Detailed Routing Short Violation Prediction Using Graph-Based Deep Learning Model
IEEE Transactions on Circuits and Systems II: Express Briefs(2022)
摘要
As the manufacturing process continuously shrinks, how to accurately estimate routability at placement is becoming increasingly important. In addition to extracting local features, this article innovatively constructs an adjacency matrix to represent the connection relationship among tiles, which can reflect the placement quality more comprehensively. To effectively map local features of tiles to the corresponding adjacency matrix, a graph neural network is employed. This trained model is used to predict short violations at the placement stage. Experimental results demonstrate the proposed method can achieve better binary classification quality for designs with severe shorts and outperforms in inductive learning than available machine learning frameworks.
更多查看译文
关键词
Machine learning,physical design,graph neural network,routability estimation
AI 理解论文
溯源树
样例
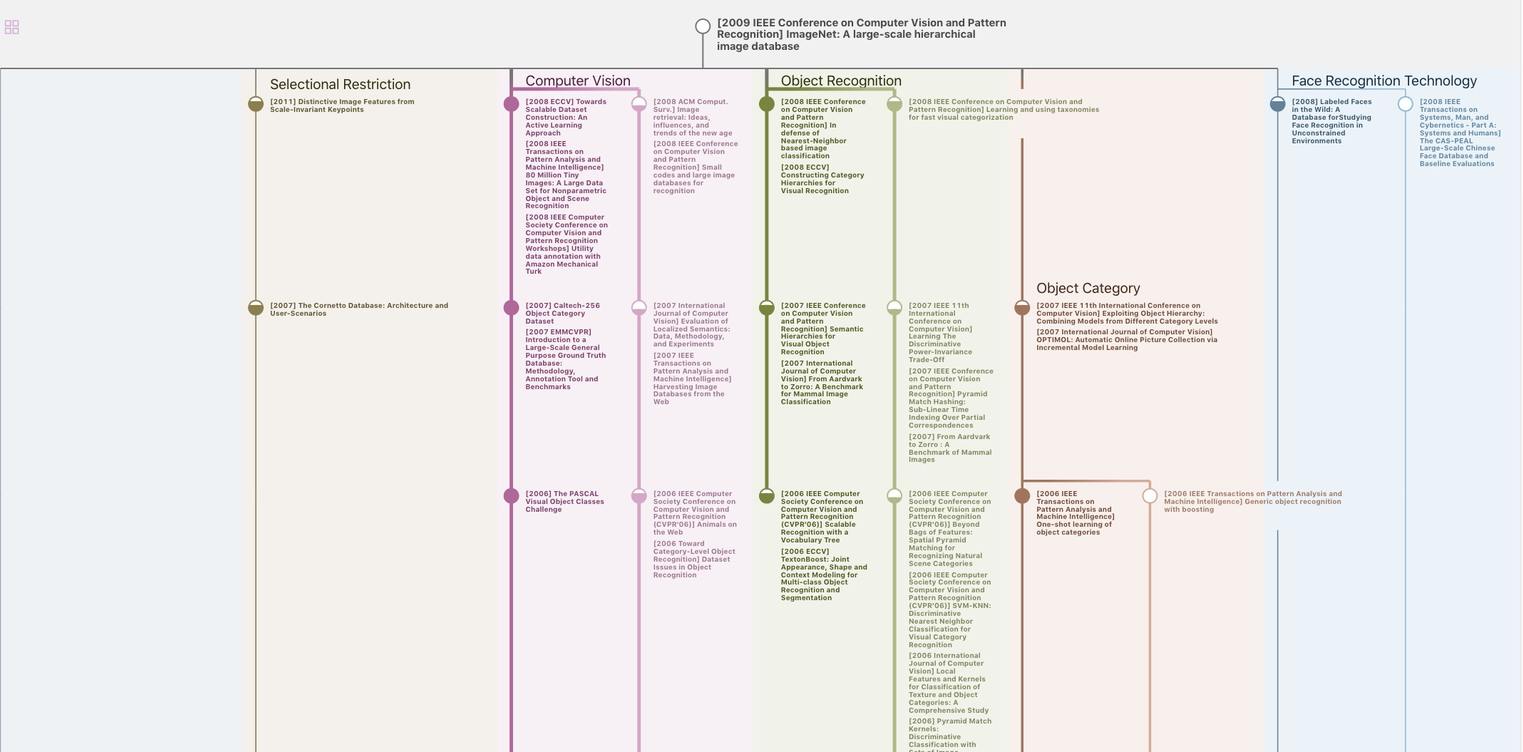
生成溯源树,研究论文发展脉络
Chat Paper
正在生成论文摘要