Differentiable Preisach Modeling for Characterization and Optimization of Particle Accelerator Systems with Hysteresis
Physical Review Letters(2022)
摘要
Future improvements in particle accelerator performance are predicated on increasingly accurate online modeling of accelerators. Hysteresis effects in magnetic, mechanical, and material components of accelerators are often neglected in online accelerator models used to inform control algorithms, even though reproducibility errors from systems exhibiting hysteresis are not negligible in high precision accelerators. In this Letter, we combine the classical Preisach model of hysteresis with machine learning techniques to efficiently create nonparametric, high-fidelity models of arbitrary systems exhibiting hysteresis. We experimentally demonstrate how these methods can be used in situ, where a hysteresis model of an accelerator magnet is combined with a Bayesian statistical model of the beam response, allowing characterization of magnetic hysteresis solely from beam-based measurements. Finally, we explore how using these joint hysteresis-Bayesian statistical models allows us to overcome optimization performance limitations that arise when hysteresis effects are ignored.
更多查看译文
关键词
Hysteresis Model,Motor Design Optimization,High-Speed Applications
AI 理解论文
溯源树
样例
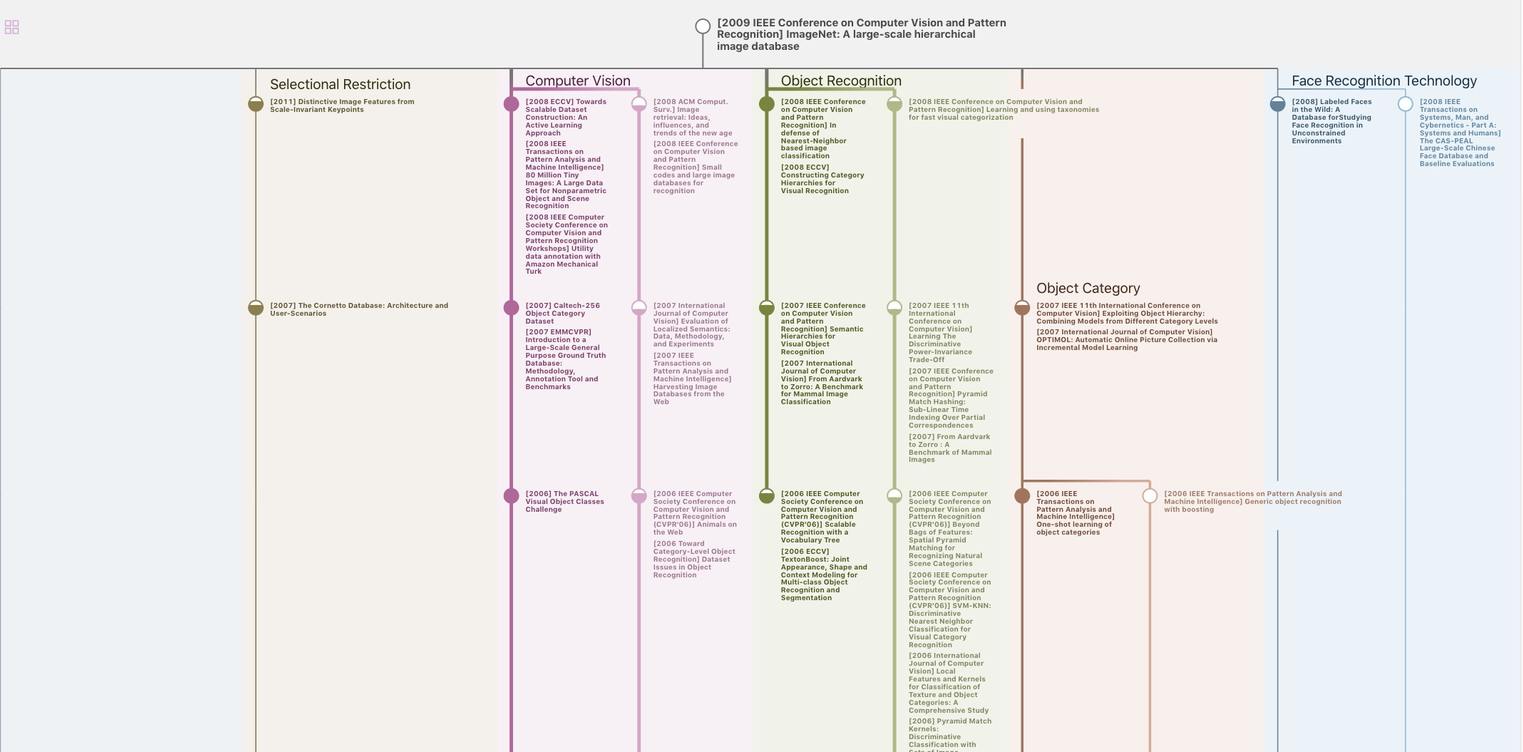
生成溯源树,研究论文发展脉络
Chat Paper
正在生成论文摘要