Predatory Conversation Detection Using Transfer Learning Approach
MACHINE LEARNING, OPTIMIZATION, AND DATA SCIENCE (LOD 2021), PT I(2022)
摘要
Predatory conversation detection on social media can proactively prevent the netizens, including youngsters and children, from getting exploited by sexual predators. Earlier studies have majorly employed machine learning approaches such as Support Vector Machine (SVM) for detecting such conversations. Since deep learning frameworks have shown significant improvements in various text classification tasks, therefore, in this paper, we propose a deep learning-based classifier for detecting predatory conversations. Furthermore, instead of designing the system from the beginning, transfer learning has been proposed where the potential of the pre-trained BERT (Bidirectional Encoder Representations from Transformers) model is utilized to solve the predator detection problem. BERT is mostly used to encode the textual information of a document into its context-aware mathematical representation. The inclusion of this pre-trained model solves two major problems, i.e. feature extraction and Out of Vocabulary (OOV) terms. The proposed system comprises two components: a pre-trained BERT model and a feed-forward neural network. To design the classification system with a pretrained BERT model, two approaches (feature-based and fine-tuning) have been used. Based on these approaches two solutions are proposed, namely, BERT_frozen and BERT_tuned where the latter approach is seen performing better than the existing classifiers in terms of F-1 and F-0.5- scores.
更多查看译文
关键词
Child grooming, Online sexual predators, Deep learning, Language modelling, BERT
AI 理解论文
溯源树
样例
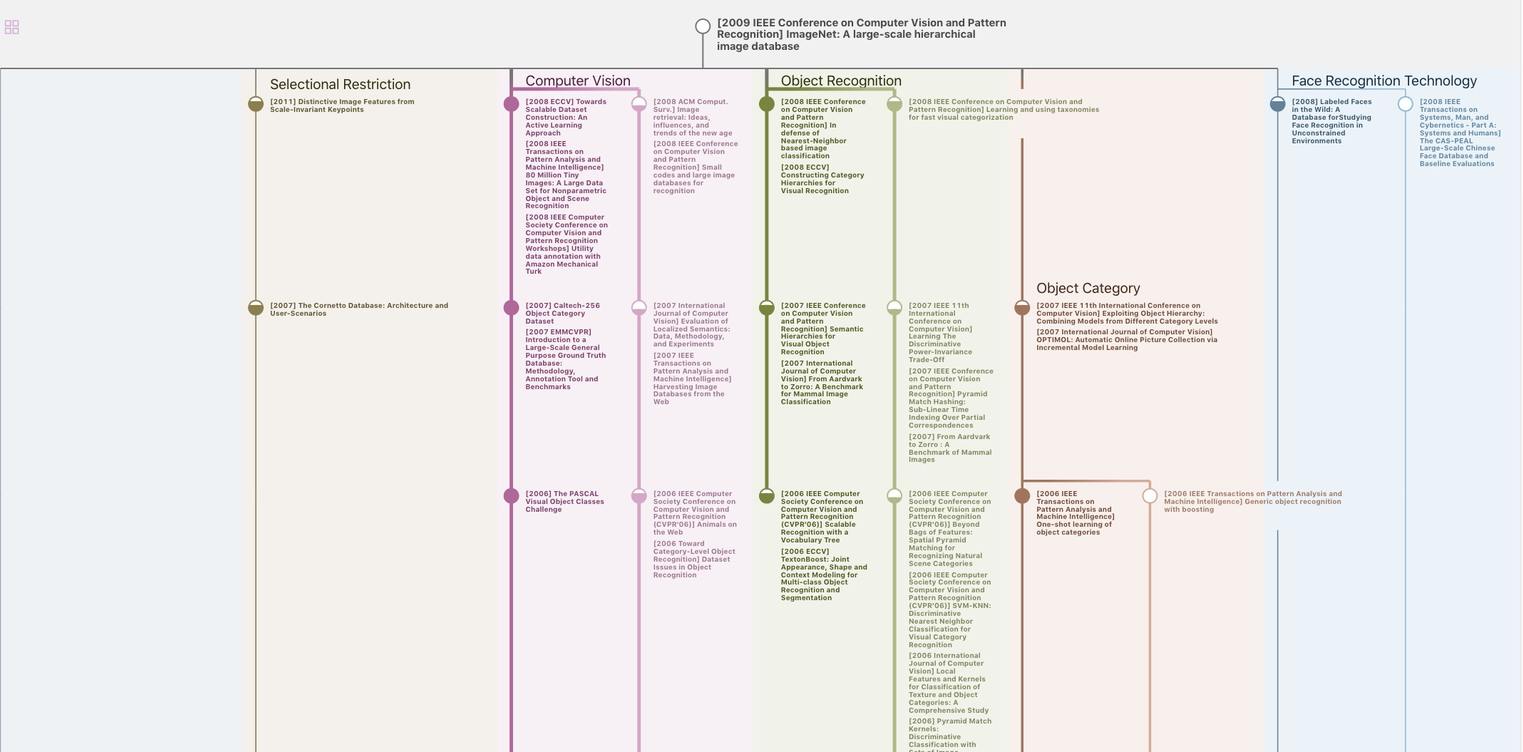
生成溯源树,研究论文发展脉络
Chat Paper
正在生成论文摘要