Improved Lightcnn with Attention Modules for Asv Spoofing Detection
ICME(2021)
摘要
With the advent of many state-of-the-art speech synthesis or conversion techniques, speaker recognition system is confronted with the imperceptible interference caused by spoofed speech. In this paper, we realize that filter bank distributions of cepstral features in the frequency domain cause considerable influence to the system performance through experiments. Therefore, given attention mechanism may help to focus on key information, this paper presents improved light convolutional neural network (LCNN) with attention modules, separately named Squeeze-and-Excitation (SE) block, Convolutional Block Attention Module (CBAM) and Dual Attention Network (DANet). To our knowledge, we are the first to do systematic study of attention mechanisms in the field of speech anti-spoofing. Experimented on ASVspoof 2019 dataset, our proposed single system can get 22% min-tDCF reduction as well as 42% EER reduction over LCNN baseline and its performance can even rank fourth among all participating teams using fusion systems in ASVspoof 2019 competition.
更多查看译文
关键词
anti-spoofing,attention module,light convolutional neural network,speech spoofing detection,speaker verification
AI 理解论文
溯源树
样例
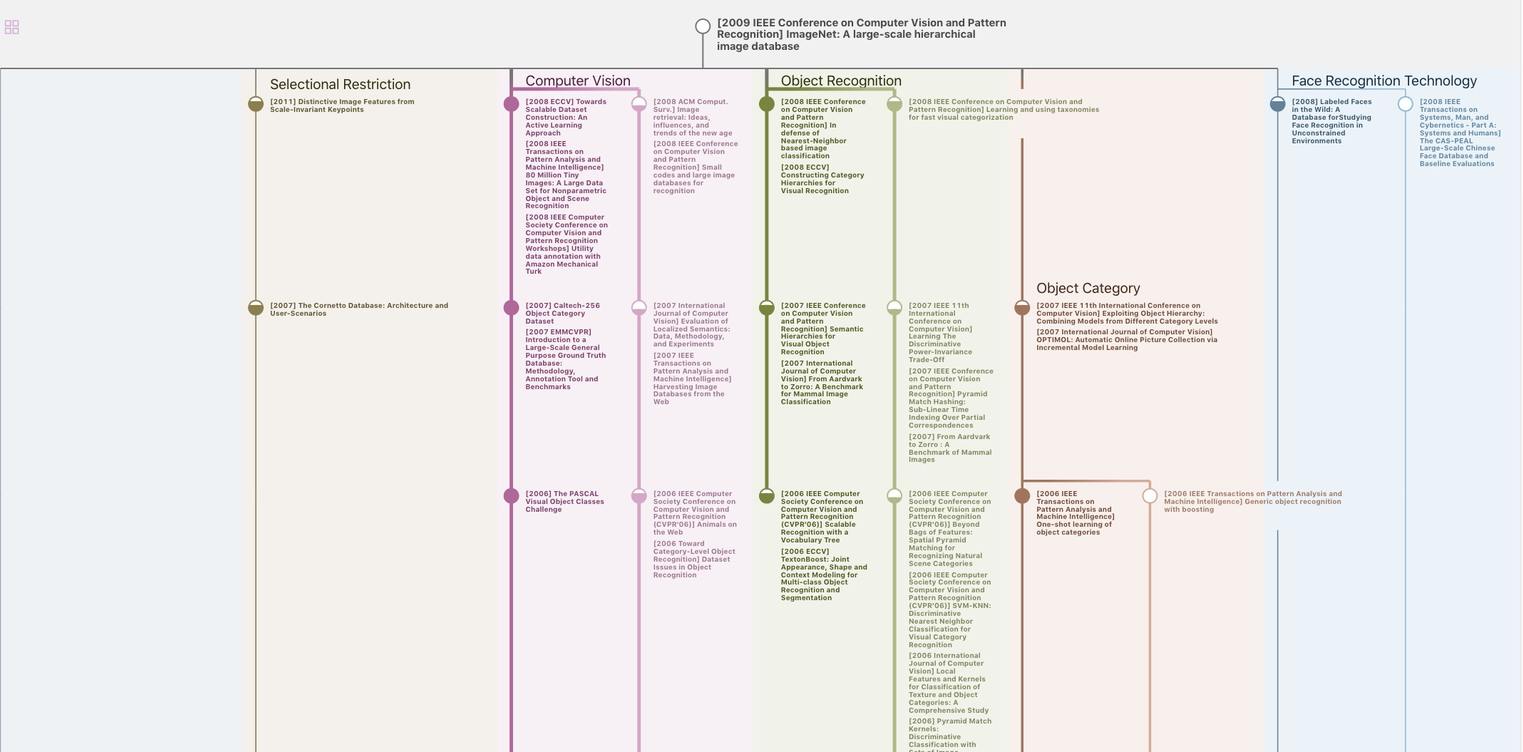
生成溯源树,研究论文发展脉络
Chat Paper
正在生成论文摘要