On the Complexity of Object Detection on Real-world Public Transportation Images for Social Distancing Measurement
IEEE International Joint Conference on Neural Network (IJCNN)(2022)
摘要
Social distancing in public spaces has become an essential aspect in helping to reduce the impact of the COVID-19 pandemic. Exploiting recent advances in machine learning, there have been many studies in the literature implementing social distancing via object detection through the use of surveillance cameras in public spaces. However, to date, there has been no study of social distance measurement on public transport. The public transport setting has some unique challenges, including some low-resolution images and camera locations that can lead to the partial occlusion of passengers, which make it challenging to perform accurate detection. Thus, in this paper, we investigate the challenges of performing accurate social distance measurement on public transportation. We benchmark several state-of-the-art object detection algorithms using real-world footage taken from the London Underground and bus network. The work highlights the complexity of performing social distancing measurement on images from current public transportation onboard cameras. Further, exploiting domain knowledge of expected passenger behaviour, we attempt to improve the quality of the detections using various strategies and show improvement over using vanilla object detection alone.
更多查看译文
关键词
real-world public transportation images,social distancing measurement,public spaces,public transport setting,object detection algorithms,vanilla object detection,public transportation onboard cameras,COVID-19 pandemic,machine learning,low-resolution images,physical camera locations,London underground network,bus network
AI 理解论文
溯源树
样例
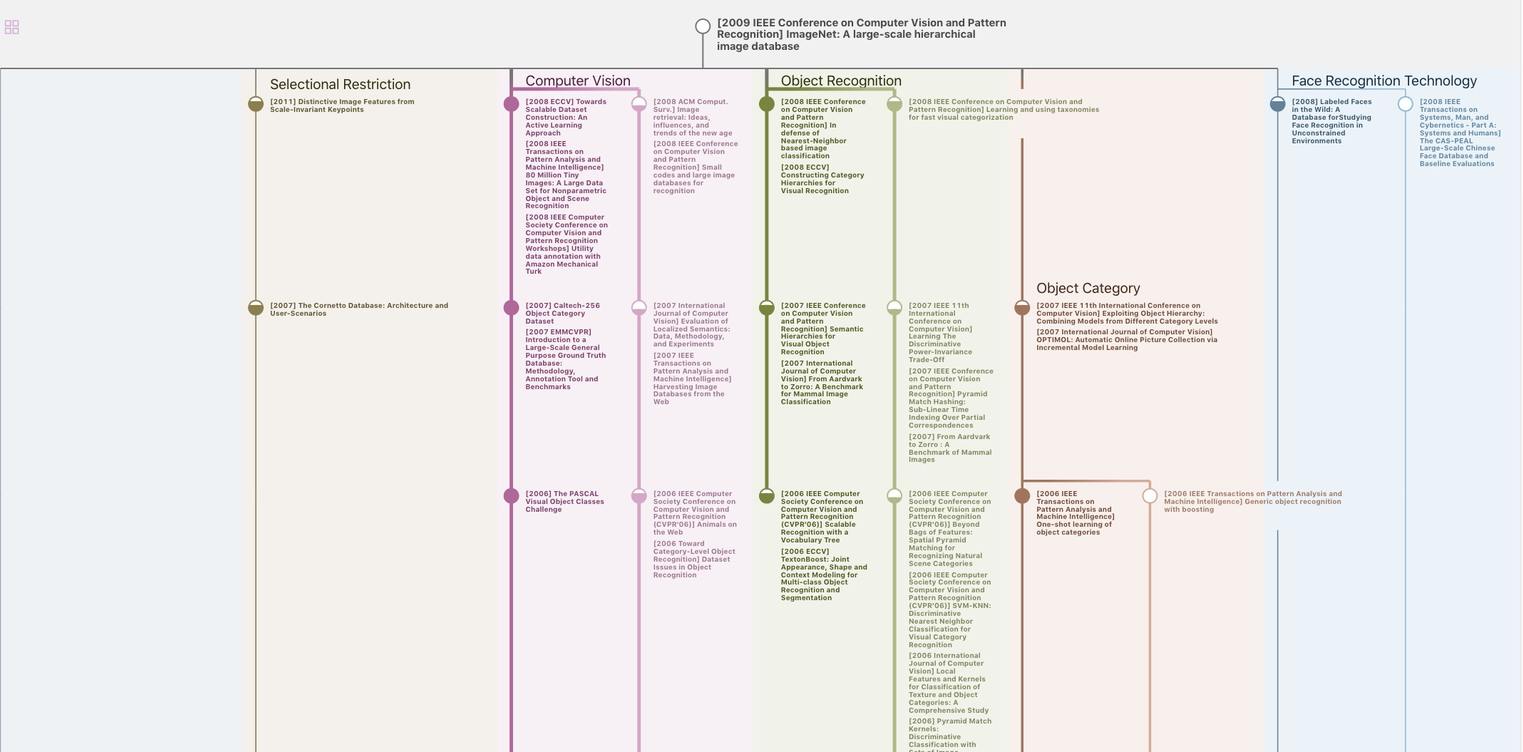
生成溯源树,研究论文发展脉络
Chat Paper
正在生成论文摘要