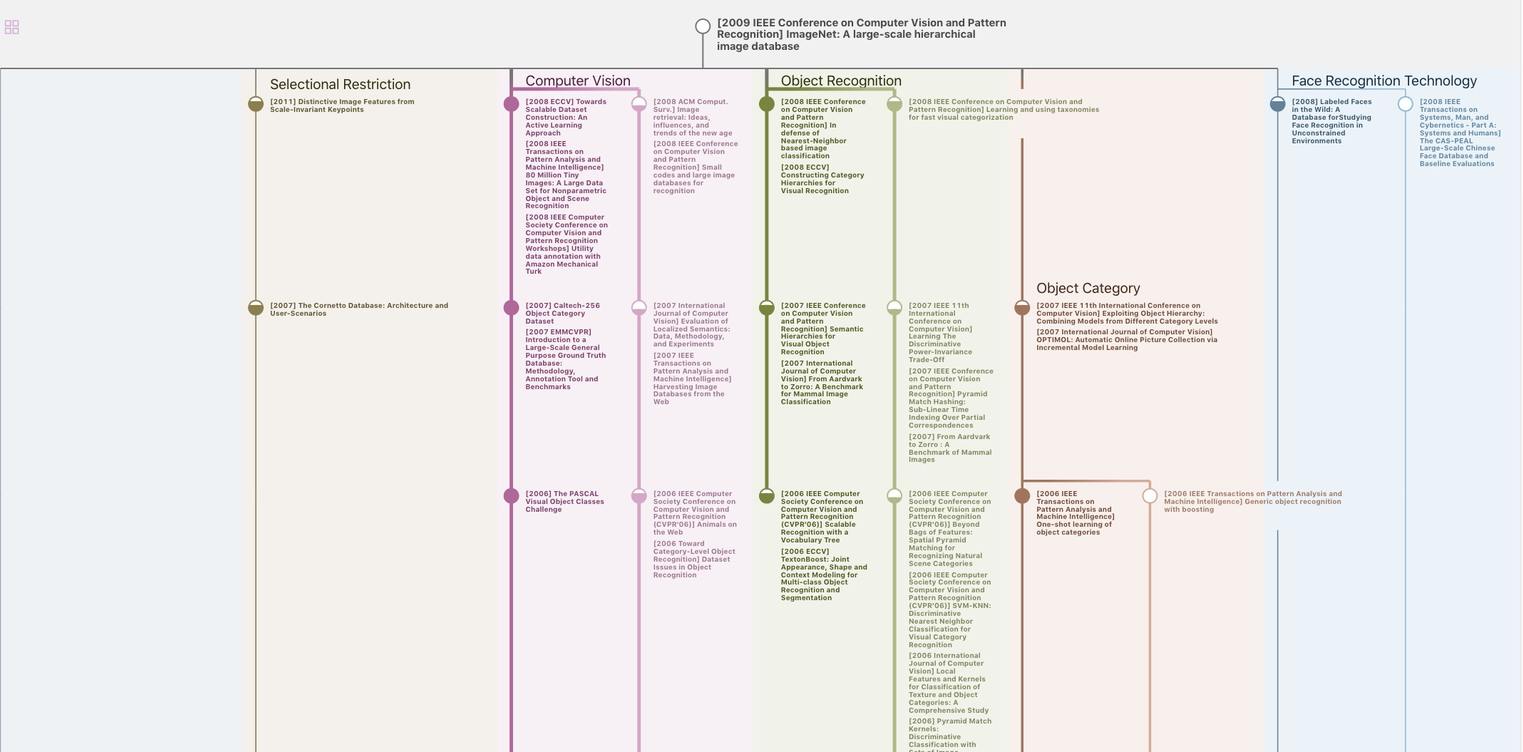
生成溯源树,研究论文发展脉络
Chat Paper
正在生成论文摘要