Hierarchical pyramid attentive network with spatial separable convolution for crowd counting
Engineering Applications of Artificial Intelligence(2022)
摘要
To tackle the challenging scale variation issue of the crowd counting task so as to improve the counting accuracy, we present a novel method based on Hierarchical Pyramid Attentive Network (HPANet) for crowd counting. Specifically, a Scale-aware Pyramid Attentive (SPA) block, extracting the rich multi-scale context, is designed elaborately as using the two-branch spatial separable convolution as its core component to replace the conventional pure convolution with larger kernel size to reduce the computation, as well as adopting a self-attention operation for the spatial feature aggregation. In order to further learn the scale-aware feature representation well from the input image, we stack the designed SPA block in a hierarchical way and fuse their features flexibly as another crucial module of the proposed HPANet, the Hierarchical Feature Fusion (HFF) module. Combining the designed SPA block and HFF module, the developed HPANet could remedy the scale variation issue and thus improve the counting performance with the mighty scale-aware feature representation. The performance of the HPANet is evaluated on four public available benchmark datasets in this paper, including ShanghaiTech, Mall, Beijing BRT and UCF-QNRF. Extensive experimental results on benchmarks demonstrate that the proposed HPANet could have an effective performance for crowd counting and the ablation experimental results validate the effectiveness of the components of HPANet on the counting task. The designed HPANet could realize a preferable counting performance in view of alleviating the scale variation issue, without the cost of introducing too much additional parameters for the multi-column structure.
更多查看译文
关键词
Crowd counting,Scale-aware attentive context,Hierarchical feature fusion,Scale variation
AI 理解论文
溯源树
样例
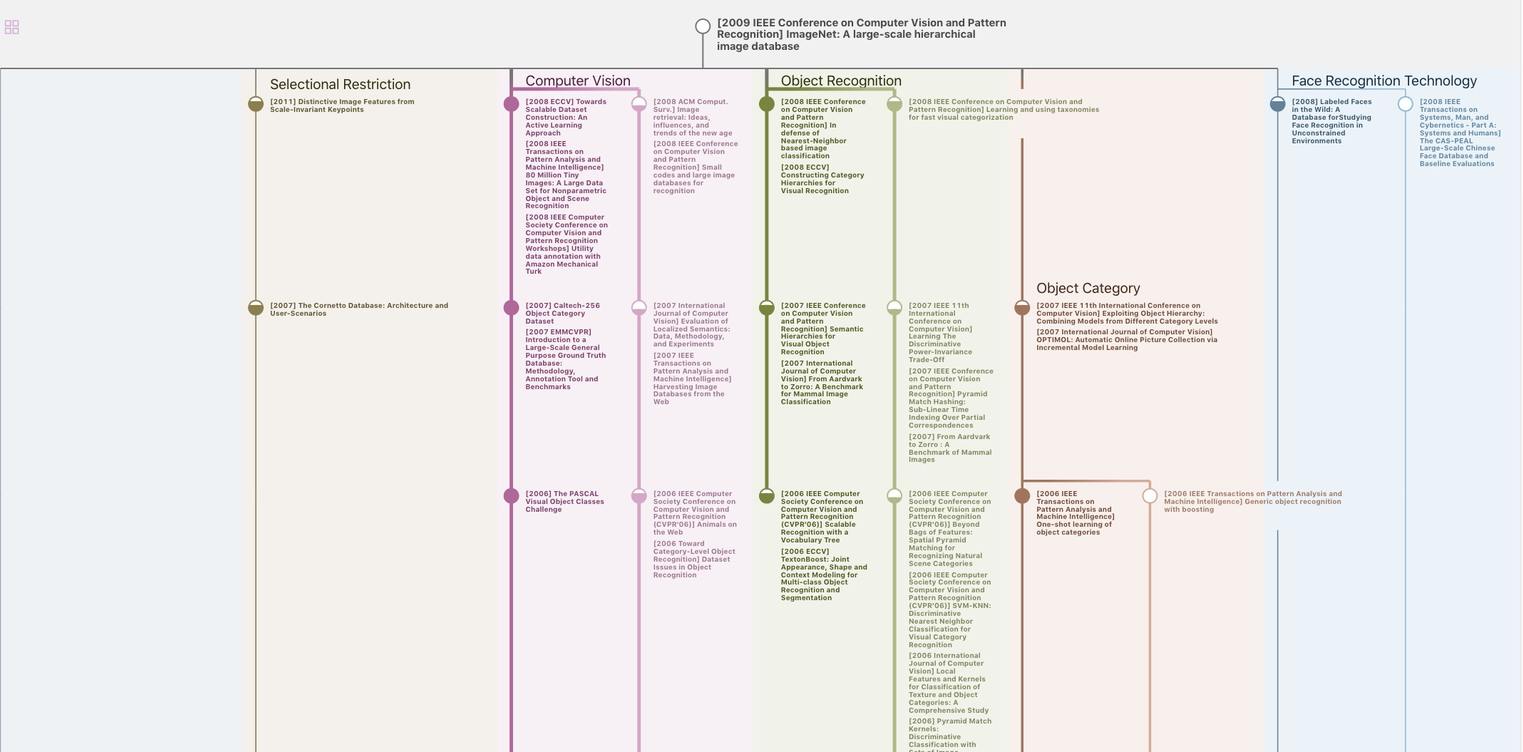
生成溯源树,研究论文发展脉络
Chat Paper
正在生成论文摘要