Feature Selection using Modified Null Importance
SSCI(2021)
摘要
Some difficult feature selection tasks have a property of 'small
$n$
large
$p$
. ‘Feature selection methods using variable importance measures that were defined in tree-based ensemble methods have reportedly shown a relatively good performance even in 'small
$n$
large
$p$
‘tasks. Among them, this study focuses on the feature selection method using the null importance. Through our preliminary experiments, we found that this feature selection method often fails to detect input variables that weakly affect the output. We should note that some practical feature selection tasks require to find all of the input variables that actually affect the output. In order to find even input variables that weakly affect the output, we thus modify the feature selection method using the null importance. Specifically, our method, what we call the feature selection method using the modified null importance, normalizes an importance score of each of the input variables by using those of random variables, and then uses the normalized score in order to determine whether or not the variable is relevant to the output. Through numerical experiments, we finally show that the modified method is capable of detecting even input variables that weakly affect the output.
更多查看译文
关键词
random forest,null importance,feature selection
AI 理解论文
溯源树
样例
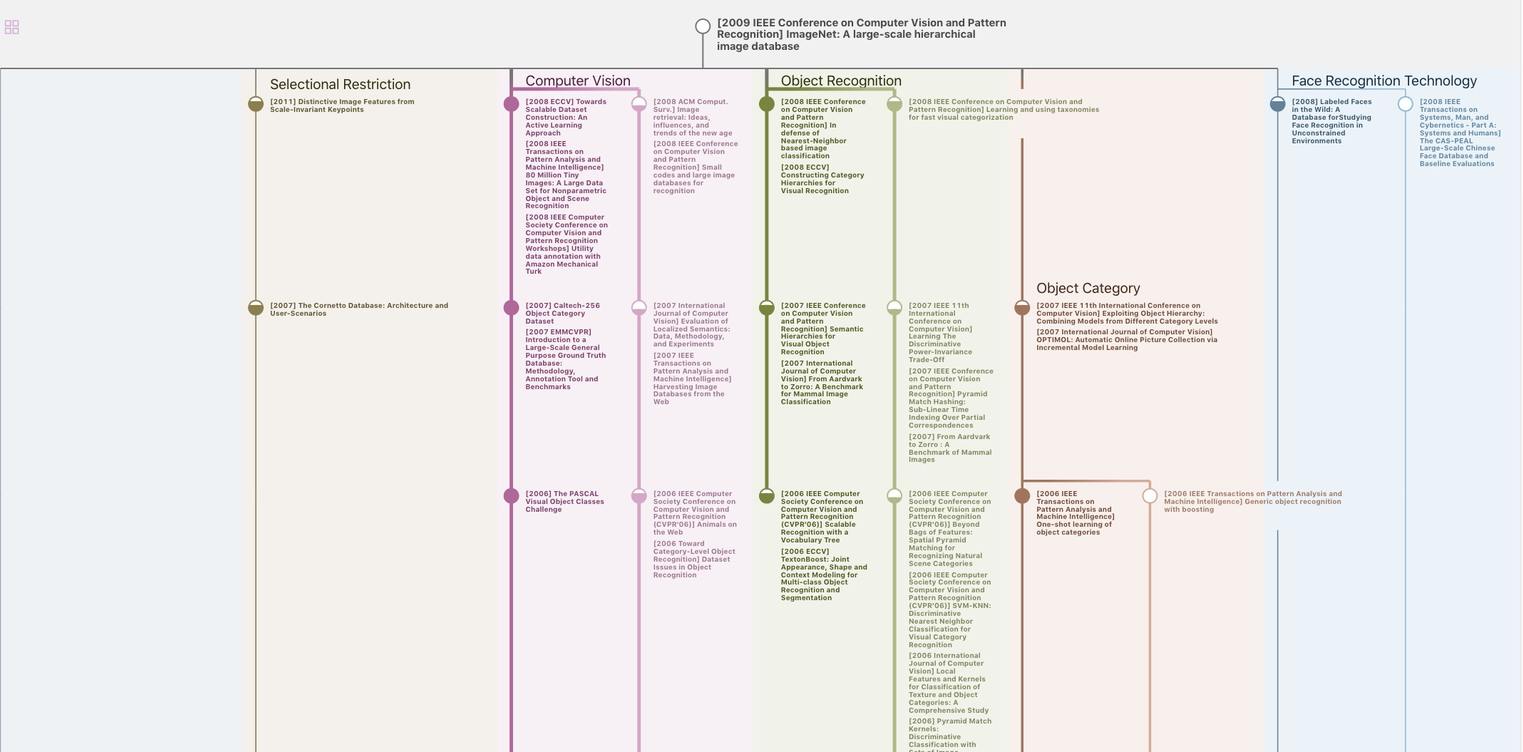
生成溯源树,研究论文发展脉络
Chat Paper
正在生成论文摘要