Enhancing Crop Yield Prediction Utilizing Machine Learning on Satellite-Based Vegetation Health Indices
SENSORS(2022)
摘要
Accurate crop yield forecasting is essential in the food industry's decision-making process, where vegetation condition index (VCI) and thermal condition index (TCI) coupled with machine learning (ML) algorithms play crucial roles. The drawback, however, is that a one-fits-all prediction model is often employed over an entire region without considering subregional VCI and TCI's spatial variability resulting from environmental and climatic factors. Furthermore, when using nonlinear ML, redundant VCI/TCI data present additional challenges that adversely affect the models' output. This study proposes a framework that (i) employs higher-order spatial independent component analysis (sICA), and (ii), exploits a combination of the principal component analysis (PCA) and ML (i.e., PCA-ML combination) to deal with the two challenges in order to enhance crop yield prediction accuracy. The proposed framework consolidates common VCI/TCI spatial variability into their respective subregions, using Vietnam as an example. Compared to the one-fits-all approach, subregional rice yield forecasting models over Vietnam improved by an average level of 20% up to 60%. PCA-ML combination outperformed ML-only by an average of 18.5% up to 45%. The framework generates rice yield predictions 1 to 2 months ahead of the harvest with an average of 5% error, displaying its reliability.
更多查看译文
关键词
crop yield prediction, vegetation condition index (VCI), thermal condition index (TCI), independent component analysis (ICA), principle component analysis (PCA), machine learning
AI 理解论文
溯源树
样例
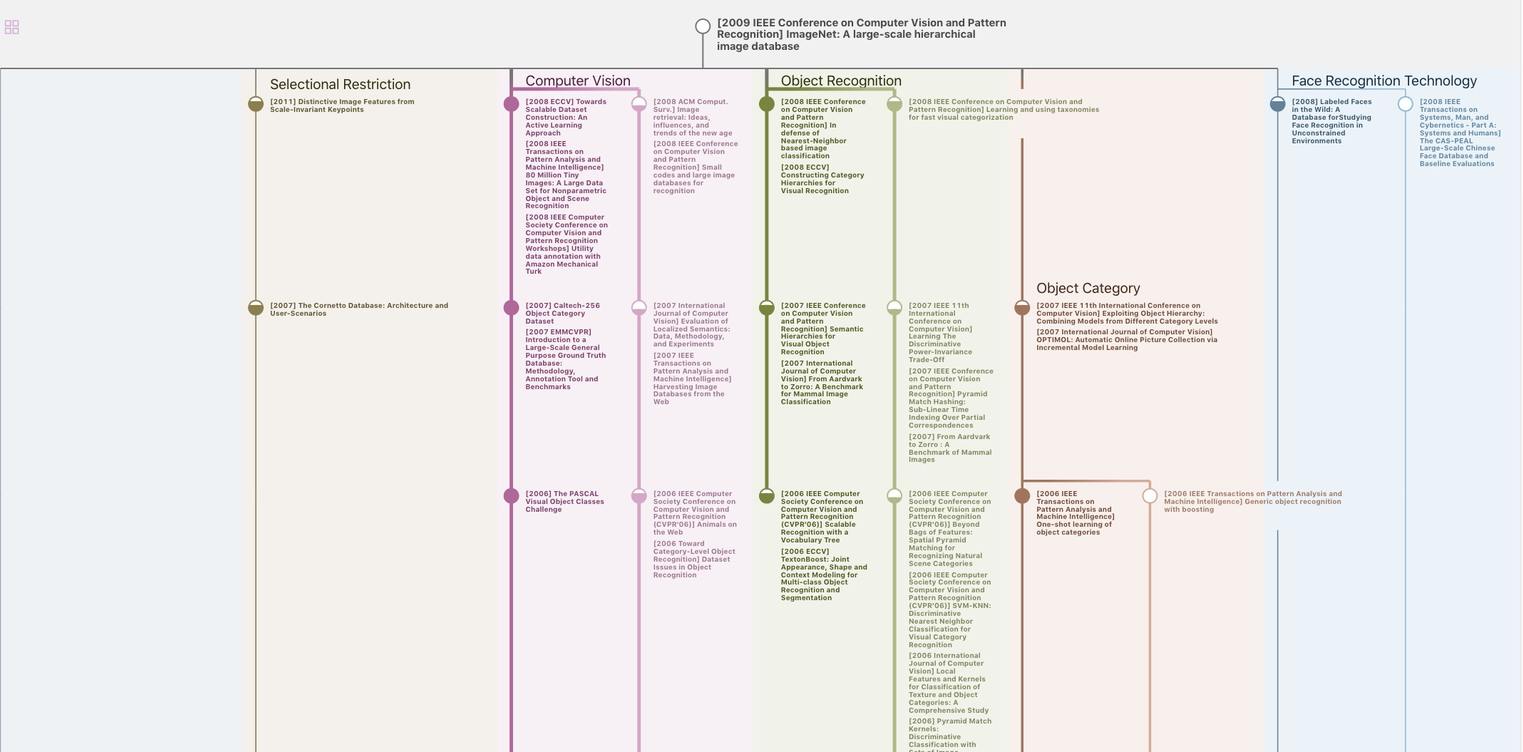
生成溯源树,研究论文发展脉络
Chat Paper
正在生成论文摘要