Analysis of random sequential message passing algorithms for approximate inference
JOURNAL OF STATISTICAL MECHANICS-THEORY AND EXPERIMENT(2022)
Abstract
We analyze the dynamics of a random sequential message passing algorithm for approximate inference with large Gaussian latent variable models in a student-teacher scenario. To model nontrivial dependencies between the latent variables, we assume random covariance matrices drawn from rotation invariant ensembles. Moreover, we consider a model mismatching setting, where the teacher model and the one used by the student may be different. By means of dynamical functional approach, we obtain exact dynamical mean-field equations characterizing the dynamics of the inference algorithm. We also derive a range of model parameters for which the sequential algorithm does not converge. The boundary of this parameter range coincides with the de Almeida Thouless (AT) stability condition of the replica-symmetric ansatz for the static probabilistic model.
MoreTranslated text
Key words
analysis of algorithms, machine learning, message-passing algorithms, statistical inference
AI Read Science
Must-Reading Tree
Example
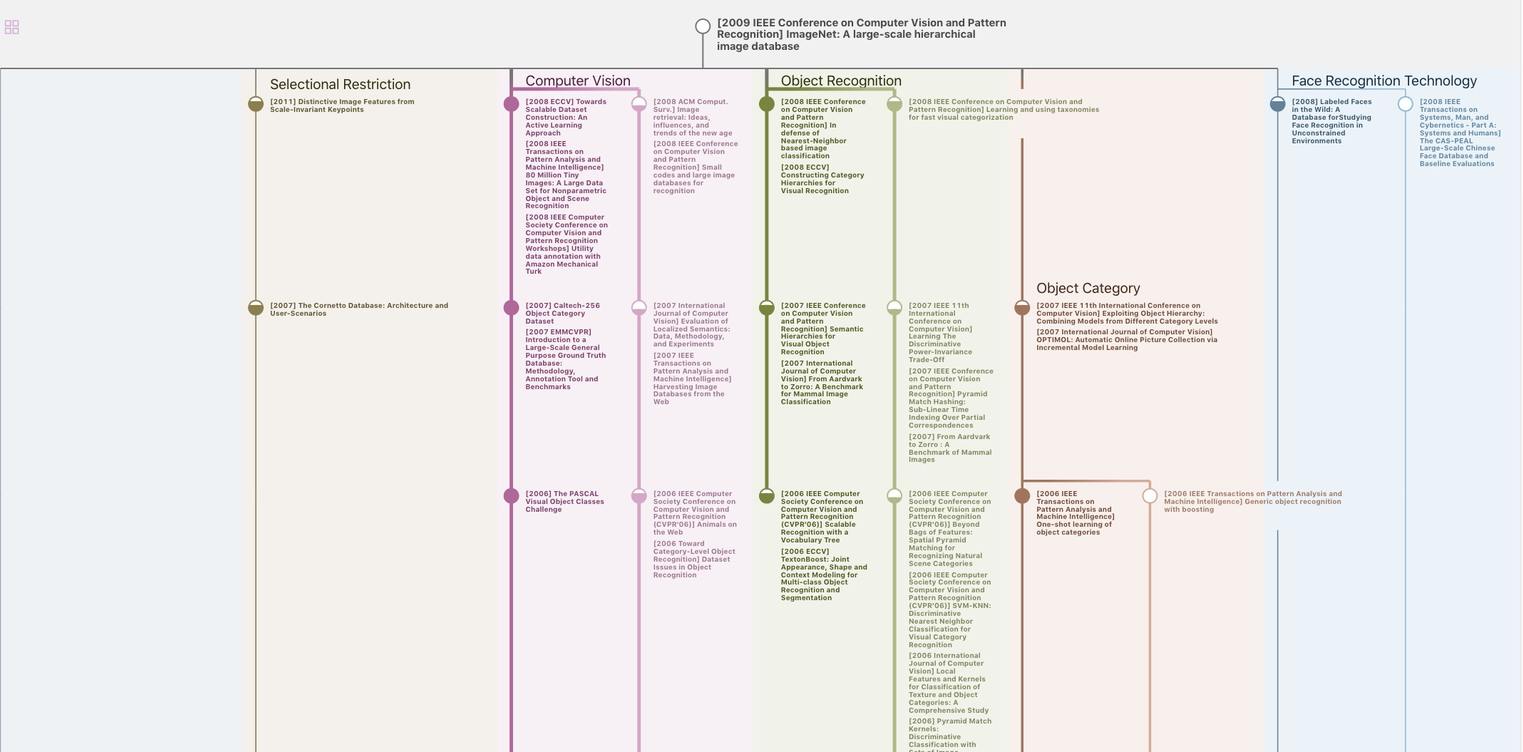
Generate MRT to find the research sequence of this paper
Chat Paper
Summary is being generated by the instructions you defined