Efficient inference models for classification problems with a high number of fuzzy rules
APPLIED SOFT COMPUTING(2022)
摘要
In data science there are problems that are not visible until you work with a sufficiently large number of data. This is the case, for example, with the design of the inference engine in fuzzy rule-based classification systems. The most common way to implement the winning rule inference method is to use sequential processing that reviews each of the rules in the rule set, to determine the best one and return the associated class. This implementation produces fast response times when the set of rules is small and is applied to a small set of examples. In this paper we explore new versions to implement this inference method, avoiding analyzing all the rules and focusing the analysis on the neighborhood of rules around the example. We study experimentally the conditions where each of them should be applied. Finally, we propose an implementation that combines all the studied versions offering good accuracy results and a significant reduction in the response time. (C) 2021 Elsevier B.V. All rights reserved.
更多查看译文
关键词
Big data, Fuzzy rule-based classification systems, Inference engine, Fuzzy reasoning, Soft computing, Explainable AI
AI 理解论文
溯源树
样例
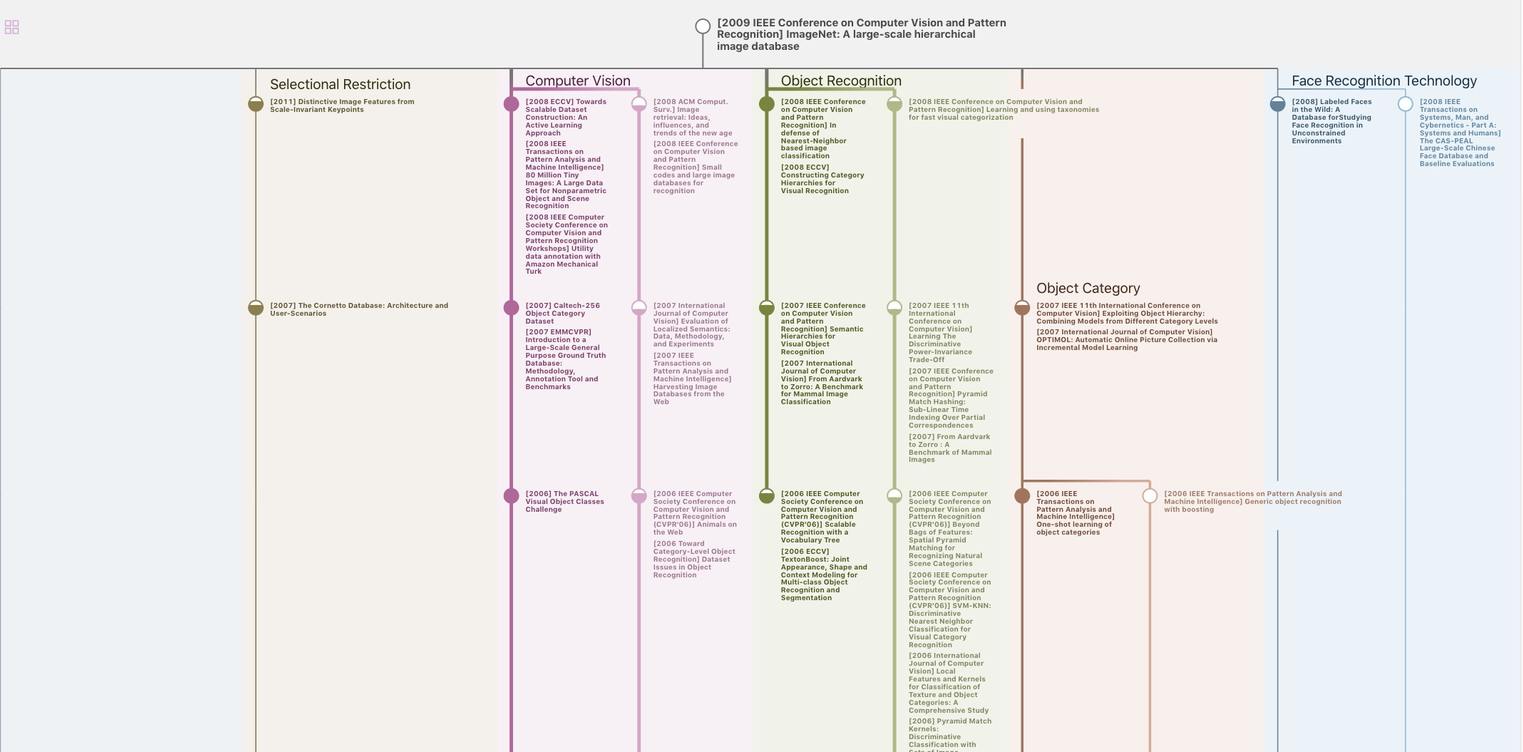
生成溯源树,研究论文发展脉络
Chat Paper
正在生成论文摘要