Driver Distraction Impact on Road Safety: A Data-driven Simulation Approach
2021 IEEE GLOBAL COMMUNICATIONS CONFERENCE (GLOBECOM)(2021)
摘要
Driver distraction identification is crucial to improve road safety. Through vehicular communications, vehicles can exchange driver behavior information, based on which distracted drivers can be identified, and all drivers can be notified, which can mitigate the impact of driver distraction on road safety. To build such systems, it is essential to understand the different types of distraction, and how they affect driver behavior and their relationship to crashes or near-crashes. This understating should be based on real datasets, which are very limited. Therefore, in this paper, we cover this gap by building a data-driven simulation model to quantify the impact of realistic driver distraction on traffic safety. In particular, we use the 2nd Strategic Highway Research Program Naturalistic Driving Study (SHRP2 NDS) dataset to develop a simulation framework for driver distraction. First, we pre-process and analyze the dataset for different types of distractions. The analysis shows that the data can not be fitted to any of the known distributions. Therefore, we use the Gaussian Mixture Model (GMM) to represent the distraction intervals for the different distraction types. We then use these GMM models and the statistics collected from the data to realistically simulate the driver distraction using the Simulation for Urban MObility (SUMO) software. Finally, we use this framework to simulate the driver distraction in a real network. The data analysis and simulation results revealed important and interesting conclusions, such as decreasing the crash ratio when roads become congested.
更多查看译文
关键词
Driver Distraction, Traffic Safety, Gaussian Mixture Model, Simulation
AI 理解论文
溯源树
样例
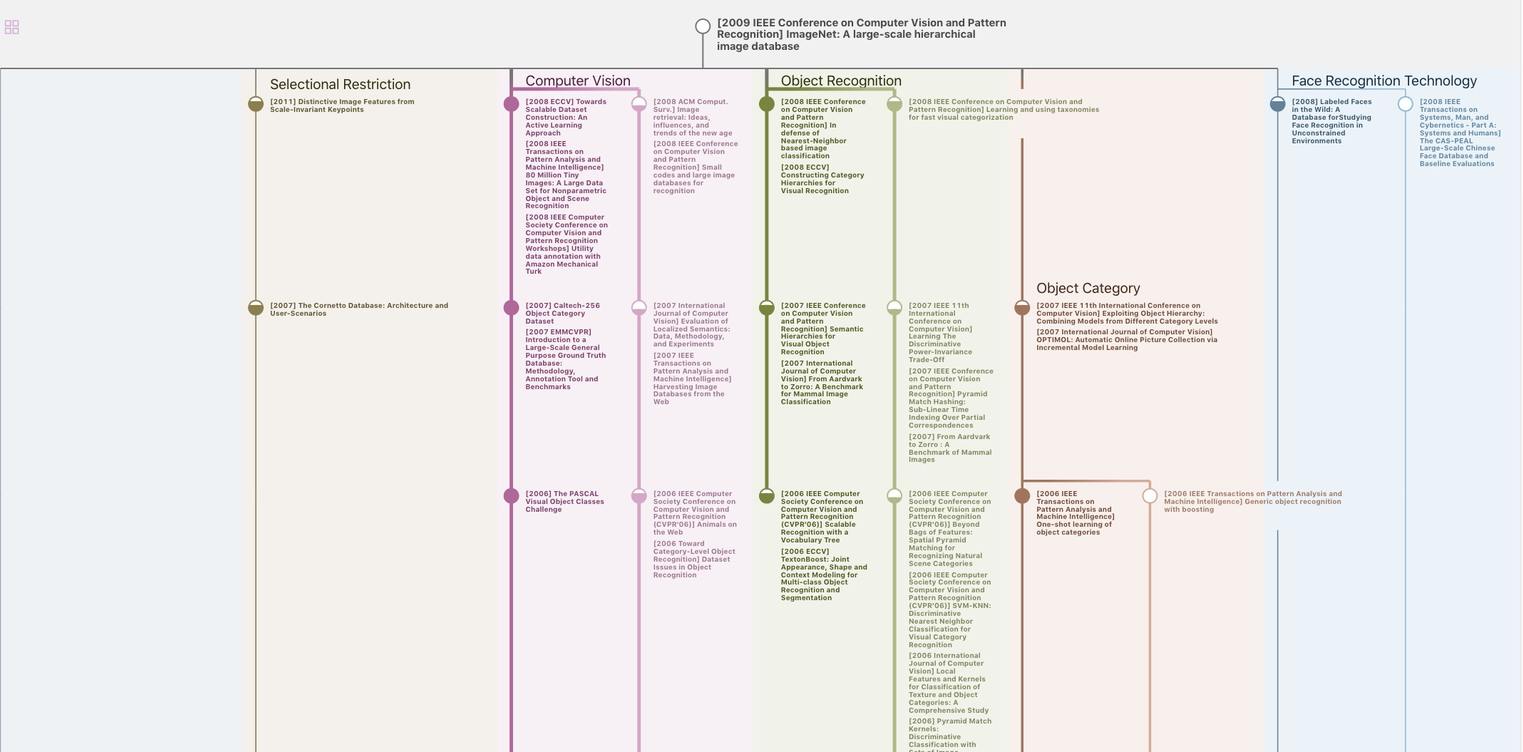
生成溯源树,研究论文发展脉络
Chat Paper
正在生成论文摘要