A novel ensemble learning approach for hourly global solar radiation forecasting
NEURAL COMPUTING & APPLICATIONS(2021)
摘要
Precise solar radiation forecasting can provide great benefits and solutions for smart grid distribution and electricity management. However, its non-stationary behavior and randomness render its estimation very difficult. In this respect, a new hybrid learning approach is proposed for multi-hour global solar radiation forecasting, relying on Convolutional Neural Network (CNN), Nonparametric Gaussian Process Regression (GPR), Least Support Vector Machine (LS-SVM), and Extreme Learning Machine (ELM) as essence predictors. Then compressive sensing technique is applied to perform a hybridization scheme of the model’s output. Hourly global solar radiation data from two sites in Algeria with different climate conditions are used to evaluate the full potential of the integrated model, with stationarity checks with an advanced clear sky model (MecClear model). Different comparative simulations show the superiority of the proposed pipeline in forecasting hourly global solar radiation data for multi-hour ahead compared to the stand-alone model. Experimental results show that the proposed hybridization methodology can effectively improve the prediction accuracy and outperforms benchmarking models during all the forecasting horizons.
更多查看译文
关键词
Clear sky model, Forecasting, Machine learning, Deep learning model, Solar radiation energy, Compressive sensing
AI 理解论文
溯源树
样例
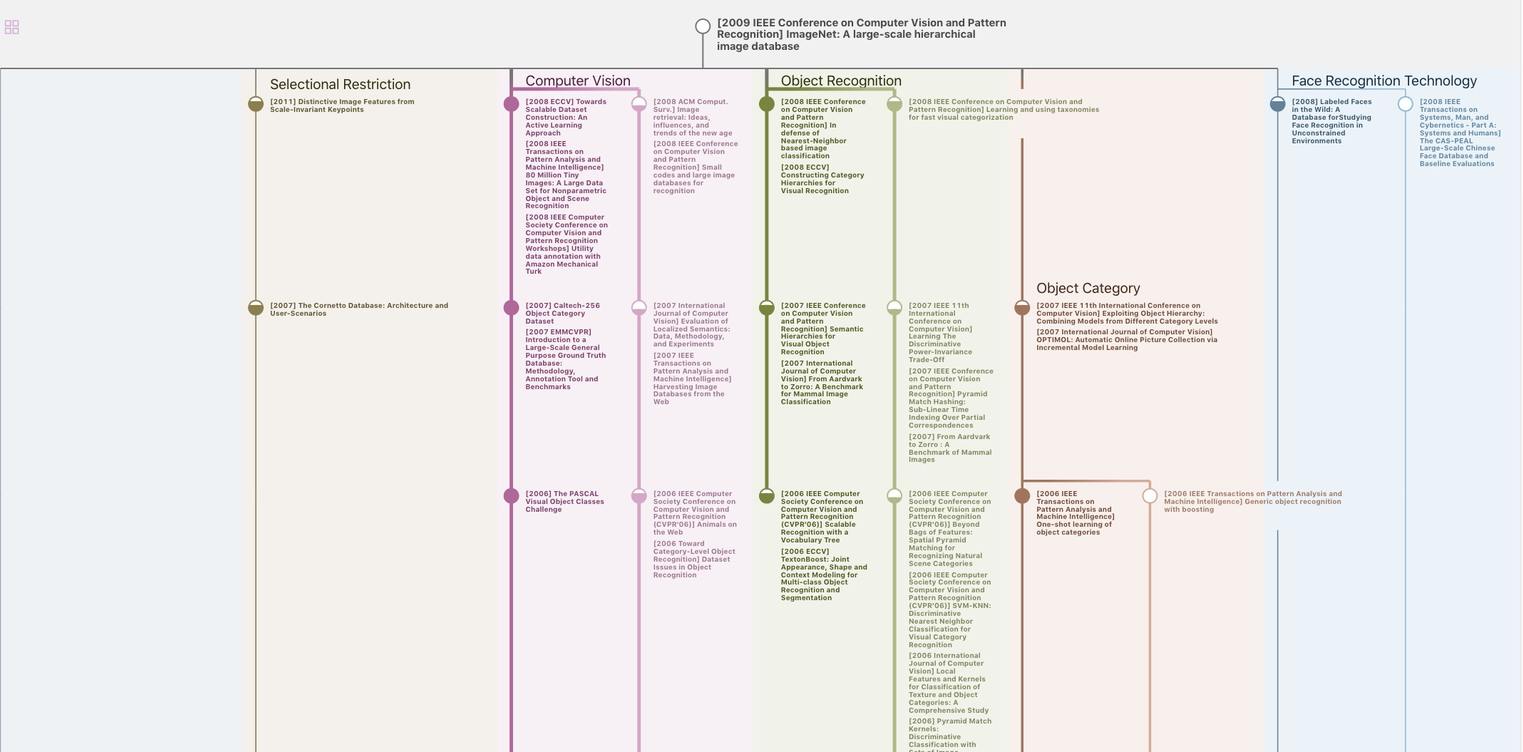
生成溯源树,研究论文发展脉络
Chat Paper
正在生成论文摘要