Privacy-preserving estimation of an optimal individualized treatment rule: a case study in maximizing time to severe depression-related outcomes
Lifetime Data Analysis(2022)
摘要
Estimating individualized treatment rules—particularly in the context of right-censored outcomes—is challenging because the treatment effect heterogeneity of interest is often small, thus difficult to detect. While this motivates the use of very large datasets such as those from multiple health systems or centres, data privacy may be of concern with participating data centres reluctant to share individual-level data. In this case study on the treatment of depression, we demonstrate an application of distributed regression for privacy protection used in combination with dynamic weighted survival modelling (DWSurv) to estimate an optimal individualized treatment rule whilst obscuring individual-level data. In simulations, we demonstrate the flexibility of this approach to address local treatment practices that may affect confounding, and show that DWSurv retains its double robustness even when performed through a (weighted) distributed regression approach. The work is motivated by, and illustrated with, an analysis of treatment for unipolar depression using the United Kingdom’s Clinical Practice Research Datalink.
更多查看译文
关键词
Data aggregation,Distributed regression,Dynamic weighted survival modelling,Effect modification,Precision medicine,Selective serotonin reuptake inhibitors
AI 理解论文
溯源树
样例
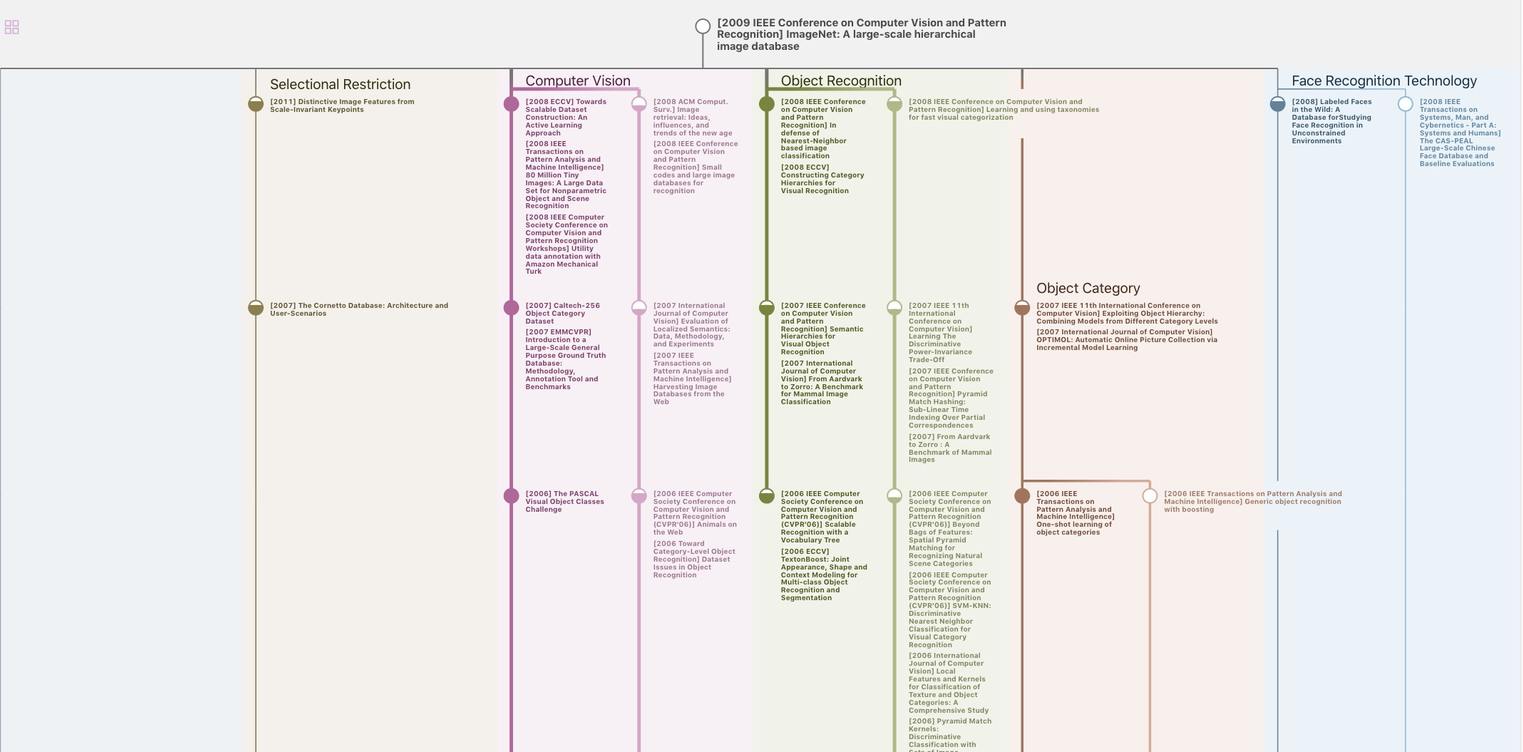
生成溯源树,研究论文发展脉络
Chat Paper
正在生成论文摘要