TransMKR: Translation-based knowledge graph enhanced multi-task point-of-interest recommendation
Neurocomputing(2022)
摘要
Point-of-interest (POI) recommendation aims to predict favorite POIs that have never visited before for users given their historical check-ins records. The introduction of knowledge graph can solve the heterogeneity of auxiliary information. However, the existing knowledge graph methods about POI recommendation only make use of the relationship between users and POIs or the context information (such as time series) for recommendation, but they neglect the deep relation consider the attribute connection of POIs themselves. In this paper, we propose a translation-based knowledge graph enhanced multi-task learning framework (TransMKR) for the POI recommendation. We improve the KGE module of MKR with TransR to quantify the relation between POIs and their attributes. As the POIs vector and the entity vector are actually two descriptions of the same item, the cross-sharing of information between them makes them obtain additional information from each other, and this enhances the expressive ability of POI data, thus it can alleviate the problem of data sparsity. An exquisitely designed structure is devised to capture the deep associated attributes of POIs under different relations. Empirically, this approach achieves the state-of-the-art model for POI recommendation based on geographical location on two public real-world datasets.
更多查看译文
关键词
Point-of-interest recommendation,TransMKR,Knowledge graph,Knowledge representation
AI 理解论文
溯源树
样例
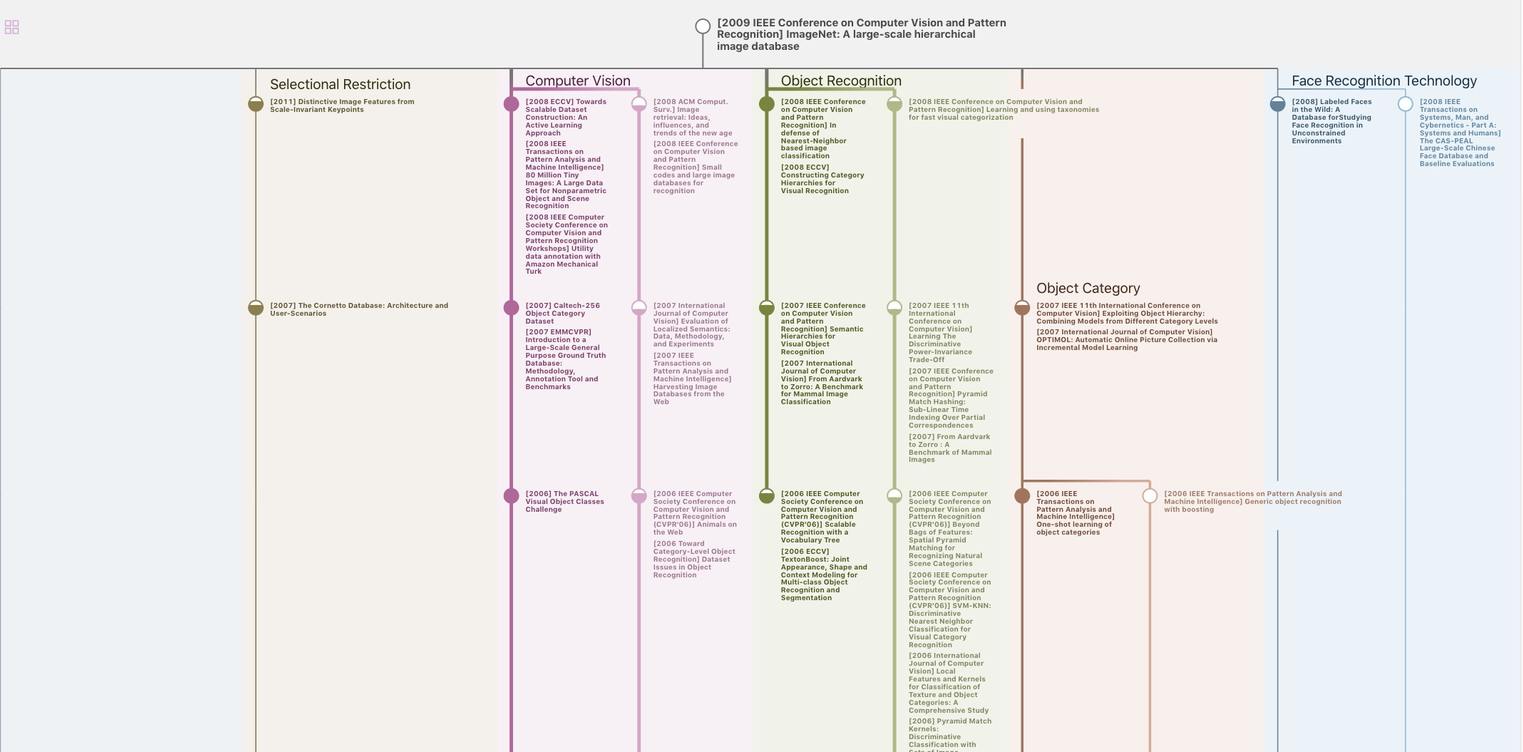
生成溯源树,研究论文发展脉络
Chat Paper
正在生成论文摘要