Dual Prototype Relaxation for Generalized Zero Shot Learning.
ICME(2021)
摘要
Generalized Zero Shot Learning (GZSL) is proposed to solve the training data missing problem by transferring the knowledge learned in seen classes to unseen classes. Many methods project the visual features into semantic space and find their nearest neighbours among the pre-defined attributes, which has achieved significant success. However, there are two problems involved in this type of methods, one is that the projection is a many-to-one mapping, which cannot maintain the diversity of features in semantic space, the other is that searching within all classes cannot well utilize the knowledge learned in the seen classes. In this paper, we propose a novel method named Dual Prototype Relaxation (DPR) by relaxing the projection from many-to-one to many-to-many. Specifically, we add noise to the semantic prototype in response to the projection of multiple features within a class, and reconstruct the visual features with the same relaxed prototype. Besides, in order to make better use of the knowledge learned in the seen classes, an Out-of-Domain (OoD) based method is employed to first classify the feature to seen or unseen domains, and then the same DPR model is applied to recognize its category within each domain. Extensive experiments on four popular datasets are conducted and the results show that our method can outperform many linear and deep state-of-the-art methods although our method is a linear one.
更多查看译文
关键词
Generalized Zero-Shot Learning,Out-of-Domain Detection,Dual Prototype Relaxation
AI 理解论文
溯源树
样例
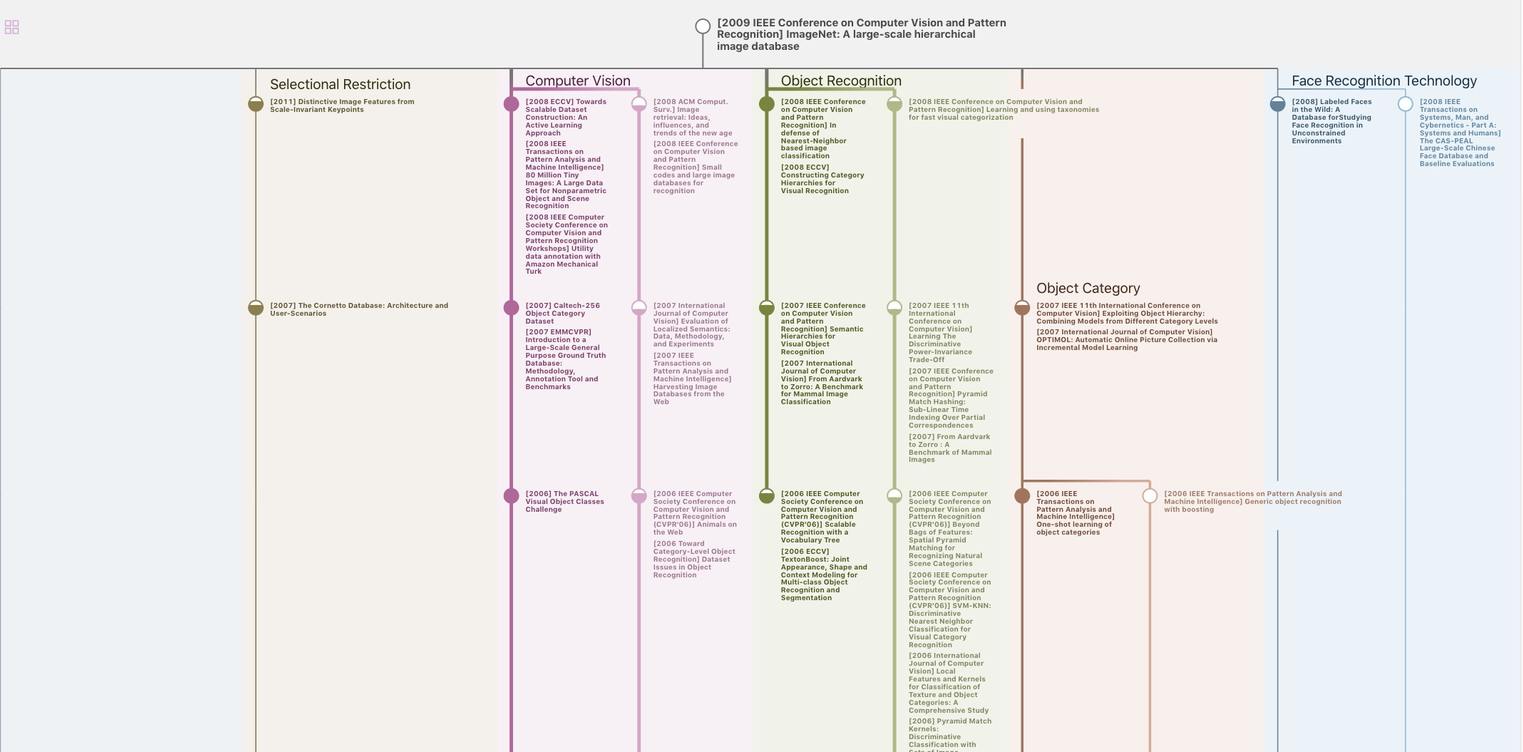
生成溯源树,研究论文发展脉络
Chat Paper
正在生成论文摘要