The Label Recorder Method Testing the Memorization Capacity of Machine Learning Models
MACHINE LEARNING, OPTIMIZATION, AND DATA SCIENCE (LOD 2021), PT I(2022)
摘要
Highly-parameterized deep neural networks are known to have strong data-memorization capability, but does this ability to memorize random data also extend to simple standard learning methods with few parameters? Following recent work exploring memorization in deep learning, we investigate memorization in standard non-neural learning models through the label recorder method, which uses a model's training accuracy on randomized data to estimate its memorization ability, giving a distribution- and regularization-dependent label recording score. Label recording scores can be used to measure how capacity changes in response to regularization and other hyperparameter choices. This method is fully empirical, easy to implement, and works for all black-box classification methods. The label recording score supplements existing theoretical measures of model capacity such as Rademacher complexity and Vapnik-Chervonenkis (VC) dimension, while agreeing with conventional intuitions regarding statistical learning processes. We find that memorization ability is not limited to only over-parameterized models, but instead exists as a continuum, being present (to some degree) even in simple learning models with few parameters.
更多查看译文
关键词
Representational capacity, Label recorder, Label recording score
AI 理解论文
溯源树
样例
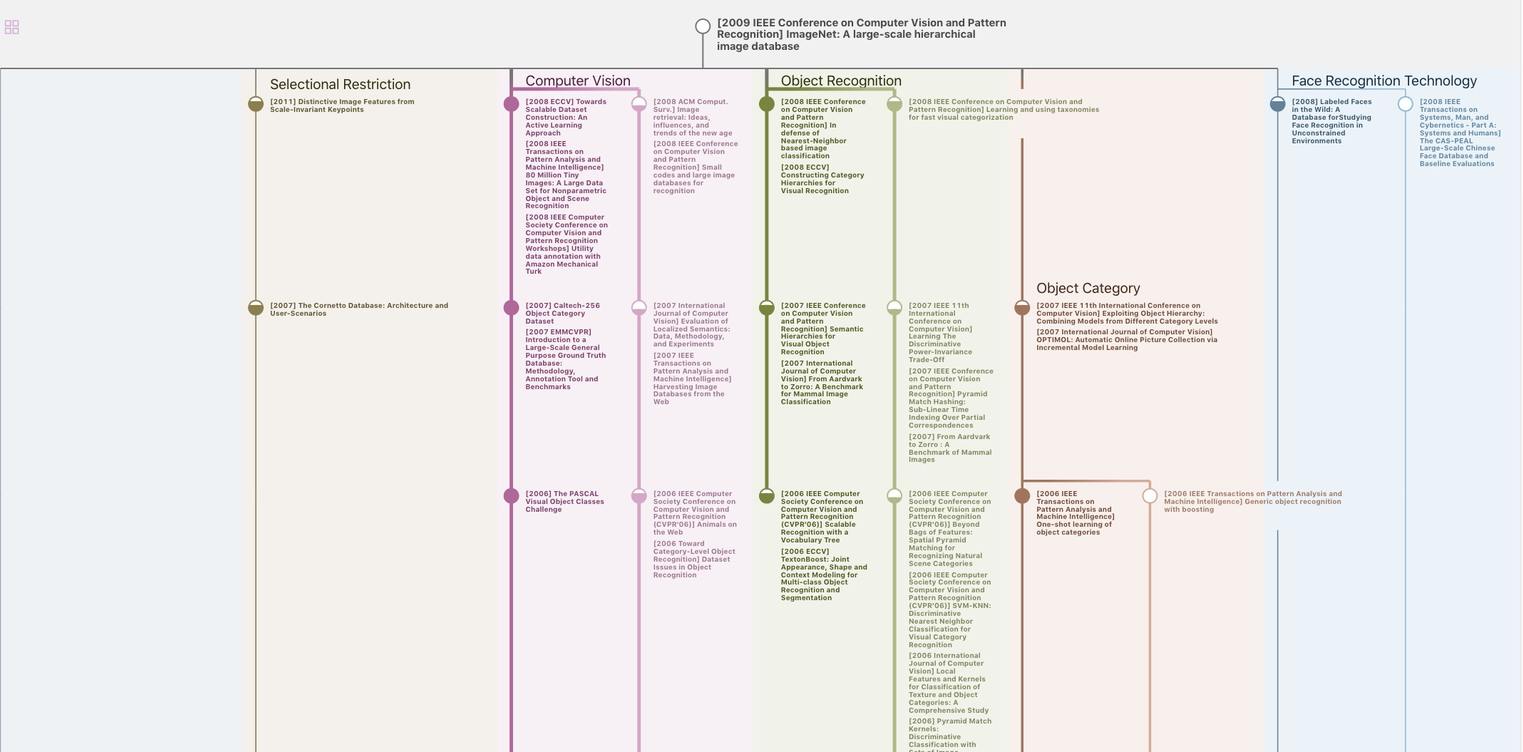
生成溯源树,研究论文发展脉络
Chat Paper
正在生成论文摘要