Enhancing Adversarial Examples Via Self-Augmentation.
ICME(2021)
摘要
Recently, adversarial attacks pose a challenge for the security of Deep Neural Networks, which motivates researchers to establish various defense methods. However, do current defenses really achieve real security? To answer the question, we propose self-augmentation method (SA) for circumventing defenders to transferable adversarial examples. Concretely, self-augmentation includes two strategies: (1) self-ensemble, which applies additional convolution layers to an existing model to build diverse virtual models that be fused for achieving an ensemble-model effect and preventing overfitting; and (2) deviation-augmentation, which based on the observation of defense models that the input data is surrounded by highly curved loss surfaces, thus inspiring us to apply deviation vectors to input data for escaping from their vicinity space. Extensive experiments conducted on four vanilla models and ten defenses suggest the superiority of our method compared with the state-of-the-art transferable attacks. The source code is public available at https://github.com/zhuangwz/ICME2021_self_augmentation.
更多查看译文
关键词
transferability,black-box attack,adversarial example,robustness,defense
AI 理解论文
溯源树
样例
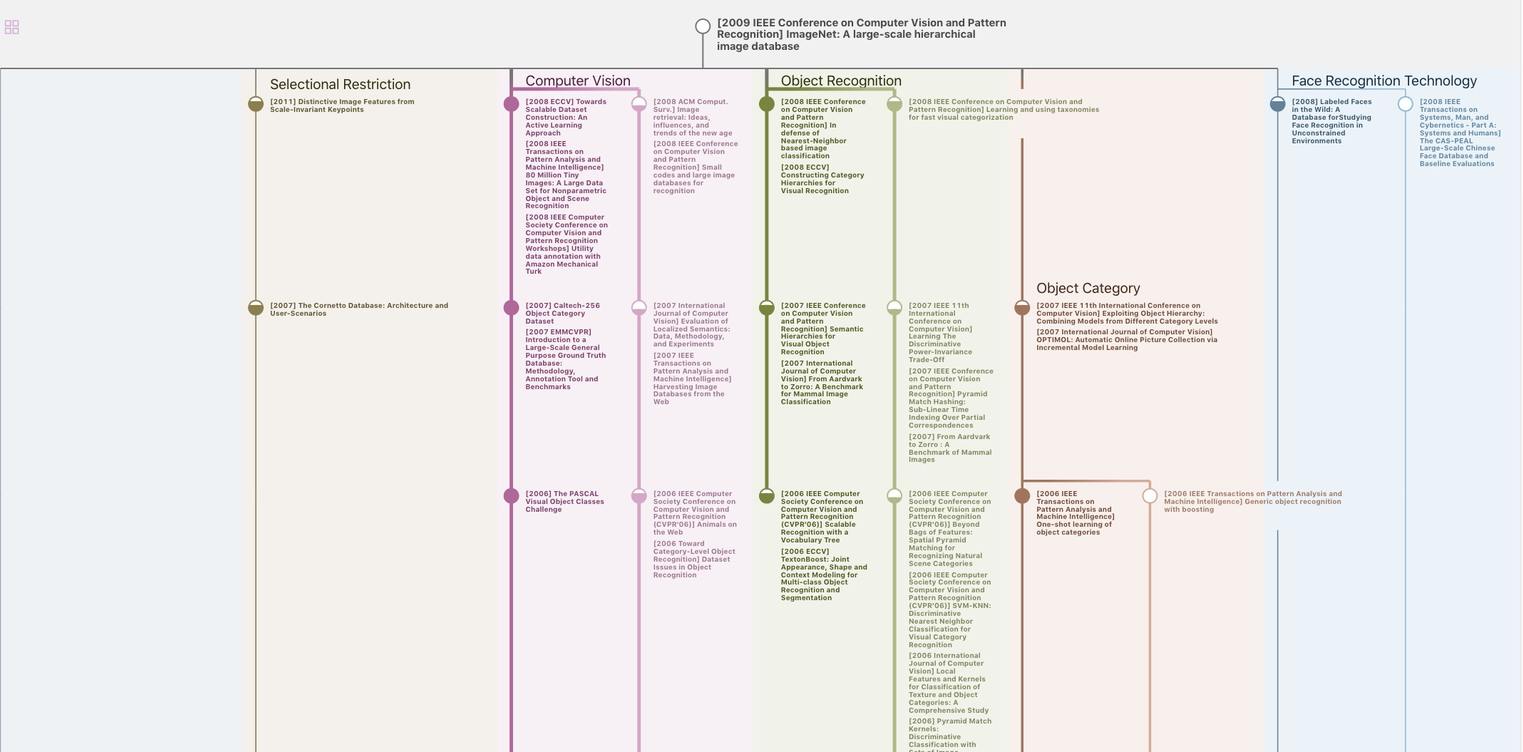
生成溯源树,研究论文发展脉络
Chat Paper
正在生成论文摘要