Unsupervised Fast Visual Localization and Mapping with Slow Features.
ICIP(2021)
摘要
Visual localization is the task of accurately estimating the camera's position in a known environment. State-of-the-art methods use the 3D structure of a scene for precise visual localization. However, 3D scene reconstruction is resource-intensive in terms of hardware requirements and computation time, making it infeasible to run on low-cost embedded hardware. Unsupervised spatial representation learning with Slow Feature Analysis (SFA) enables computationally inexpensive localization and mapping. This paper analyzes SFA-based and the well-known structure-based localization, i.e., Active Search, in two distinct settings: short-term temporal and extreme spatial generalization. We present the experimental results from an outdoor environment and compare both methods w.r.t localization accuracy and computation time. Results show that the SFA-based approach is 886x faster in mapping time and 34x faster in localization than Active Search while achieving comparable localization accuracy in our test scenario.
更多查看译文
关键词
Localization,Mapping,Unsupervised learning,Computer vision,Service robots
AI 理解论文
溯源树
样例
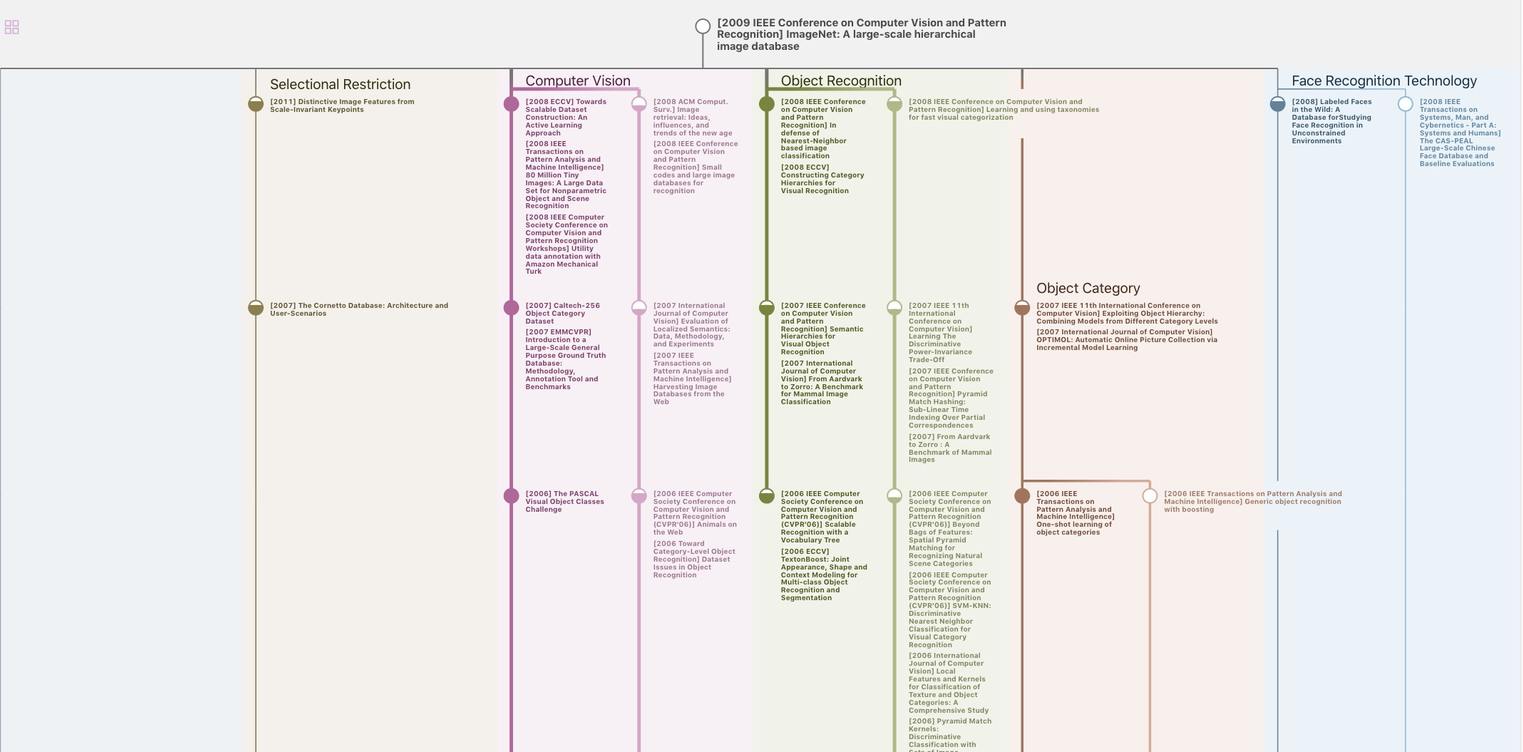
生成溯源树,研究论文发展脉络
Chat Paper
正在生成论文摘要