AFB: Improving Communication Load Forecasting Accuracy with Adaptive Feature Boosting
2021 IEEE GLOBAL COMMUNICATIONS CONFERENCE (GLOBECOM)(2021)
摘要
Prediction of key system characteristics, such as the communication load, is required to overcome the delays in wireless communication systems. State-of-The-Art (SOTA) approaches mostly apply existing Neural Network (NN) structures, and extract latent features purely based on their sensitivity to the forecasting accuracy. This way of feature extraction may neglect some non-obvious yet informative dimensions in the model input, leading to inaccurate forecasting results. In this paper, we present an Adaptive Feature Boosting (AFB) approach, which integrates multiple AutoEncoders (AEs) to automatically extract robust and comprehensive latent features for communication load forecasting. The recurrent and residual connections among the AEs make sure that the extracted latent features are representative for all input dimensions. With more comprehensive information extracted from the history, the forecasting accuracy is thus improved. We evaluate AFB against existing approaches on a real-world dataset that contains Call Detail Records (CDRs) of the Milan city over a period of two months. The evaluation shows that our AFB-based approach achieves 35.2% more accurate load forecasting results than the SOTA deep approaches.
更多查看译文
关键词
model input,inaccurate forecasting results,adaptive feature boosting approach,AEs,robust features,comprehensive latent features,recurrent connections,residual connections,extracted latent features,input dimensions,AFB-based approach,accurate load forecasting results,SOTA deep approaches,communication load forecasting accuracy,key system characteristics,wireless communication systems,neural network structures,feature extraction,informative dimensions
AI 理解论文
溯源树
样例
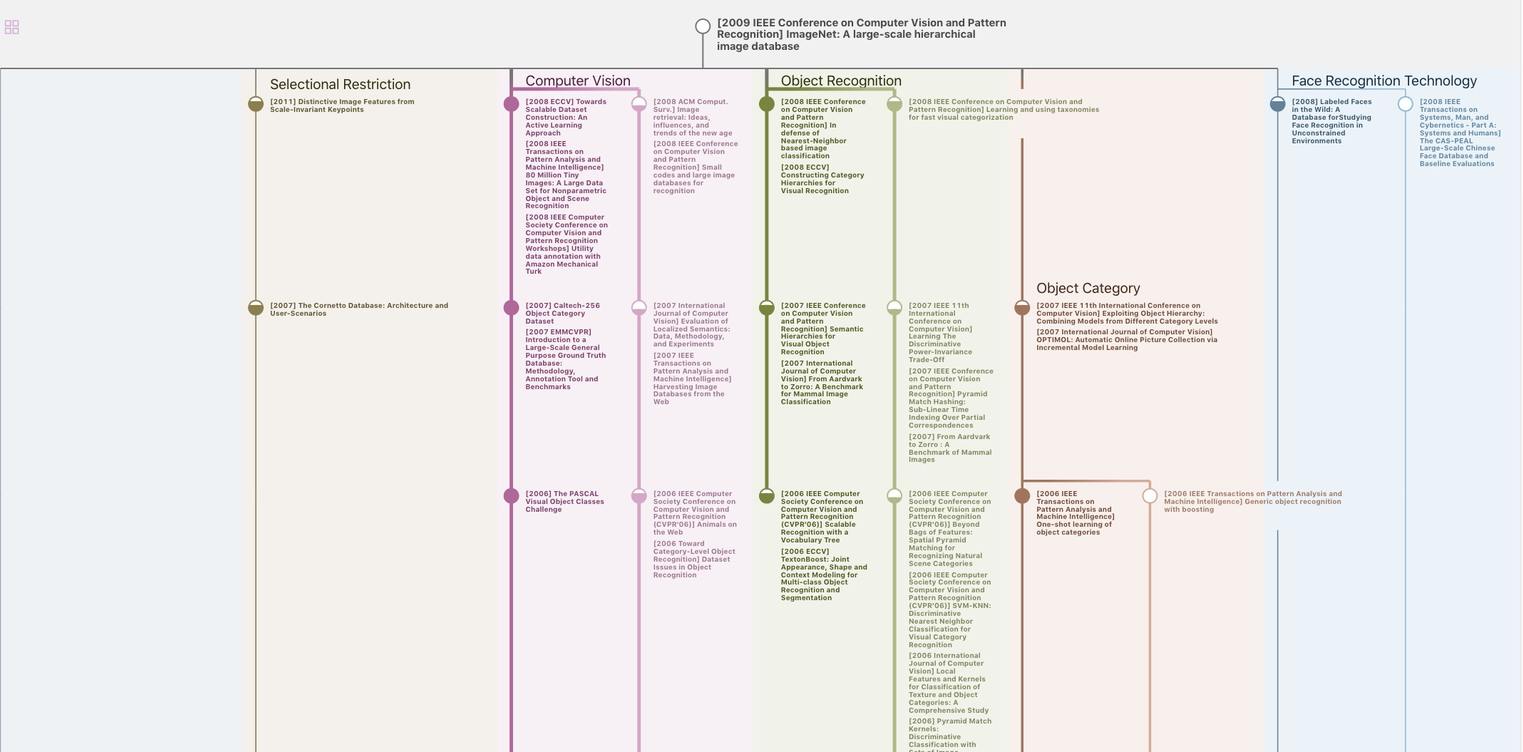
生成溯源树,研究论文发展脉络
Chat Paper
正在生成论文摘要