Classification of cervical cells leveraging simultaneous super-resolution and ordinal regression
APPLIED SOFT COMPUTING(2022)
摘要
Automatic classification of cervical cells plays a critical role in the Computer-assisted Cytology Test (CCT) system. The efficiency of the CCT system can be promoted by sacrificing the microscopic image resolution to speed up the microscopic image acquisition. In this case, the low resolution of the cell image will severely deteriorate the performance of available Convolutional Neural Networks (CNN) based classification methods. Inspired by the positive effect of super-resolution in addressing classification or recognition tasks, we propose a cervical cell classification algorithm leveraging simultaneous superresolution, which is achieved using Generative Adversarial Network (GAN) techniques. Our framework is designed in an end-to-end manner wherein the classification loss is back-propagated into the superresolution network during training. Moreover, we perform ordinal regression with smooth L1 loss to further improve the classification results. Extensive experiments have verified the effectiveness of our method. Our simultaneous super-resolution based method achieves 93.5% classification accuracy on the 6-class Heer dataset, outperforming the method using only the state-of-the-art classifier by an obvious margin of 3.2%. Besides, our ordinal regression method significantly improves the MAE (Mean Absolute Error) by 0.0143 and 1-off accuracy by 0.95% on the 4-class Heer dataset. For the Herlev dataset, our method yields the classification accuracy of 98.1% and 97.6% for the 2-class and 7-class problems, which is still competitive even with low-resolution input. (c) 2021 Elsevier B.V. All rights reserved.
更多查看译文
关键词
Cervical cell classification, Deep-learning, Super-resolution, GAN, Ordinal regression, Smooth L1 loss
AI 理解论文
溯源树
样例
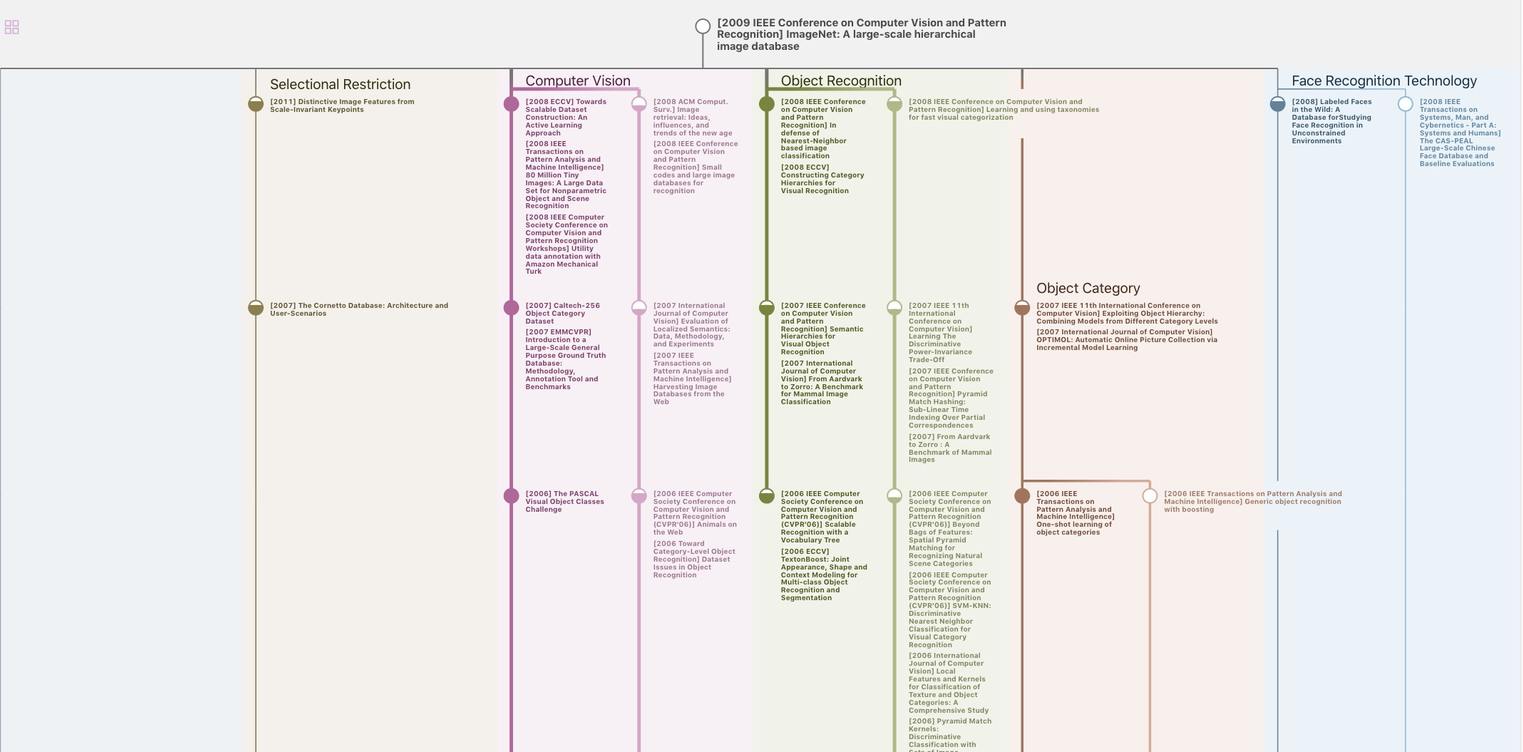
生成溯源树,研究论文发展脉络
Chat Paper
正在生成论文摘要