Temporally Coarse to Fine Snippets Relationship Learning with Graph Convolution for Temporal Action Proposal Generation.
ICME(2021)
摘要
Previous works have shown that explicit snippets relationship modeling can be helpful for feature learning on untrimmed action videos. However, the snippets relationship learning in these methods are far from optimal in that they failed to consider the valuable temporally coarse-grained features, learnable soft relationship weights, and separate relationship learning in different temporal orders. To address this issue, we proposed a novel SGC-Block for improved snippet relationship learning, which enables the temporally coarse-to-fine soft valued snippet-wise relationship learning in different temporal directions. The SGC-Block constructs the snippets graph and explicitly models the (1) temporal relations (TPR); (2) coarsegrained snippet-wise relations (CSR); (3) fine-grained snippet-wise relations (FSR); and an additional (4) adaptive relations (ADR). Especially, the novel CSR is inspired by the feature pyramid pooling structure to obtain the coarse feature presentations in the temporal dimension. Experimental results showed that our proposed approach outperforms most state-of-the-art methods on the THUMOS14 and ActivityNet-1.3 benchmarks.
更多查看译文
关键词
Snippets Relationship Learning,Graph Convolution,Action Proposal Generation,Video Analysis
AI 理解论文
溯源树
样例
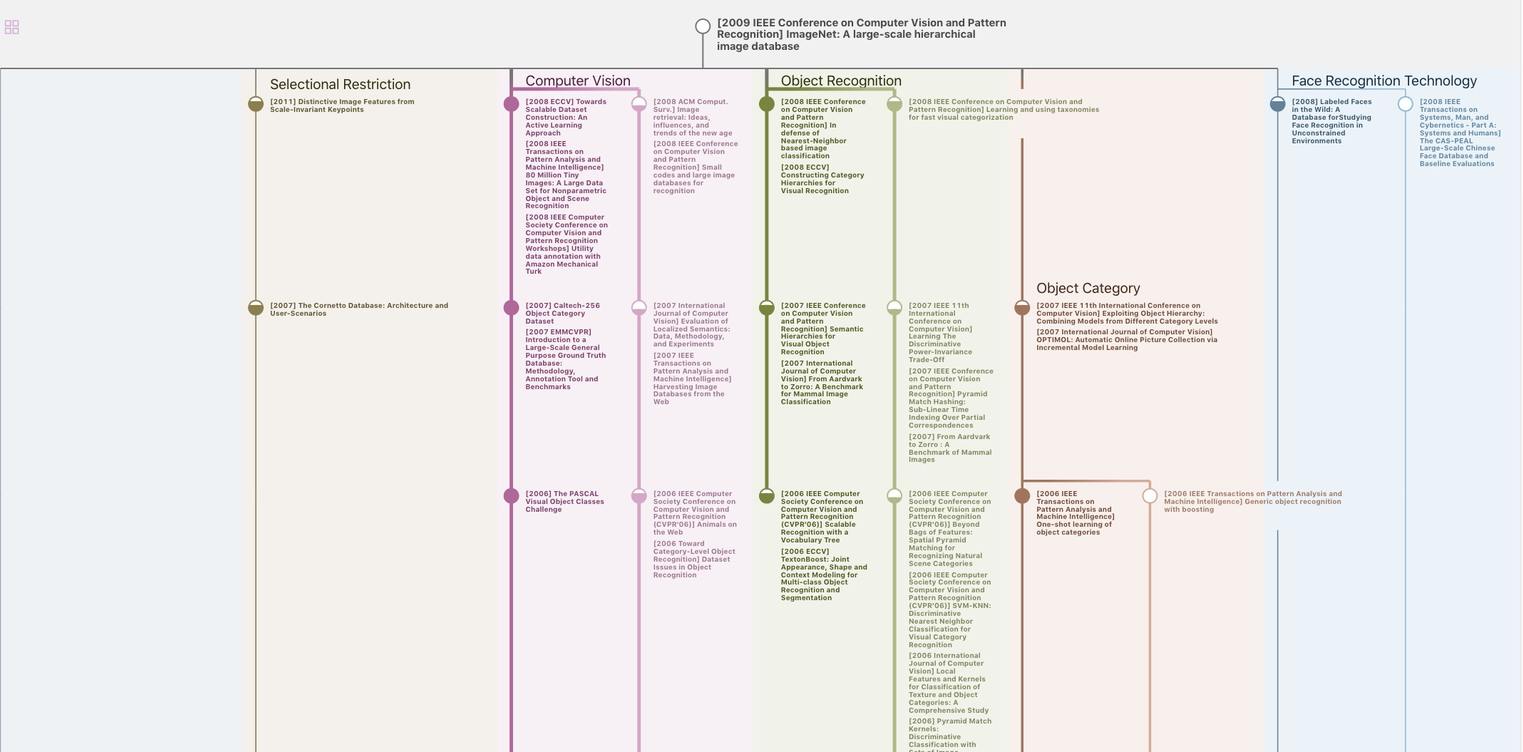
生成溯源树,研究论文发展脉络
Chat Paper
正在生成论文摘要