Adaptive Dual-View WaveNet for urban spatial–temporal event prediction
Information Sciences(2022)
摘要
Spatial–temporal event prediction is a particular task for multivariate time series forecasting. Therefore, the complex entangled dynamics of space and time need to be considered. This task is an essential but crucial loop in future smart cities construction, which can be widely applied in urban traffic management, disaster monitoring and mobility analysis. In recent years, video-like spatial–temporal modelling has been the most common approach in many deep learning models. However, the video-like modelling approach cannot consider some latent region-wise correlations other than geographic spatial distance information. To overcome the limitation, we propose a novel neural network framework, Adaptive Dual-View WaveNet (ADVW-Net), for the urban spatial–temporal event prediction. By integrating the spatial representations from Convolutional Neural Network (CNN) and that from adaptive Graph convolutional neural network (GCN), our proposed model can capture not only the geographic correlations but also some latent region-wise dependencies from the input data. In addition, the effective architecture, WaveNet, can be transferred to region-wise spatial–temporal prediction scenarios for long-range temporal dependencies learning. Experimental results on three urban datasets demonstrate the superior performance of our proposed model.
更多查看译文
关键词
Spatial–temporal prediction,Representation learning,WaveNet,Graph convolutional neural network
AI 理解论文
溯源树
样例
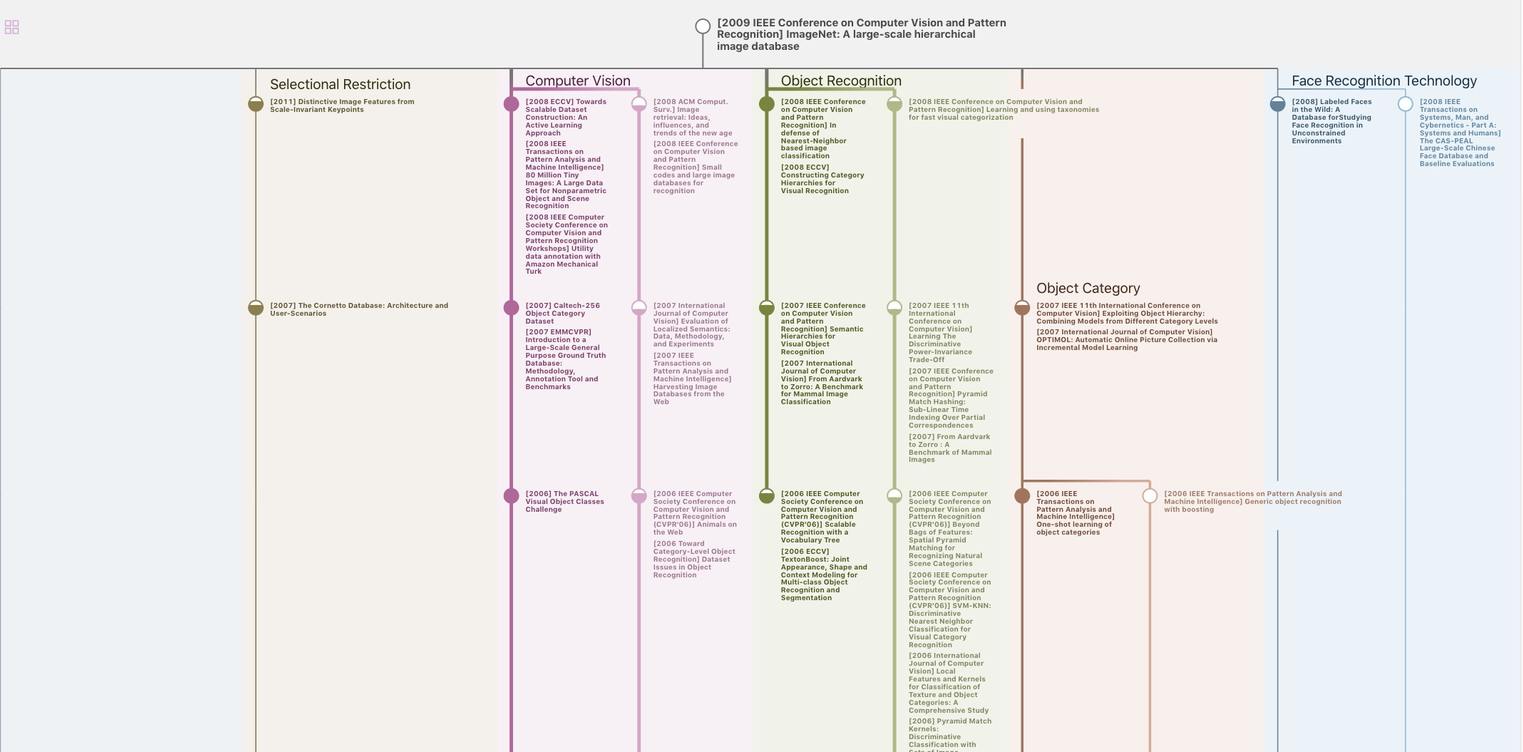
生成溯源树,研究论文发展脉络
Chat Paper
正在生成论文摘要