MTCNet: Multi-Task Complex Network for Concurrent Channel Estimation and Equalization
2021 IEEE Global Communications Conference (GLOBECOM)(2021)
摘要
Convolutional neural networks (CNNs) have been widely adopted in various fields and the wireless communication domain is no exception; researchers leveraged CNNs for communication tasks such as channel estimation and equalization. They showed the potential of CNNs for these applications but were insufficient to be considered for real world implementation due to the limited applicability and high execution latency. In this paper, we propose a novel complex number-based neural network architecture, a multi-task loss function, and a training scheme to learn channel estimation and equalization tasks simultaneously in an end-to-end manner. The resulting single-stage CNN architecture, Multi-Task Complex Network (MTCNet), achieves better performance than any other conventional methods. In addition, to the best of our knowledge, MTCNet is the first CNN-based channel estimation and equalization that can operate with various subcarrier settings with inferencing time below 1 ms.
更多查看译文
关键词
channel estimation,channel equalization,convolutional neural network,complex number-based operation,end-to-end learning,multi-task learning
AI 理解论文
溯源树
样例
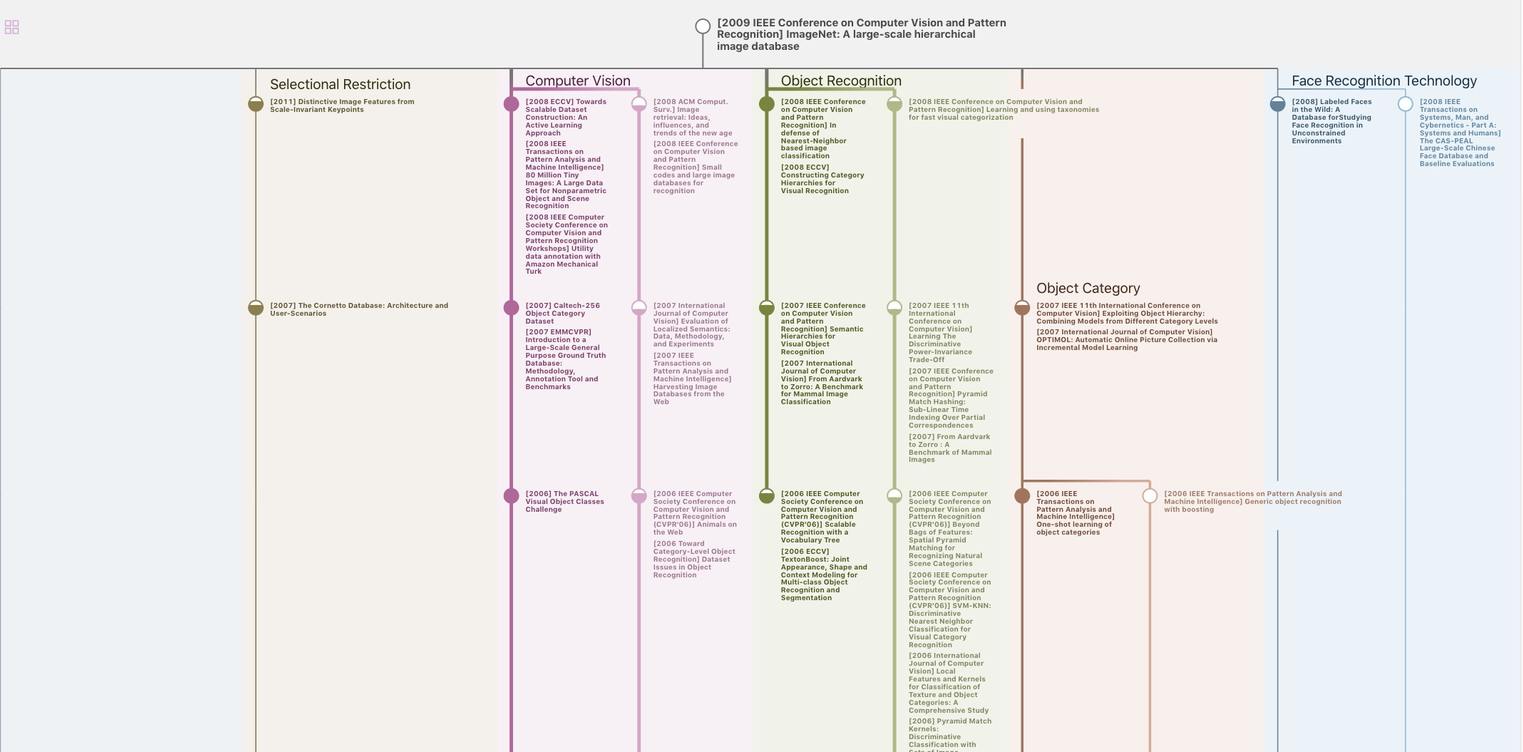
生成溯源树,研究论文发展脉络
Chat Paper
正在生成论文摘要