GDST: Global Distillation Self-Training for Semi-Supervised Federated Learning
2021 IEEE Global Communications Conference (GLOBECOM)(2021)
Abstract
Federated Learning (FL) refers to the machine learning scheme that enables decentralized model training over massive separate data sources without privacy concerns. However, existing works rarely consider difficulty of obtaining sufficient data labels due to uncontrollable user behavior, especially in cross-device FL scenarios. In this paper, we consider semi-supervised federated learning (SSFL) setups and mainly focus on the disjoint scenario where local clients only have access to unlabeled data. By integrating self-training scheme for unlabeled data, we propose self-training loss as part of local training objective within federated learning framework. To further stablize and improve the learning process, we propose global distillation loss that utilize output logits of global model for per client-sample as supervision and also soften such distillation by temperature to obtain more discriminative information. Based on self-training and global distillation loss, combined with server-side training, we propose Global Distillation Self-Training (GDST) Federated Learning algorithm, which enables to distributedly learn a global model in the disjoint scenario of SSFL. Finally, we do sufficient ablation study to explore the role of each component of our GDST method, experimentally guarantee the interpretability.
MoreTranslated text
Key words
federated learning,semi-supervised,self-training,global distillation
AI Read Science
Must-Reading Tree
Example
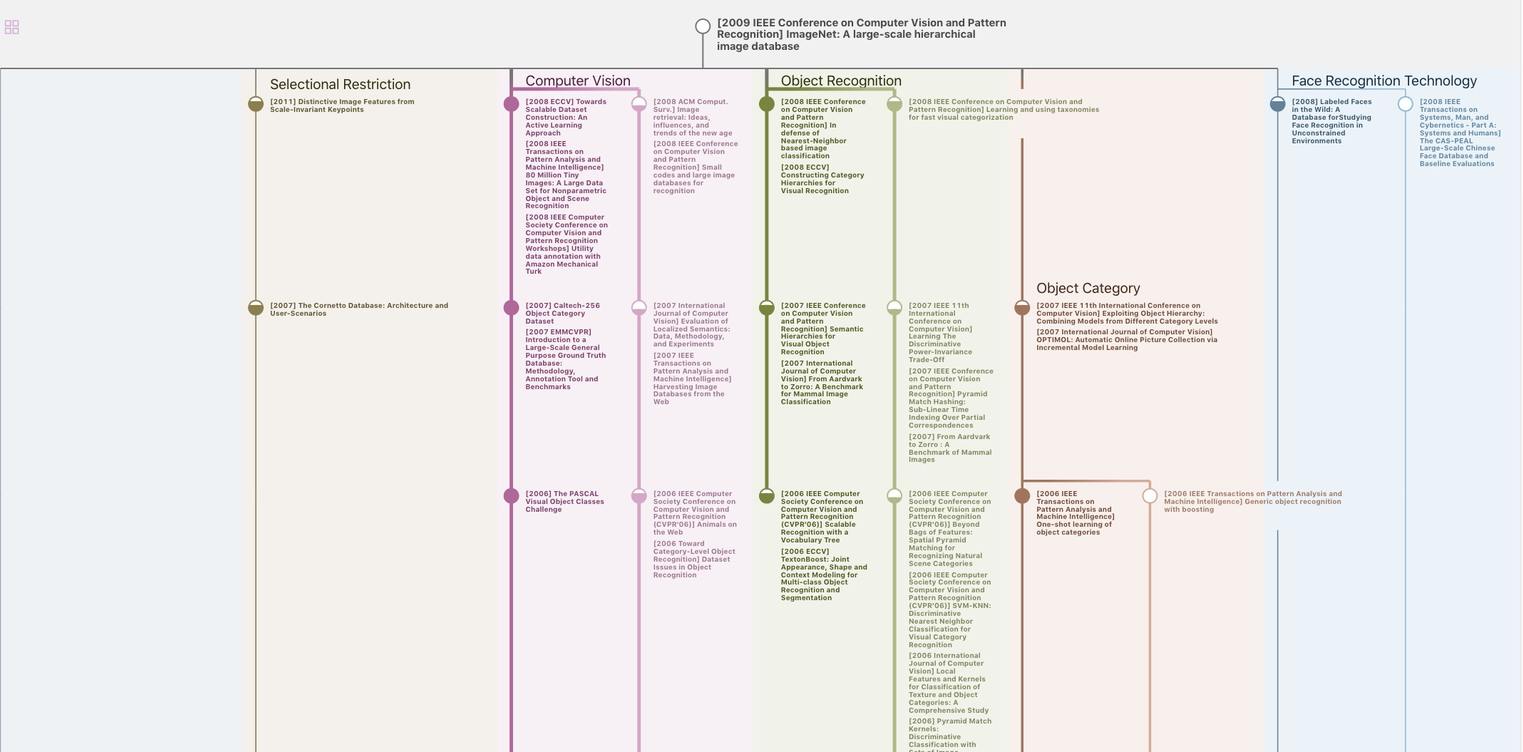
Generate MRT to find the research sequence of this paper
Chat Paper
Summary is being generated by the instructions you defined