The efficacy and generalizability of conditional gans for posterior inference in physics-based inverse problems
NUMERICAL ALGEBRA CONTROL AND OPTIMIZATION(2024)
摘要
. In this work, we train conditional Wasserstein generative adversarial networks to effectively sample from the posterior of physics-based Bayesian inference problems. The generator is constructed using a U-Net architecture, with the latent information injected using conditional instance normalization. The former facilitates a multiscale inverse map, while the latter enables the decoupling of the latent space dimension from the dimension of the measurement, and introduces stochasticity at all scales of the U-Net. We solve PDE-based inverse problems to demonstrate the performance of our approach in quantifying the uncertainty in the inferred field. Further, we show the generator can learn inverse maps which are local in nature, which in turn promotes generalizability when testing with out-of-distribution samples.
更多查看译文
关键词
Bayesian inference,generative adversarial networks,out <span style="color,#c71585">of distribution generalization,PDE-based inverse problems,uncertainty quantification
AI 理解论文
溯源树
样例
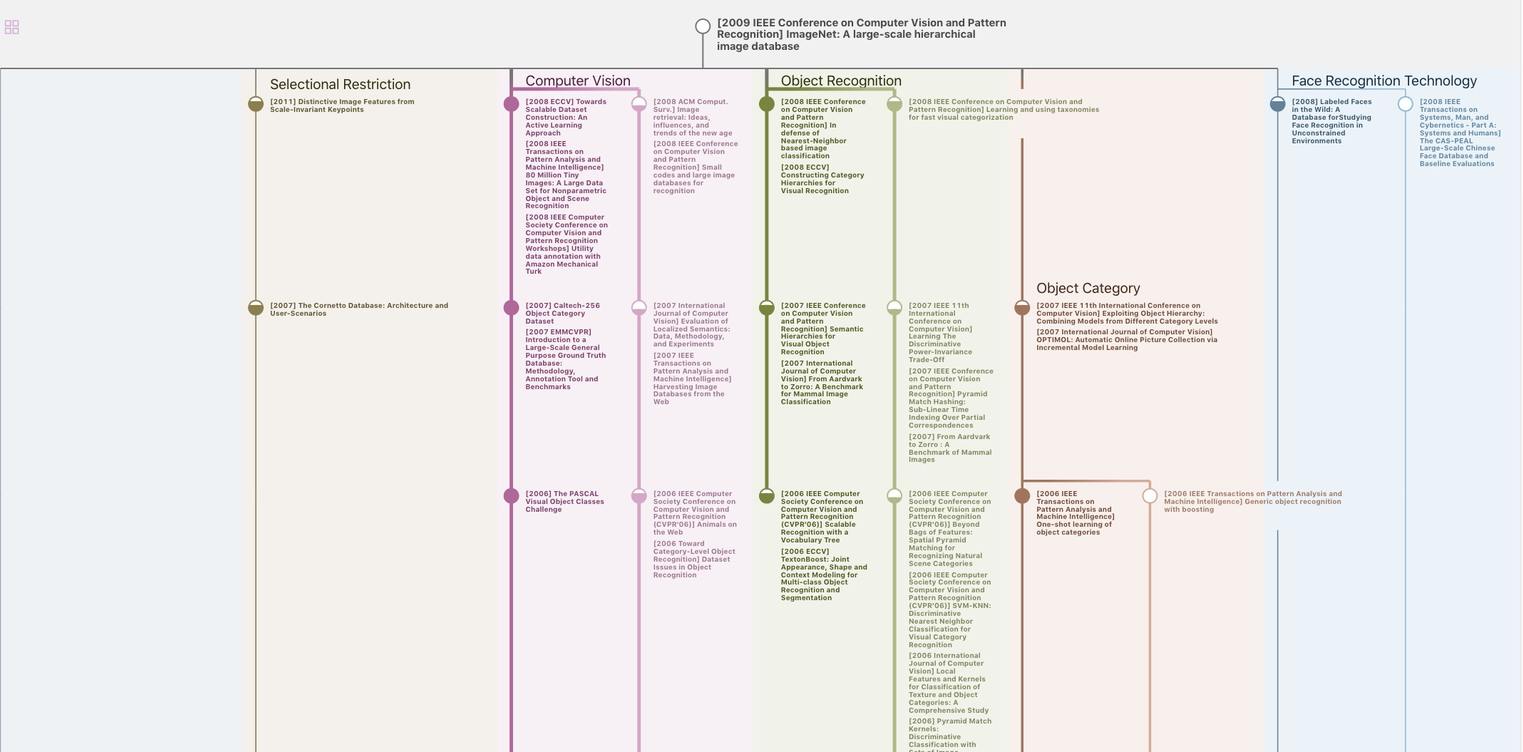
生成溯源树,研究论文发展脉络
Chat Paper
正在生成论文摘要