Annotation of Struck-out Text in Handwritten Documents.
ADCS(2021)
摘要
Annotating handwritten documents for training deep learning models is a major issue in handwritten text recognition. It requires manual effort to annotate each word in a document to specify the ground truth. Often documents contain struck-out text which needs to be ignored by the recognition process. In preparing training data, struck-out text needs to be represented in a way that can help deep learning models to learn to deal appropriately with the strike-outs. The question is how to do this. In this paper, we have investigated two approaches for struck-out text annotation: (1) provide no annotation, thus reducing the annotation burden, and (2) mark the struck-out text with a special symbol, we have used the symbol #. We have trained two models on a synthetically generated dataset using a convolutional neural network and LSTM. We obtained 8.8% and 9.0% character error rates for models one and two respectively. There was no statistically significant difference in the performance of the two models. This indicates that a model trained with minimal annotations can perform as well as a model trained with extra annotations for struck-out text.
更多查看译文
关键词
annotation,text,documents,struck-out
AI 理解论文
溯源树
样例
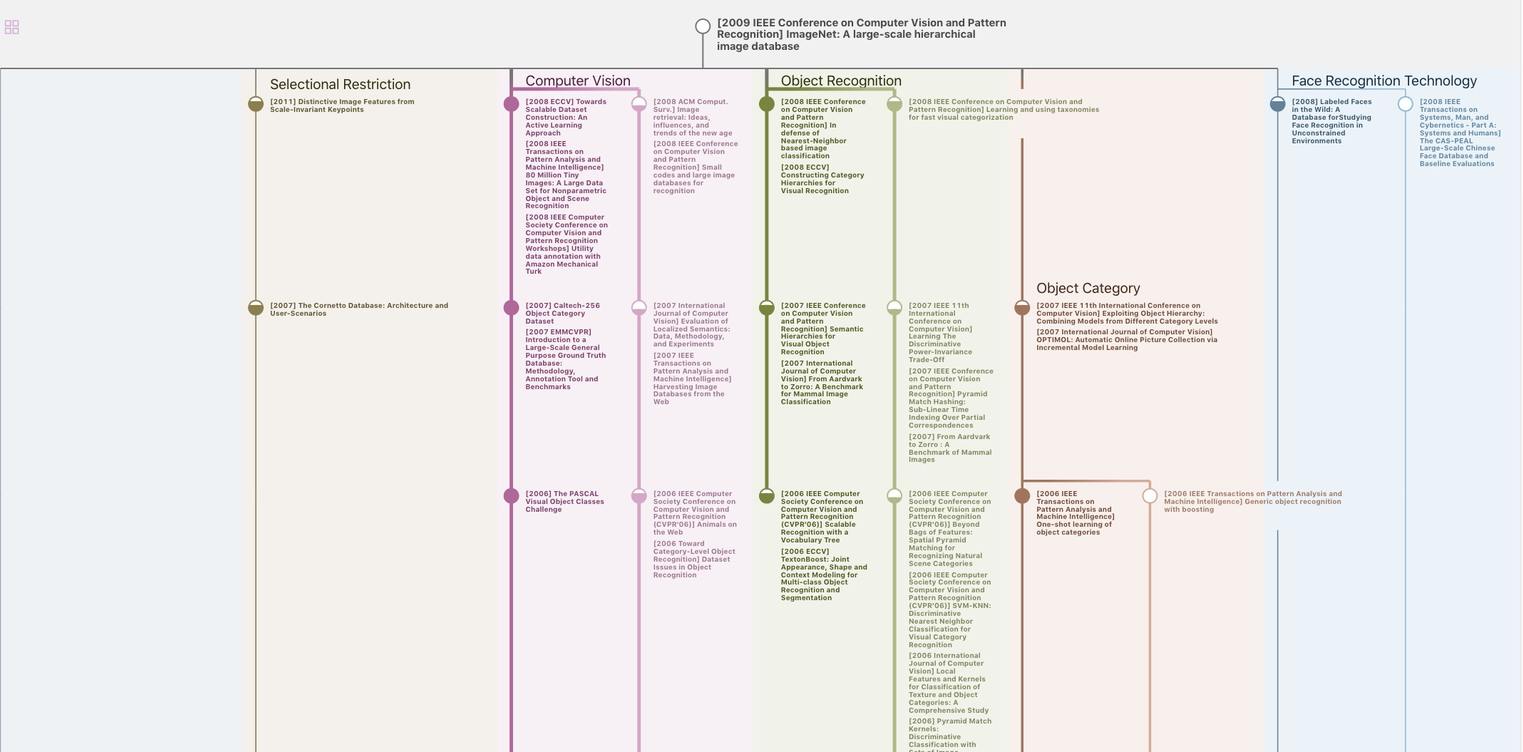
生成溯源树,研究论文发展脉络
Chat Paper
正在生成论文摘要