Few-shot learning based on hierarchical classification via multi-granularity relation networks
International Journal of Approximate Reasoning(2022)
摘要
Few-shot learning is one of the significant areas of machine learning, which aims to recognize novel visual classes from few labeled examples. Many existing models make full use of the similarity of inner-class features and achieve satisfactory results. However, these models assume that classes are independent of each other, ignoring the inter-class relationship. In this paper, we propose a few-shot hierarchical classification model via multi-granularity relation networks (HMRN) considering both the inner-class similarity and inter-class relationship. The multi-granularity relationship among coarse- and fine-grained classes is an important auxiliary information in the class hierarchical structure originated from data. Thus, we first extract hierarchical features of different granularity classes according to the membership relationship among the classes. Second, we build multi-granularity relation networks to obtain the inner-class similarity relation of different granularity classes using the hierarchical features. Finally, we consider the tradeoff among the inner-class similarity relation of different granularity classes for hierarchical few-shot learning, which takes the information of coarse-grained classes to assist the learning of fine-grained classes. Experimental results show that our model outperforms several state-of-the-art flat (without hierarchical structure) models and hierarchical models. For example, the accuracy of HMRN is about 3.00% better than that of flat models on the tieredImageNet dataset.
更多查看译文
关键词
Few-shot learning,Hierarchical classification,Multi-granularity,Relation network
AI 理解论文
溯源树
样例
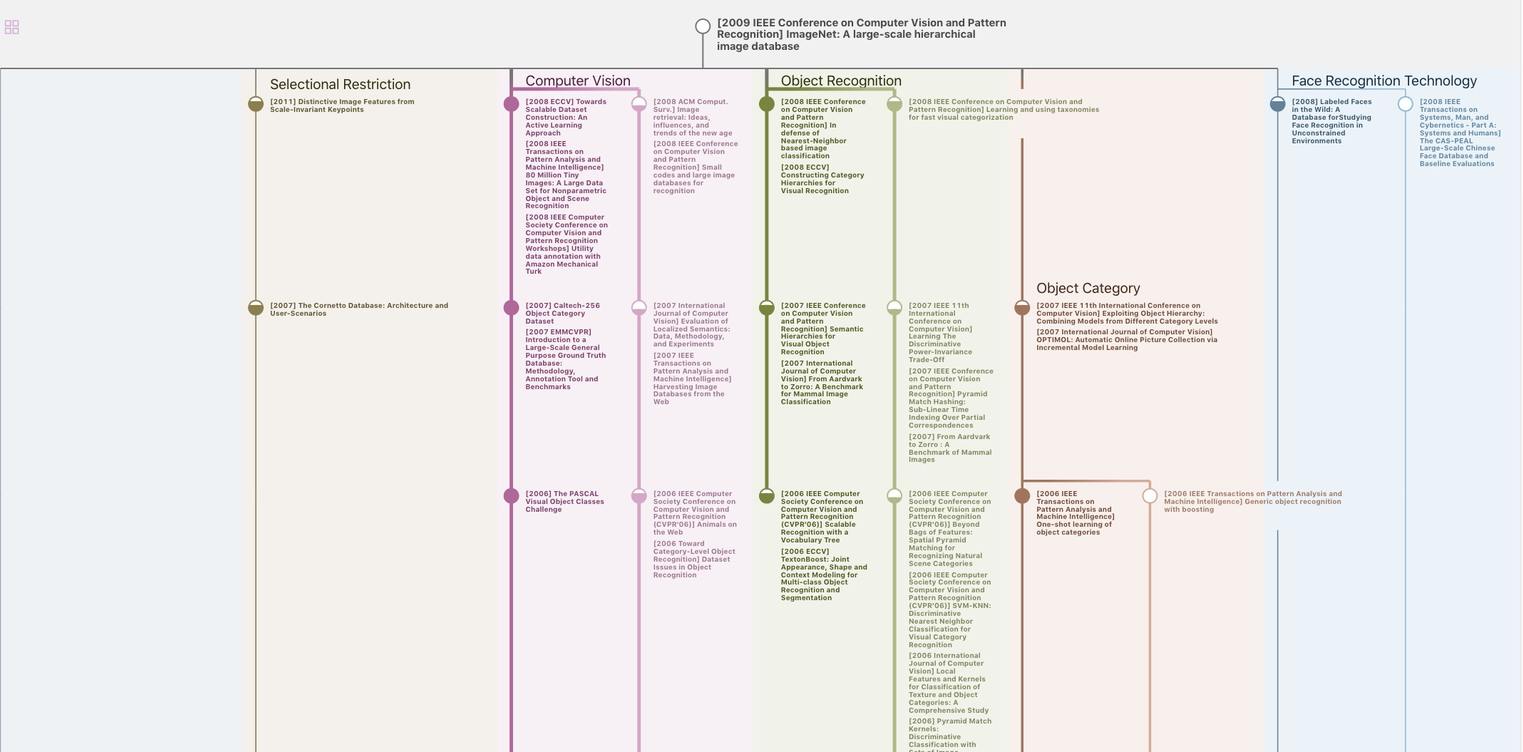
生成溯源树,研究论文发展脉络
Chat Paper
正在生成论文摘要