Bayesian stochastic gradient descent for stochastic optimization with streaming input data
SIAM JOURNAL ON OPTIMIZATION(2024)
摘要
We consider stochastic optimization under distributional uncertainty, where the unknown distributional parameter is estimated from streaming data that arrive sequentially over time. Moreover, data may depend on the decision at the time when they are generated. For both decision -independent and decision -dependent uncertainties, we propose an approach to jointly estimate the distributional parameter via Bayesian posterior distribution and update the decision by applying stochastic gradient descent (SGD) on the Bayesian average of the objective function. Our approach converges asymptotically over time and achieves the convergence rates of classical SGD in the decision -independent case. We demonstrate the empirical performance of our approach on both synthetic test problems and a classical newsvendor problem.
更多查看译文
关键词
Key words. Bayesian estimation,streaming input data,stochastic gradient descent,endogenous uncertainty
AI 理解论文
溯源树
样例
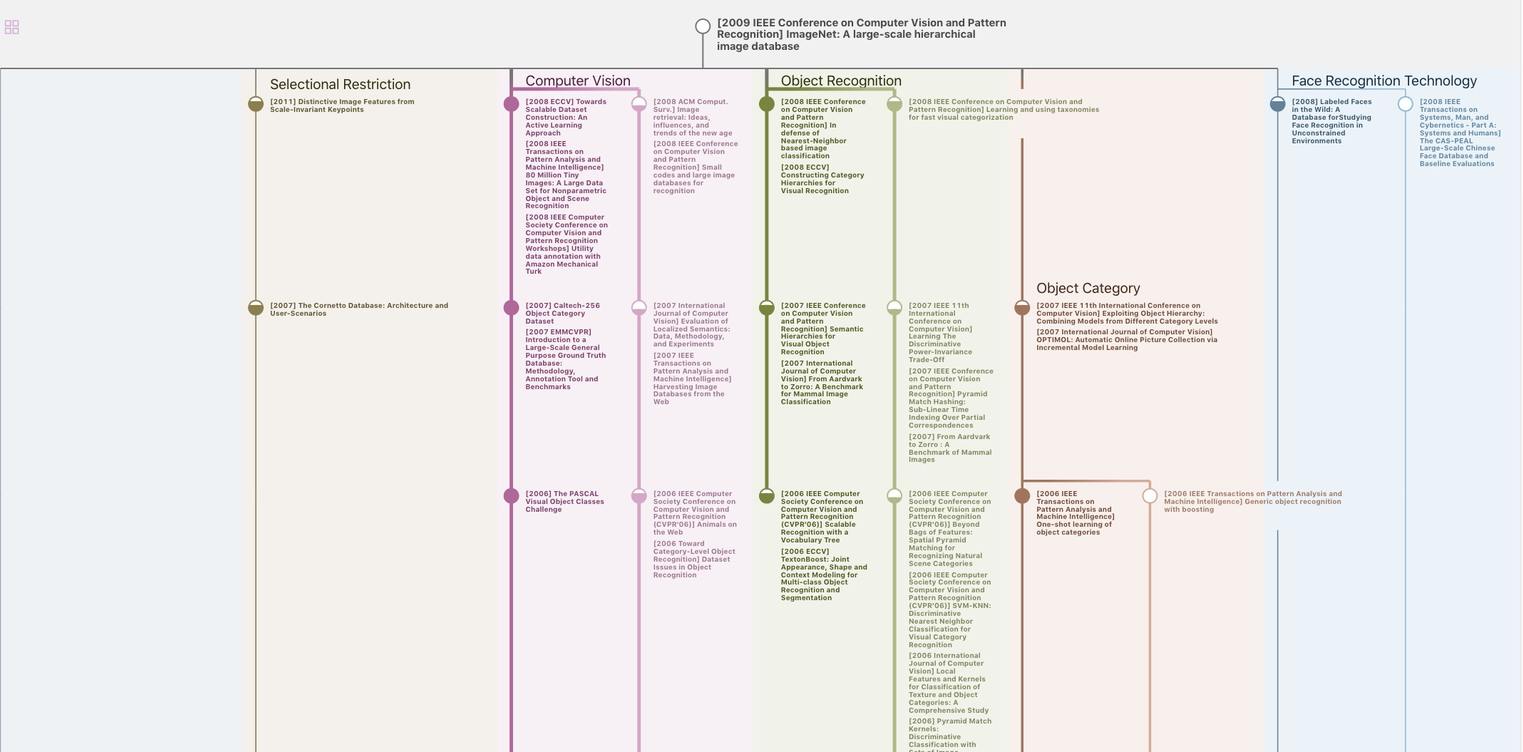
生成溯源树,研究论文发展脉络
Chat Paper
正在生成论文摘要