Knowledge Transferred Fine-Tuning for Anti-Aliased Convolutional Neural Network in Data-Limited Situation.
ICIP(2021)
摘要
Anti-aliased convolutional neural networks (CNNs) introduce blur filters to intermediate representations in CNNs to achieve high accuracy. A promising way to build a new antialiased CNN is to fine-tune a pre-trained CNN, which can easily be found online, with blur filters. However, blur filters drastically degrade the pre-trained representation, so the finetuning needs to rebuild the representation by using massive training data. Therefore, if the training data is limited, the fine-tuning cannot work well because it induces overfitting to the limited training data. To tackle this problem, this paper proposes "knowledge transferred fine-tuning." On the basis of the idea of knowledge transfer, our method transfers the knowledge from intermediate representations in the pre-trained CNN to the anti-aliased CNN while fine-tuning. We transfer only essential knowledge using a pixel-level loss that transfers detailed knowledge and a global-level loss that transfers coarse knowledge. Experimental results demonstrate that our method significantly outperforms the simple fine-tuning method.
更多查看译文
关键词
Convolutional neural network,anti-aliased CNN,knowledge transfer,data-limited situation
AI 理解论文
溯源树
样例
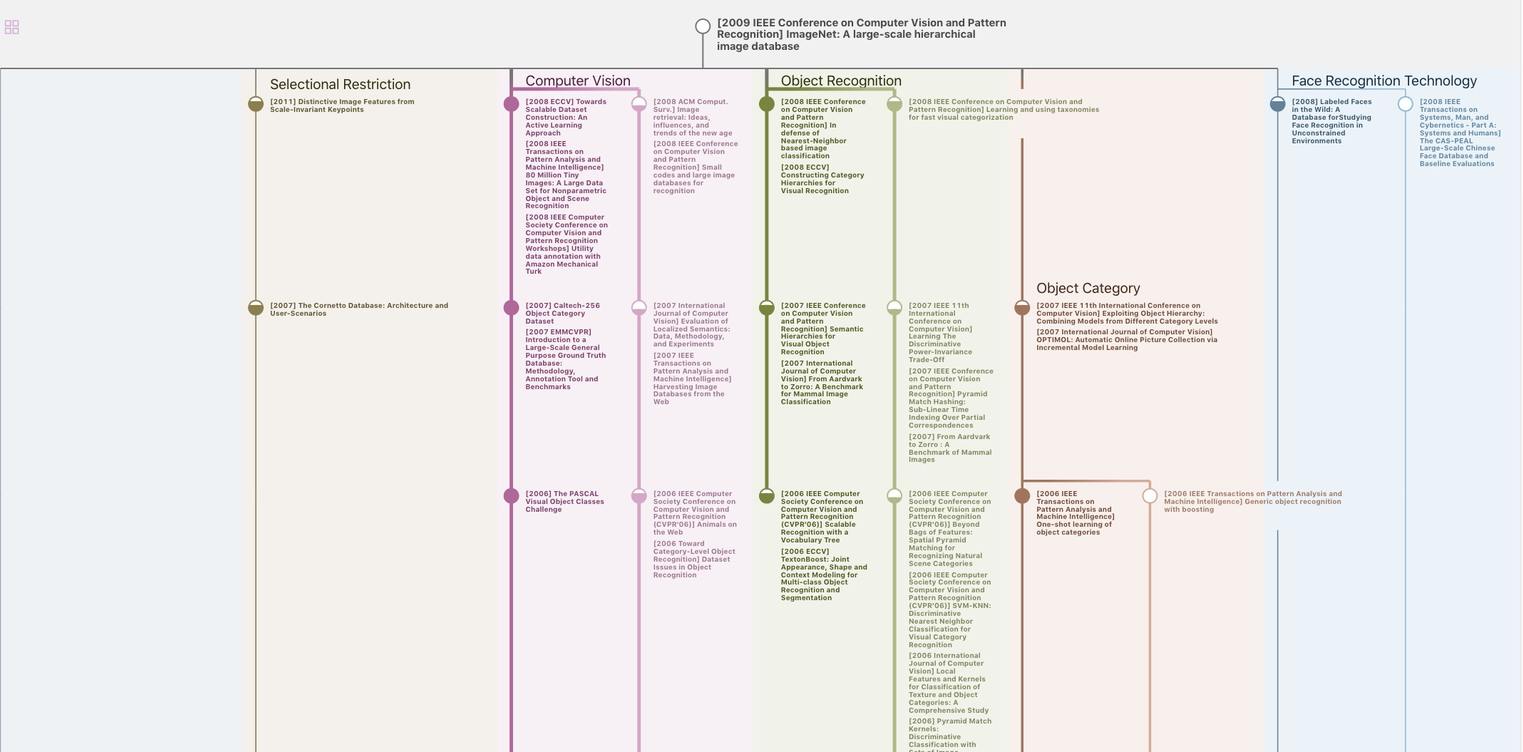
生成溯源树,研究论文发展脉络
Chat Paper
正在生成论文摘要