Convex Programs and Lyapunov Functions for Reinforcement Learning: A Unified Perspective on the Analysis of Value-Based Methods.
ACC(2022)
摘要
Value-based methods play a fundamental role in Markov decision processes (MDPs) and reinforcement learning (RL). In this paper, we present a unified control-theoretic framework for analyzing valued-based methods such as value computation (VC), value iteration (VI), and temporal difference (TD) learning (with linear function approximation). Built upon an intrinsic connection between value-based methods and dynamic systems, we can directly use existing convex testing conditions in control theory to derive various convergence results for the aforementioned value-based methods. These testing conditions are convex programs in form of either linear programming (LP) or semidefinite programming (SDP), and can be solved to construct Lyapunov functions in a straightforward manner. Our analysis reveals some intriguing connections between feedback control systems and RL algorithms. It is our hope that such connections can inspire more work at the intersection of system/control theory and RL.
更多查看译文
关键词
convex programs,Lyapunov functions,reinforcement learning,valued-based methods,value iteration,semidefinite programming,Markov decision processes,MDPs,value computation,control theory,temporal difference learning,linear function approximation,dynamic systems,convex testing,linear programming,SDP,feedback control systems,RL algorithms
AI 理解论文
溯源树
样例
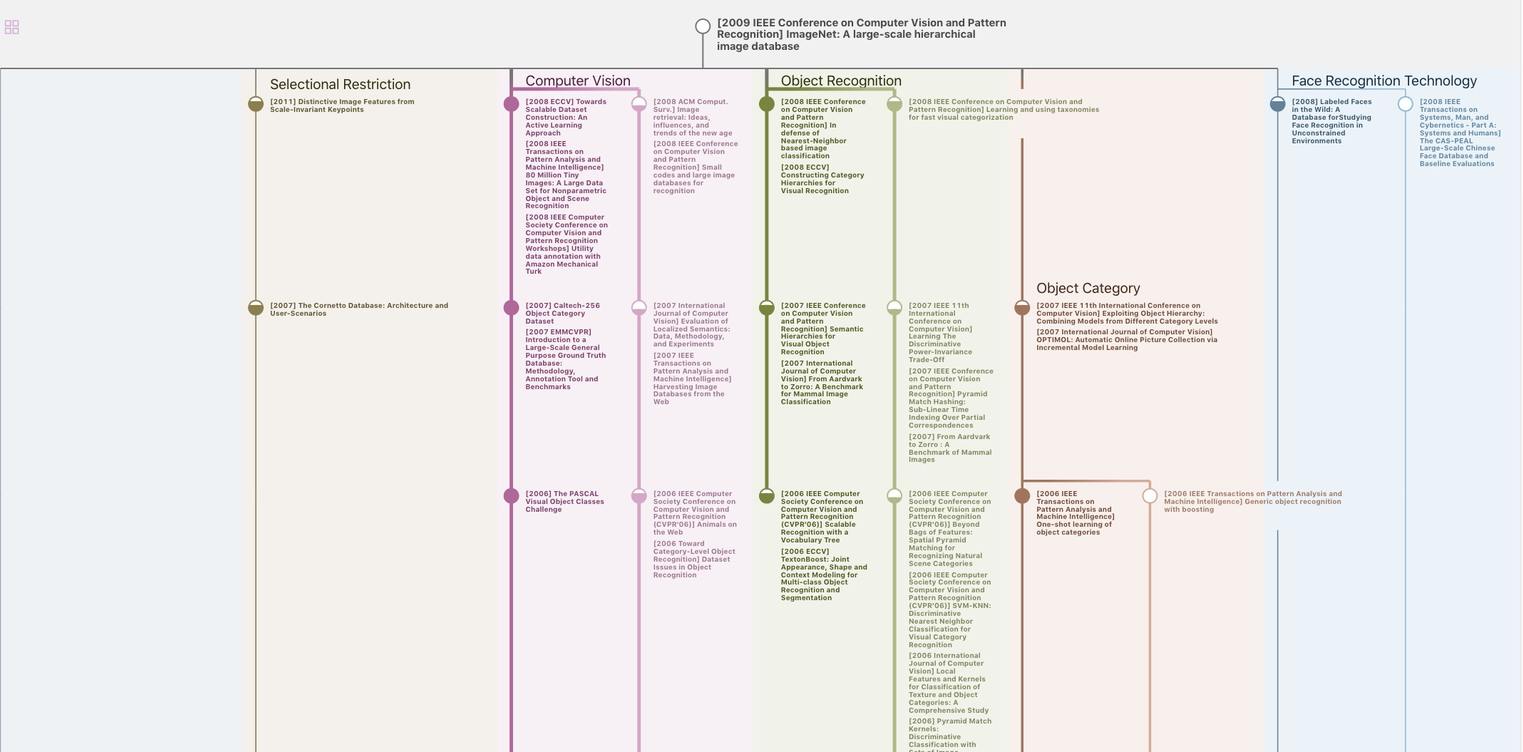
生成溯源树,研究论文发展脉络
Chat Paper
正在生成论文摘要